Self-Supervised Learning for Multimedia Recommendation
IEEE Transactions on Multimedia(2023)
摘要
Learning representations for multimedia content is critical for multimedia recommendation. Current representation learning methods roughly fall into two groups: (1) using the historical interactions to create ID embeddings of users and items, and (2) treating multi-modal data as the side information of items to enrich their ID embeddings. Each user-item interaction offers the supervisory signal to optimize the representation learning by the traditional supervised learning paradigm. Due to the overlook of the multi-modal patterns (
$e.g.$
, co-occurrence of visual, acoustic, textual features in micro-videos a user saw before, and her behavioral features) hidden in the data, these methods are insufficient to create powerful representations and obtain satisfactory recommendation accuracy. To capture multi-modal patterns in the data itself, we go beyond the supervised learning paradigm, and incorporate the idea of self-supervised learning (SSL) into multimedia recommendation. Specifically, SSL consists of two components: (1) data augmentation upon multi-modal contents, where we design three operators — feature dropout (FD), feature masking (FM), feature fine and coarse spaces (FAC) — to generate multiple views of individual items; and (2) contrastive learning, which differentiates the views of an item from the others’ to distill additional supervisory signals. Clearly, SSL enables us to explore and exhibit the underlying relations among modalities, thereby resulting in powerful representations. We denote the generic framework by
Self-supervised Learning-guided Multimedia Recommendation
(SLMRec). Extensive experiments are performed on three real-world datasets, showing that SLMRec achieves significant improvements over several state-of-the-art baselines like LightGCN [1], MMGCN [2]. Further analysis shows how SSL affects recommendation performance.
更多查看译文
关键词
Multimedia recommendation,self-supervised learning,graph neural network,micro-videos
AI 理解论文
溯源树
样例
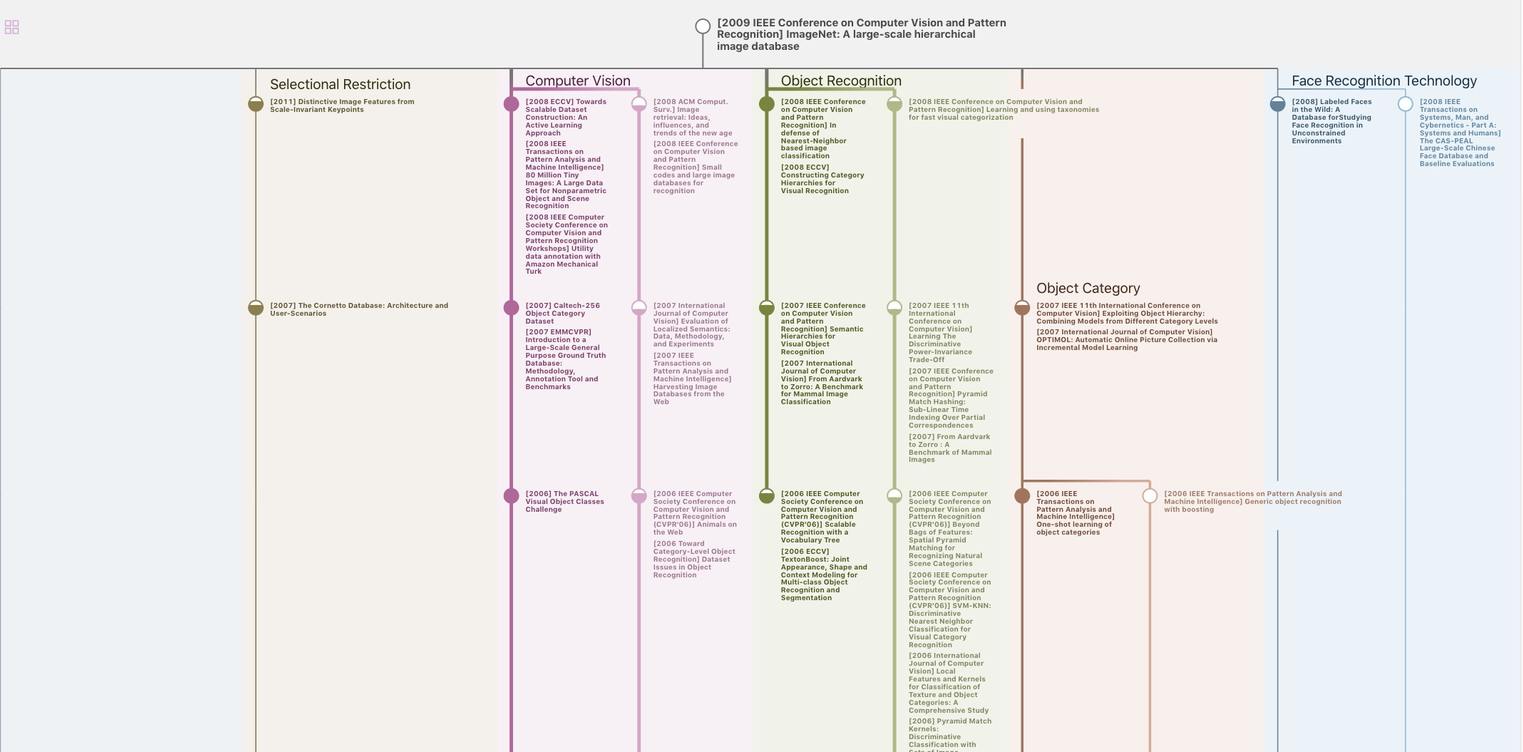
生成溯源树,研究论文发展脉络
Chat Paper
正在生成论文摘要