Utilization of cell-free DNA fragmentomics in minimal residual disease detection for non-small cell lung cancer.
Journal of Clinical Oncology(2022)
摘要
3038 Background: Non-small-cell lung cancer (NSCLC) is the leading cause of worldwide cancer-related deaths. Currently, ̃30-55% of the NSCLC patients develop recurrence due to minimal residual disease (MRD) after receiving surgical resection of the tumor. Therefore, there is an urgent clinical need to accurately predict the MRD risk in post-surgical NSCLC patients. However, the current targeted ctDNA mutation profiling method is limited by the cost of design and synthesis of patient-specific panels and relatively low sensitivity. Cell-free DNA (cfDNA) fragmentomics have recently shown great accuracy and affordability in early cancer detection. This retrospective study aims to develop an ultra-sensitive and affordable fragmentomic assay for MRD detection in NSCLC patients. Methods: A total of 116 NSCLC patients (54 stage I, 21 stage II, 40 stage III and 1 stage IV), who received curative surgical resections (32 patients relapsed during follow-up, median relapse time: 315.5 days), enrolled in the Lung Cancer Tempo-spatial Heterogeneity (LuCaTH) cohort. A total of 231 plasma samples, collected at 7 days post-surgery and 3 months thereafter, were used for whole-genome sequencings (̃5X). The cfDNA fragment size profile was used to fit a Regularized Cox Regression model. A leave-one-out cross-validation (LOOCV) was used to evaluate the model’s predictive performance, and the optimal cutoff for predicting relapse was determined by identifying the Youden index to the predicted relative risk for all samples. Results: Our machine learning model showed an excellent performance in detecting patients with a high risk of recurrence. At 7 days post-surgery, the high-risk patients detected by our model showed an increased risk of 3 times compared to the low-risk patients (hazard ratio [HR] = 3.2, p < 0.001). Multivariate analysis confirmed that the association between model-determined high-risk status and patient relapses was not affected by the baseline clinical variables (age, sex, smoking, stage, etc.). Furthermore, the longitudinal analysis showed that our model was capable of detecting high-risk patients who were 11 times more likely to develop recurrence, independent from other clinical factors (multivariate HR = 11.8, p < 0.001). Overall, our model was able to identify high-risk status in 26 of 32 relapsed patients (81.2% sensitivity), preceding radiographic relapse by a median of 216 days. Furthermore, the sensitivity for MRD detection reached 91% while combining the model prediction with mutation-based ctDNA results. Conclusions: We have developed a predictive model for patient recurrence by detecting the fragmentomic profiling of plasma cfDNA contributed by the MRD. Despite being limited by the relatively small cohort size, our model has shown great sensitivity in predicting patient recurrence, therefore exhibiting a great potential to guide adjuvant therapy decisions.
更多查看译文
关键词
cell-free lung cancer,lung cancer,minimal residual disease detection,dna,non-small
AI 理解论文
溯源树
样例
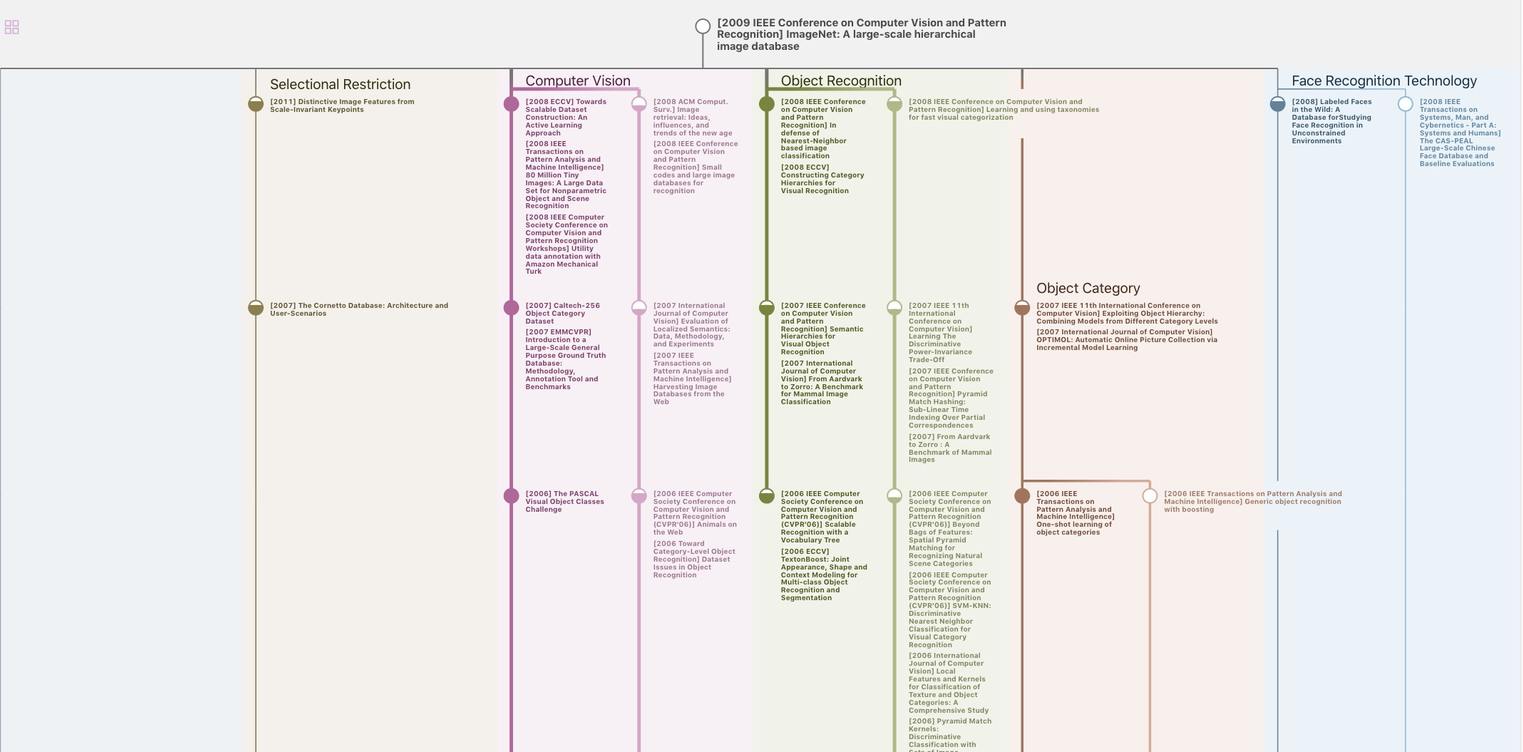
生成溯源树,研究论文发展脉络
Chat Paper
正在生成论文摘要