Abstract 1939: Multimodal prediction of metastatic relapse using federated deep learning outperforms state-of-the-art methods in soft-tissue sarcoma
Cancer Research(2022)
摘要
Abstract Soft Tissue sarcomas (STS) are a group of heterogeneous and complex diseases. Being able to predict the appearance of metastases is key to inform clinical decisions, especially the prescription of perioperative chemotherapy. While multiple methods including multivariable nomograms have been developed to tackle this issue, we leveraged artificial intelligence (AI) to extract information from raw images with Deep-Learning. In this study, we developed SarcNet: a multimodal deep-learning algorithm based on histological slides and clinical variables to predict metastatic relapse in patients affected with limbs and trunk wall STS. Two independent series were investigated simultaneously: Centre Léon Bérard, n=221 and Institut Bergonié, n=390. This study was able to gather a sufficient number of patients in this rare disease by training all the algorithms in a multicentric fashion using Federated Learning (FL). It is one of the first real-world applications of FL in Healthcare which allows to keep patients’ data behind hospital firewalls. The SarcNet algorithm achieved unprecedented performance. By leveraging an AI-driven analysis of digitized whole slide images in addition to clinical variables, our multimodal model achieved a 0.80 AUC to predict 5-year MFS (metastatic free survival) compared to 0.77 for Sarculator (current state-of-the-art nomogram). It also greatly outperformed current standard of care practices like FNCLCC grading that reached an AUC of 0.70. Interpretability investigations of the SarcNet model highlighted histological patterns that drive the prediction of metastatic relapse such as atypia, tumoral cellularity and mitosis. This model could lead to more informed clinical decisions by identifying high-risk patients that could benefit from perioperative chemotherapy. Finally, deeper investigations of the model and the highlighted biomarkers could lead to a better understanding of metastatic relapse in STS Performance of models to predict 5y MFS (AUC evaluated in 5 repeated 5-fold cross validation) Methods Whole cohort (n=611) IB (n=390) CLB (n=221) SarcNet 0.800, 95% CI [0.786-0.814] 0.770, 95% CI [0.750-0.791] 0.842, 95% CI [0.824-0.859] Sarculator 0.767, 95% CI [0.752-0.783] 0.736, 95% CI [0.718-0.755] 0.808, 95% CI [0.785-0.831] FNCLCC (French Federation of Centers for the Fight against Cancer) grading 0.699, 95% CI [0.684-0.714] 0.723, 95% CI [0.708-0.738] 0.637, 95% CI [0.612-0.663] Citation Format: Charles Maussion, Jean-Michel Coindre, Jean-Yves Blay, Kathryn Schutte, Axel Camara, François Le Loarer, Raul Perret, Myriam Jean-Denis, Alexandra Meurgey, Françoise Ducimetiere, Antoine Giraud, Jean-Baptiste Courrèges, Clément Gautier, Etienne Bendjebbar, Jean Du Terrail, Antoine Italiano, Marie Karanian. Multimodal prediction of metastatic relapse using federated deep learning outperforms state-of-the-art methods in soft-tissue sarcoma [abstract]. In: Proceedings of the American Association for Cancer Research Annual Meeting 2022; 2022 Apr 8-13. Philadelphia (PA): AACR; Cancer Res 2022;82(12_Suppl):Abstract nr 1939.
更多查看译文
关键词
sarcoma,multimodal prediction,metastatic relapse,state-of-the-art,soft-tissue
AI 理解论文
溯源树
样例
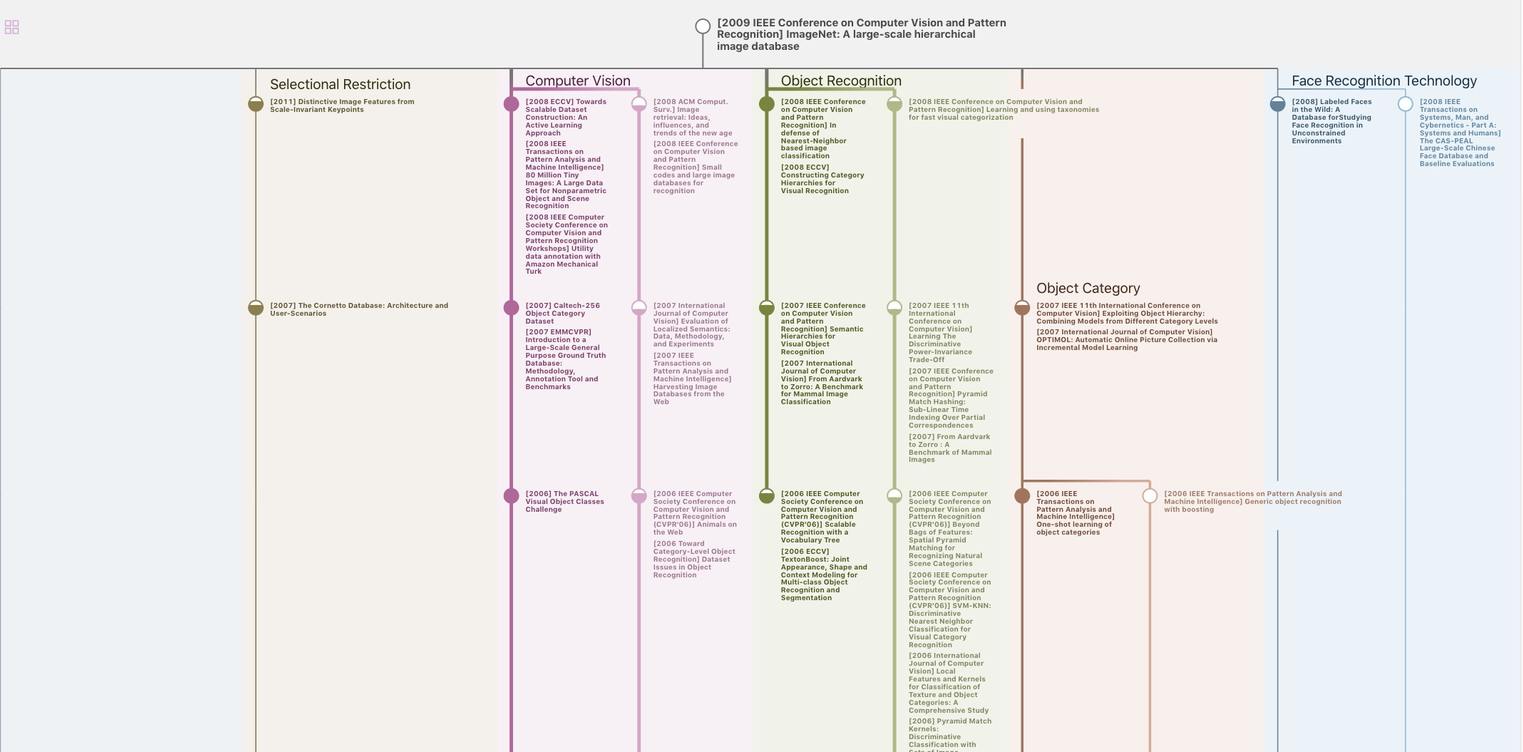
生成溯源树,研究论文发展脉络
Chat Paper
正在生成论文摘要