Collaborative federated learning behind hospitals’ firewalls for predicting histological complete response to neoadjuvant chemotherapy in triple-negative breast cancer.
Journal of Clinical Oncology(2022)
摘要
590 Background: Triple-Negative Breast Cancer (TNBC) is characterized by high metastatic potential and poor prognosis with limited treatment options. Neoadjuvant chemotherapy (NACT) is the standard of care in non-metastastic setting due to the ability to assess pathologic responses providing important prognostic information and guidance in adjuvant therapy decisions. However, the histological response heterogeneity is still poorly understood. We investigate the use of Machine Learning (ML) to predict from diagnosis Whole-Slide Images (WSI) of early TNBC the positive histological Complete Response (pCR) to NACT on surgical specimens. To overcome the known biases of small scale studies while respecting data privacy, we conduct a study in a multi-centric fashion behind hospitals’ firewalls using collaborative Federated Learning (FL). Thereby allowing access to enough TNBC data to sustain a complete response heterogeneity investigation. Methods: We collected in both comprehensive cancer centers: Centre Léon Bérard (A)(n=99) and Institut Curie (B) (n=420), WSI of biopsies performed at diagnosis and relevant clinical variables. We use traditional Multiple Instance Learning pipelines by tiling the matter on each WSI with a pre-trained Neural Network (NN). We train a second NN to predict the NACT pCR using the mean feature of each WSI. ML trainings are performed using either one cohort in isolation (NN Local) or both cohorts using FL. We compare the performance of this federated WSI based model to the best clinical model (Clin.) simulating clinical practice (using grade and Tumor-Infiltrating Lymphocytes (TILs) percentage) on both centers. Results: Performance of models to predict NACT pCR (AUC). All results are evaluated in 5 repeated 4-folds cross validations. Conclusions: The final ML model, that was trained in a privacy preserving fashion on both hospitals, provides better prediction of NACT pCR than current clinical standards. This study shows that 1. Not all relevant information is routinely extracted from WSI and 2. Non simulated FL is possible in Healthcare and gives better results than siloed studies on open medical questions. Additional interpretability results of the model show that it has re-discovered known biomarkers such as TILs and apocrine tumor cells without any tile-level annotation, and hints at potential new biomarkers. [Table: see text]
更多查看译文
关键词
neoadjuvant chemotherapy,triple-negative triple-negative breast cancer,breast cancer
AI 理解论文
溯源树
样例
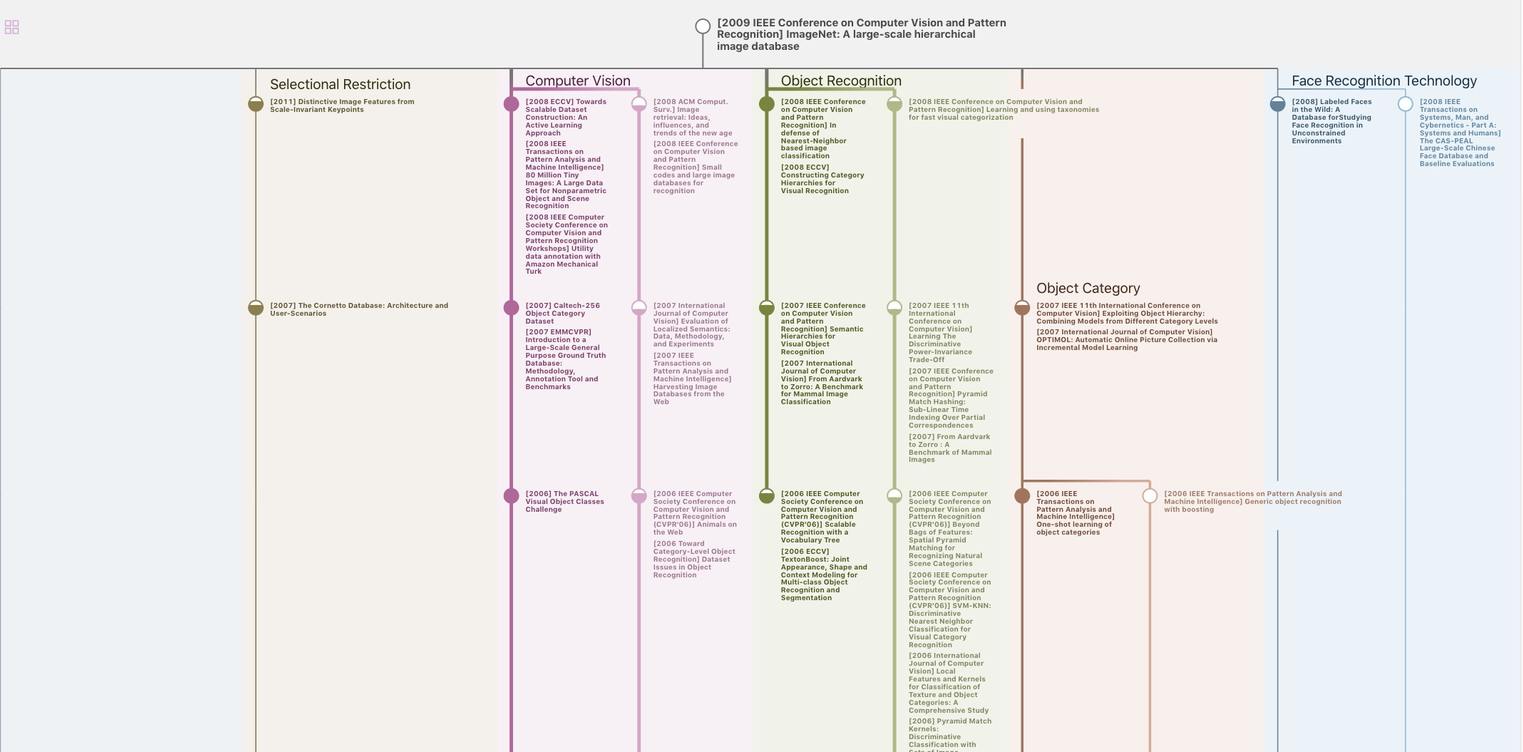
生成溯源树,研究论文发展脉络
Chat Paper
正在生成论文摘要