Domain generalization using contrastive domain discrepancy optimization for interpretation-while-drilling
Journal of Natural Gas Science and Engineering(2022)
摘要
Lithology identification is a crucial component in reservoir characterization and geological exploration. Recently, lithology identification has been studied by many researchers using machine learning methods which can fit complex models. Most of the previous studies develop machine learning models in the premise of independent and identical distribution, i.e., the collected logging data has the same probability distribution even if it comes from different wells. In this way, the model trained on multiple drilled wells could be directly used in a new well. However, this assumption is invalid in practice since there is always a large difference in depositional environment and logging equipment. Therefore, the model trained on the interpreted data of drilled wells might not perform well in interpreting the logging data of a new well, especially while drilling. In this study, we investigate the domain generalization problem in lithology identification, where the logging data of the new unseen well is unavailable and the distribution varies greatly in different wells, to ensure the prediction accuracy on a new unseen well. To tackle such a problem, we propose a contrastive domain discrepancy based adversarial autoencoder (CDD-AAE) framework. Specifically, we utilize adversarial autoencoder to extract domain invariant features by imposing contrastive domain discrepancy objective in the latent space, i.e., the maximum mean discrepancy of inter-class is maximized, and the maximum mean discrepancy of intra-class is minimized. In addition, to avoid the over-fitting problem, a prior distribution is introduced to regularize the latent space in an adversarial training manner. Finally, we conduct extensive experiments on the logging data collected from Jiyang Depression, Bohai Bay Basin to certify the effectiveness of our proposed model. The experiments results demonstrate that our method achieves the best performance of 70.4% on average. Specifically, the macro recall of each target well are 68.7%, 75.3%, 65.4% and 72.2%, respectively. Besides, our method obtains relatively balanced predictions for all lithology types and achieves, indicating that our method can effectively mitigate the domain discrepancy and learn the discriminative features.
更多查看译文
关键词
Logging-while-drilling,Lithology identification,Machine learning,Domain generalization
AI 理解论文
溯源树
样例
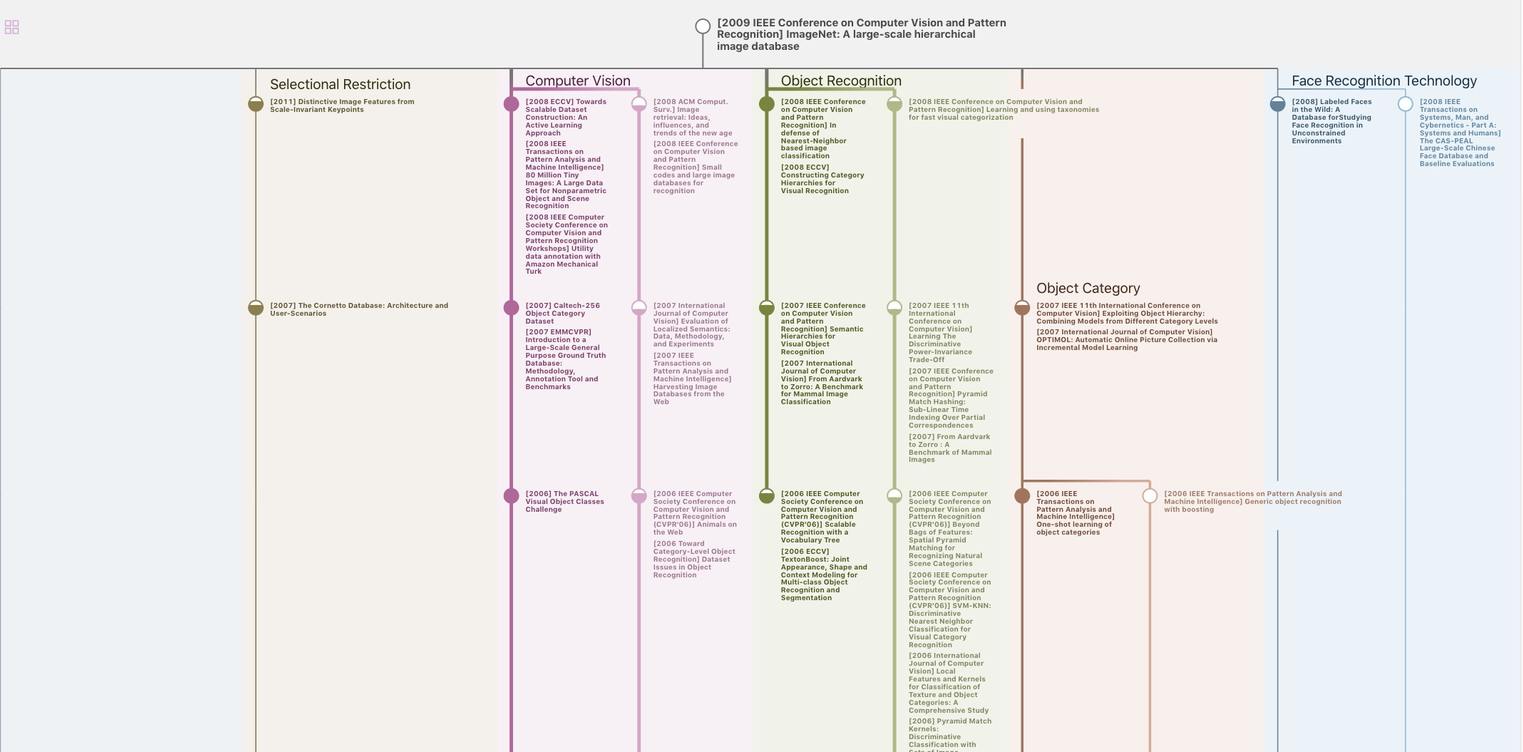
生成溯源树,研究论文发展脉络
Chat Paper
正在生成论文摘要