A reforecasting-based dynamic reserve estimation for variable renewable and demand
Electric Power Systems Research(2022)
摘要
The installed capacity of renewables-based energy sources has been increasing in traditional power systems. In order to accommodate the increased variability and uncertainty associated with the deeper penetration of renewable sources like solar and wind, adjusted amounts of dynamic reserve are needed. Although probabilistic dynamic reserve estimation methods have been previously developed, most of them consider the uncertainty to be represented by parametric density functions that tend to perform poorly under extreme events and, moreover, neglect uncertainty introduced by the forecasting model itself. Toward addressing these limitations, this work presents, for the first time, a dynamic reserve estimation method for flexibility that incorporates non parametric density estimation and a machine learning based reforecasting to provide a day-ahead prediction of the mean and spread of uncertainty around the base forecast. The prediction is, in turn, used to estimate the up and down reserve relative to the base forecast. The present method takes various endogenous and exogenous features, including the calendar variables, as input to estimate the day-ahead reserve. Using a combination of reforecasting and dynamic reserve estimation techniques, the method is shown to adjust better to the dynamic nature of reserve requirements providing only what is needed to accommodate the expected deviations. Considering California Independent System Operator (CAISO) solar, wind and load data over an 18 month period, up to 67% reduction in the amount of reserve capacity needed for a one day reserve and reserve penalty for solar uncertainty is demonstrated. Additionally, the risk of reserve insufficiency in meeting the net demand is reduced by 20% with the proposed method.
更多查看译文
关键词
Renewable energy, Solar photovoltaics, Wind, Net load, Risk management, Machine learning
AI 理解论文
溯源树
样例
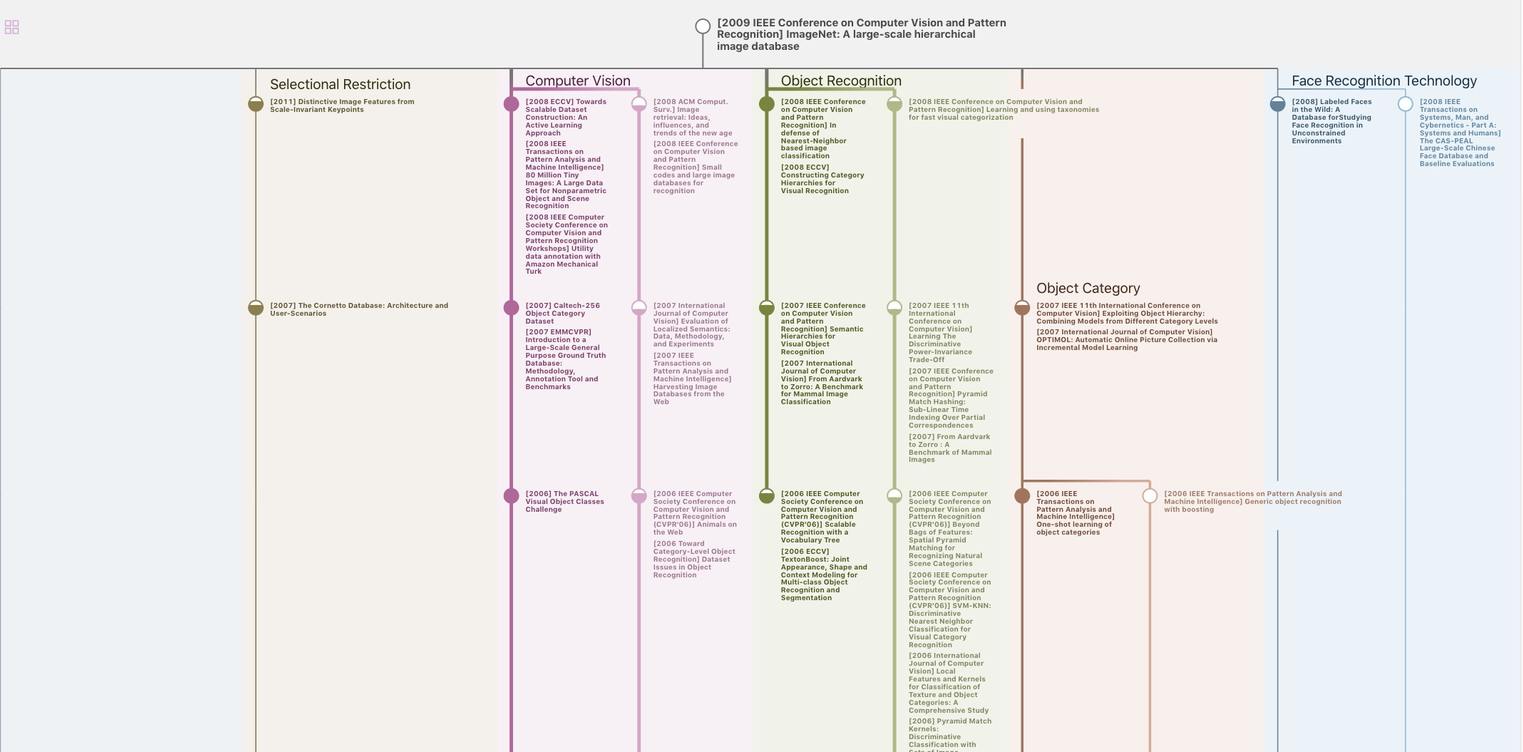
生成溯源树,研究论文发展脉络
Chat Paper
正在生成论文摘要