A Data-Driven Framework for Computationally Efficient Integration of Chemical Kinetics Using Neural Ordinary Differential Equations
SSRN Electronic Journal(2022)
摘要
Abstract A data-driven methodology is introduced for computationally efficient integration of systems of stiff rate equations in chemical kinetics using neural ordinary differential equations (NODE). A systematic algorithm is developed for training data generation and sampling. Subsequently, a novel transformation technique for sampled training data is designed to regularize the neural network parameters, leading to a stable training process. Finally, the NODE network is iteratively trained to learn the accurate neural network representation of chemical kinetics source terms by minimizing the mean absolute error between the true and predicted solutions. The computational efficiency and accuracy of the NODE network are evaluated by simulating the evolution of the thermochemical state of a constant pressure homogeneous hydrogen-air reactor. The combustion of hydrogen in air is described by a finite-rate mechanism including 9 chemical species and 21 reaction steps. The NODE network shows excellent multi-step prediction accuracy for a wide range of initial temperatures and equivalence ratios, spanning the composition space of real flames. The NODE also exhibit a significant reduction in numerical stiffness of the system, enabling the utilization of explicit solvers for integration. The present simulation results using NODE demonstrate up to 70% speed up in computation time compared to direct integration of the chemical mechanism with at most 3.16% relative error in ignition delay time.
更多查看译文
关键词
neural ordinary differential equations,chemical kinetics,computationally efficient integration,differential equations,data-driven
AI 理解论文
溯源树
样例
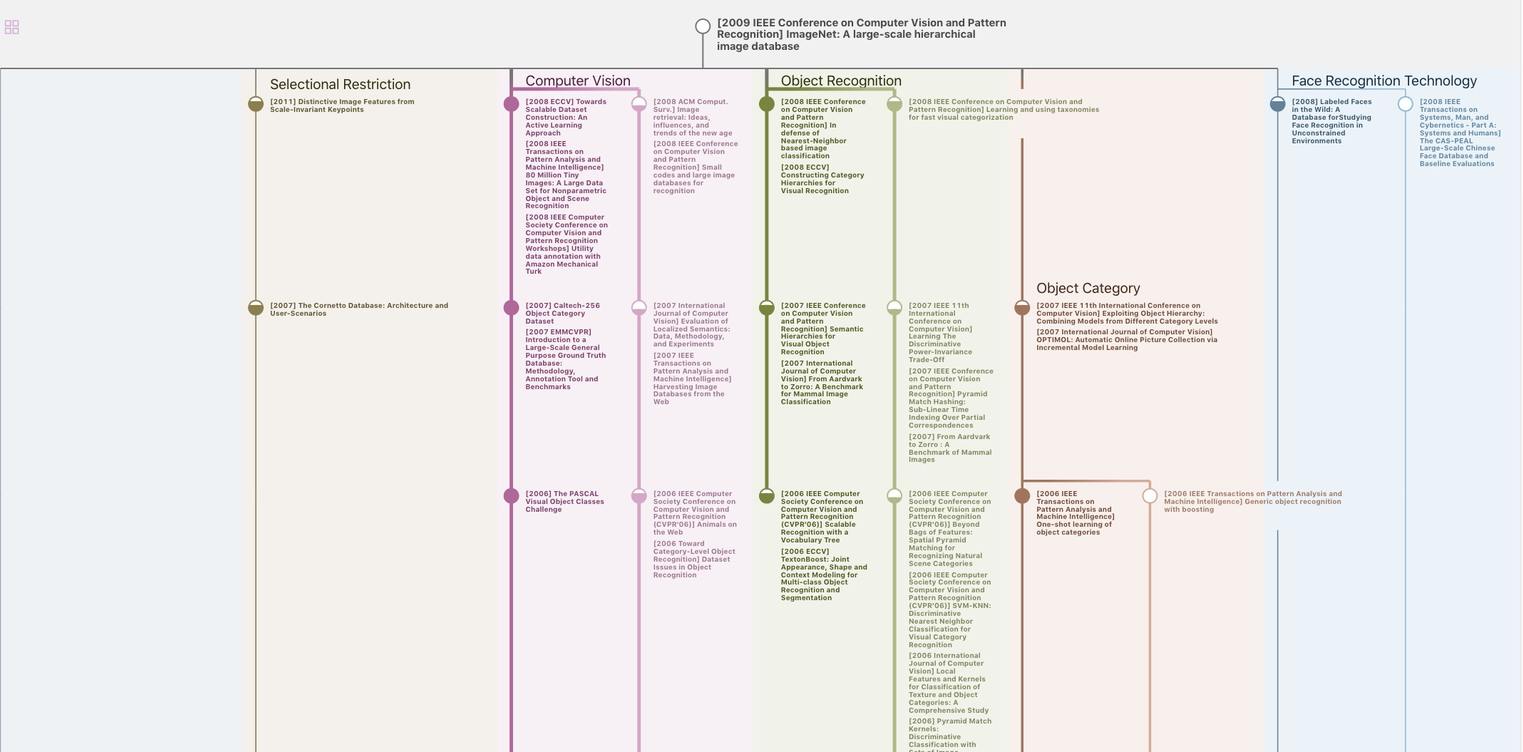
生成溯源树,研究论文发展脉络
Chat Paper
正在生成论文摘要