Cellular Traffic Prediction: A Deep Learning Method Considering Dynamic Nonlocal Spatial Correlation, Self-Attention, and Correlation of Spatiotemporal Feature Fusion
IEEE Transactions on Network and Service Management(2023)
摘要
Cellular traffic prediction will play a key role in the deployment of future smart cities. Although the current traffic prediction methods based on deep learning show better performance than traditional prediction methods, they still have the following problems: (1) In spatial domain, the correlations between cellular traffic features cannot be captured accurately in non-local (including “geographic adjacency” and long-distance) spatial areas. (2) In temporal domain, the correlation of different time-grained features is failed to consider. To address these problems, a deep learning method considering dynamic non-local spatial correlation, self-attention, and correlation of spatio-temporal feature fusion is proposed. In spatial domain, our method can accurately capture the spatial correlation and highlight the contribution of more relevant traffic in the non-local area by designing a NLG-NLAM model. In temporal domain, the correlations of time-periodic features with different granularities are considered to clarify the key roles of different periodic features and eliminate the influence of irrelevant cellular traffic features on the prediction by designing a calibration layer. Experimental results indicate that the proposed method shows better performance than other mainstream prediction methods on three real-world cellular traffic datasets.
更多查看译文
关键词
Cellular traffic prediction,deep learning,non-local spatial correlation,temporal correlation
AI 理解论文
溯源树
样例
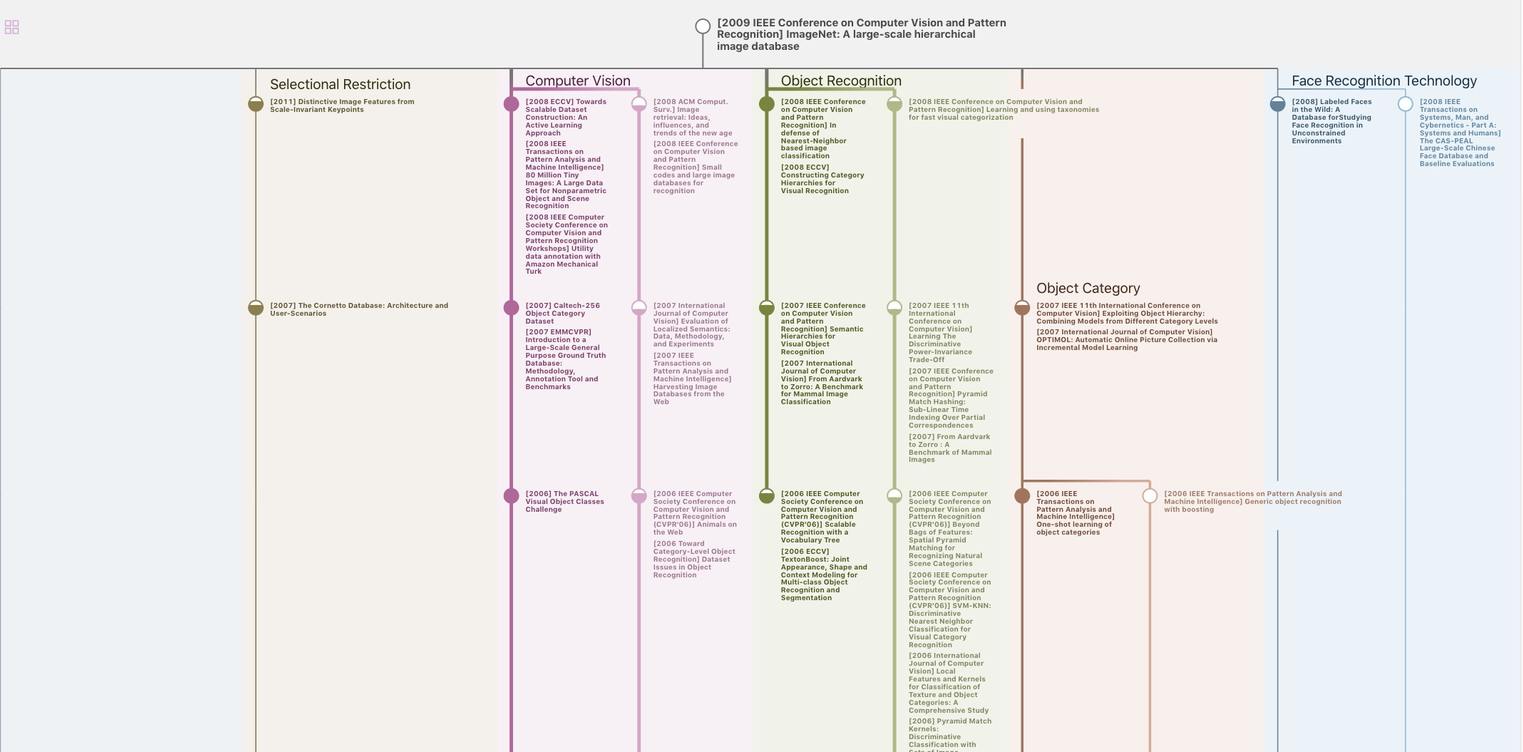
生成溯源树,研究论文发展脉络
Chat Paper
正在生成论文摘要