Cyclic Self-attention for Point Cloud Recognition.
ACM Trans. Multim. Comput. Commun. Appl.(2023)
摘要
Point clouds provide a flexible geometric representation for computer vision research. However, the harsh demands for the number of input points and computer hardware are still significant challenges, which hinder their deployment in real applications. To address these challenges, we design a simple and effective module named cyclic self-attention module (CSAM). Specifically, three attention maps of the same input are obtained by cyclically pairing the feature maps, thus exploring the features sufficiently of the attention space of the original input. CSAM can adequately explore the correlation between points to obtain sufficient feature information despite the multiplicative decrease in inputs. Meanwhile, it can direct the computational power to the more essential features, relieving the burden on the computer hardware. We build a point cloud classification network by simply stacking CSAM called cyclic self-attention network (CSAN). We also propose a novel framework for point cloud semantic segmentation called full cyclic self-attention network (FCSAN). By adaptively fusing the original mapping features and the CSAM extracted features, it can better capture the context information of point clouds. Extensive experiments on several benchmark datasets show that our methods can achieve competitive performance in classification and segmentation tasks.
更多查看译文
关键词
Point cloud,self-attention,cyclic pairing,adaptive fuse
AI 理解论文
溯源树
样例
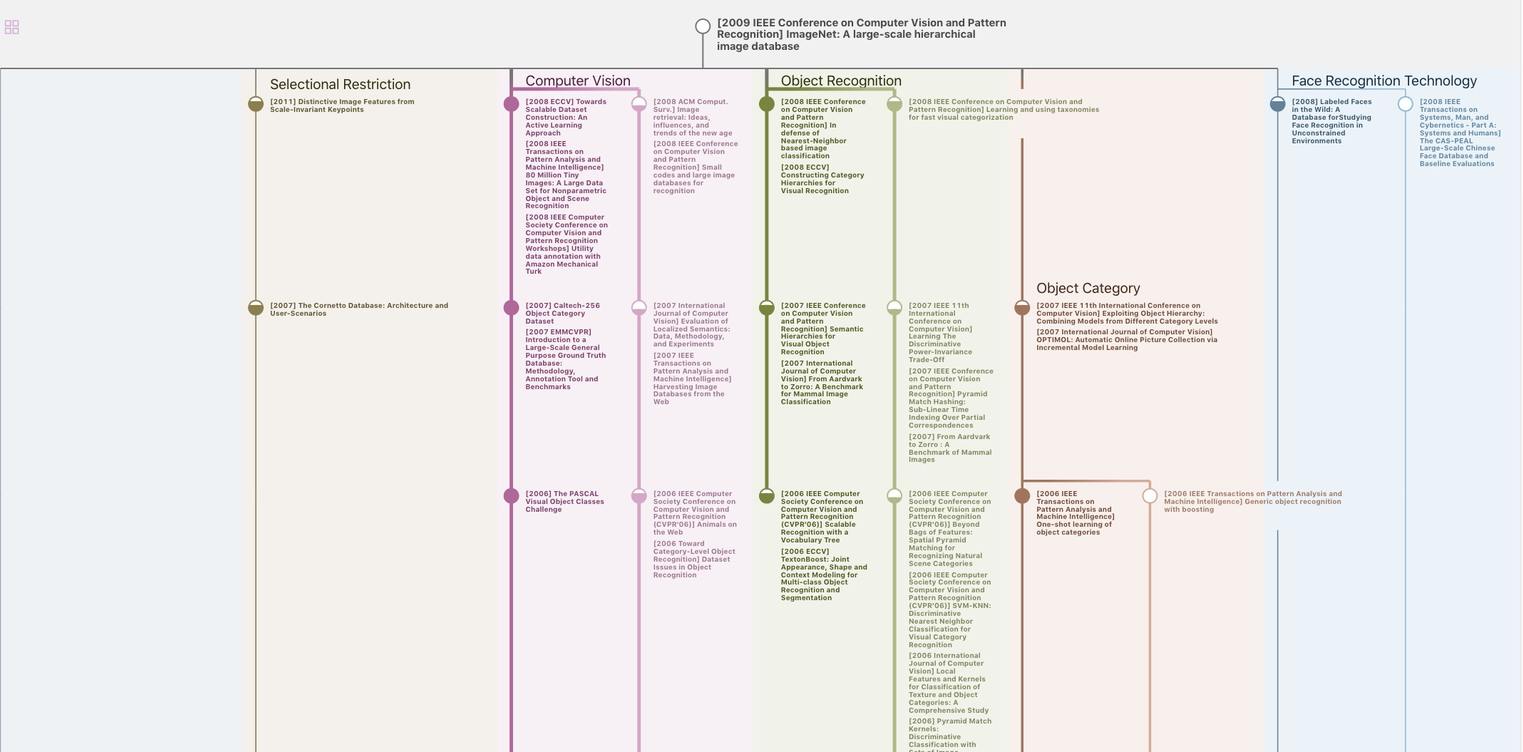
生成溯源树,研究论文发展脉络
Chat Paper
正在生成论文摘要