A Complementary Spectral-Spatial Method for Hyperspectral Image Classification
IEEE TRANSACTIONS ON GEOSCIENCE AND REMOTE SENSING(2022)
摘要
In hyperspectral image (HSI) classification, using spatial information as a supplement to spectral information is an effective way to improve classification accuracy. In this article, a novel robust complementary method using spectral-spatial information is proposed to reduce the information loss in feature extraction, thus improving the classification effect. In short, two complementary feature extraction stages are used to get the probability maps for decision fusion. In the stage of pre-processing feature extraction, we propose an adaptive cubic total variational smoothing method (ACTVSP), which is first proposed and applied in the remote sensing research field, to obtain the first-stage probability map. At the same time, we utilize edge-preserving filtering in the post-processing stage and obtain the second probability map by means of pixel-level classifier. Finally, the probability-like maps obtained in the above two stages are integrated by decision fusion rules. Experiments on ten public datasets show the effectiveness of our proposed method and demonstrate the superiority of distinguishing different land covers on the basis of very few training samples. Therefore, it can be applied to practical applications in different scenes.
更多查看译文
关键词
Feature extraction,Hyperspectral imaging,Image edge detection,Support vector machines,Kernel,Training,Smoothing methods,Adaptive cubic total variation,augmented Lag-range multiplier,feature extraction,hyperspectral classification
AI 理解论文
溯源树
样例
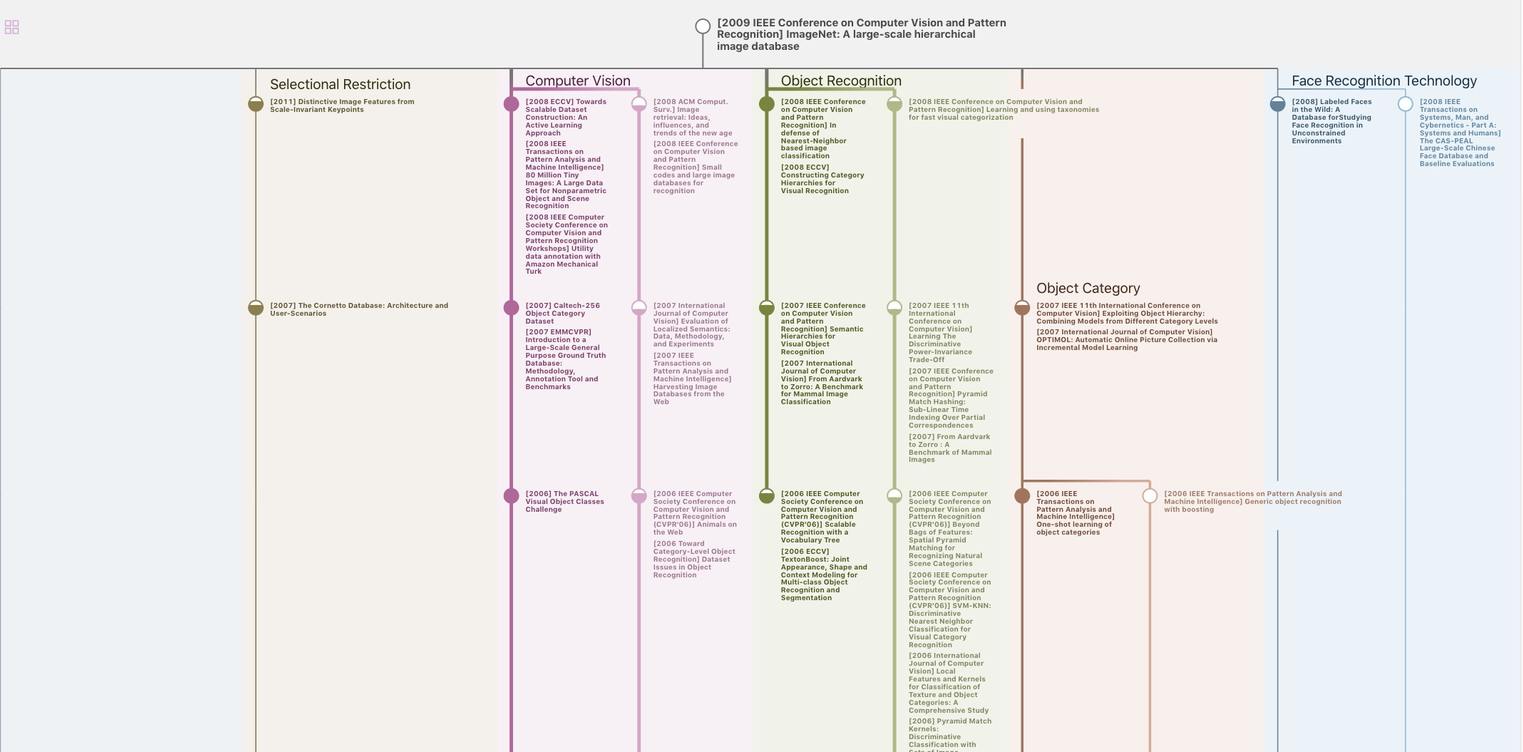
生成溯源树,研究论文发展脉络
Chat Paper
正在生成论文摘要