Abstract 467: Integrative deep learning analysis identifies cross-talk between morphology, mutation, and clinical variables for colon adenocarcinoma patient outcomes
Cancer Research(2022)
摘要
Abstract Colorectal cancer is one of the most common cancers in both men and women. Patient stratification in colorectal cancers is challenging and affects therapy decisions and patient care. In this study, we develop an integrative Inception V3-based deep learning approach to stratify TCGA-COAD patients from H&E images, showing that combining deep learning extracted morphological features with clinical and mutational status improves patient stratification. Morphological features within tumor regions can distinguish patients by short or long overall survival (OS) (OS<3 years v.s. OS>5 years, AUC 0.81±0.12), while they are less informative for intermediate OS (3<OS<5 years). Models that integrate morphological features with additional data modalities yield superior stratification to those that use only a single datatype or that use only clinical information and genomic markers. Remarkably, combining deep learning morphological features with clinical information and genomic markers also boosts stratification of patients with 3<OS<5 years, both at the 3-year time point (p <0.001) and 5-year time point (p <0.001). We validated our model on an external dataset (OS<3 years v.s. OS>5 years, AUC 0.78±0.20), and again found that combining morphological features and clinical data is superior to only using a single datatype. Our deep learning models were also able to perform reliable tumor segmentation (AUC>0.92). However, survival predictions based on deep learning tumor segmentation were inferior to those based on pathologist tumor annotations (OS<3 years v.s. OS>5 years, AUC 0.79±0.14). Furthermore, we show that the cross-talk between different data modalities is informative of patient risk. More precisely, models that directly combine image and clinical features performed superior to a naïve Bayes classifier combining predictions of models trained on each data type (integrative model AUC=0.81±0.06 and naïve Bayes AUC=0.76±0.11, p<0.01). Our results show integrative analysis utilizes the cross-talk between data modalities to boost patient risk stratification, and that deep learning is an efficient methodology for taking advantage of cross-talk between various data modalities. Citation Format: Jie Zhou, Ali Foroughi pour, Rafic Beydoun, Hany Deirawan, Fayez Daaboul, Fahad Shabbir Ahmed, Jeffrey H. Chuang. Integrative deep learning analysis identifies cross-talk between morphology, mutation, and clinical variables for colon adenocarcinoma patient outcomes [abstract]. In: Proceedings of the American Association for Cancer Research Annual Meeting 2022; 2022 Apr 8-13. Philadelphia (PA): AACR; Cancer Res 2022;82(12_Suppl):Abstract nr 467.
更多查看译文
关键词
colon adenocarcinoma patient outcomes,deep learning,cross-talk
AI 理解论文
溯源树
样例
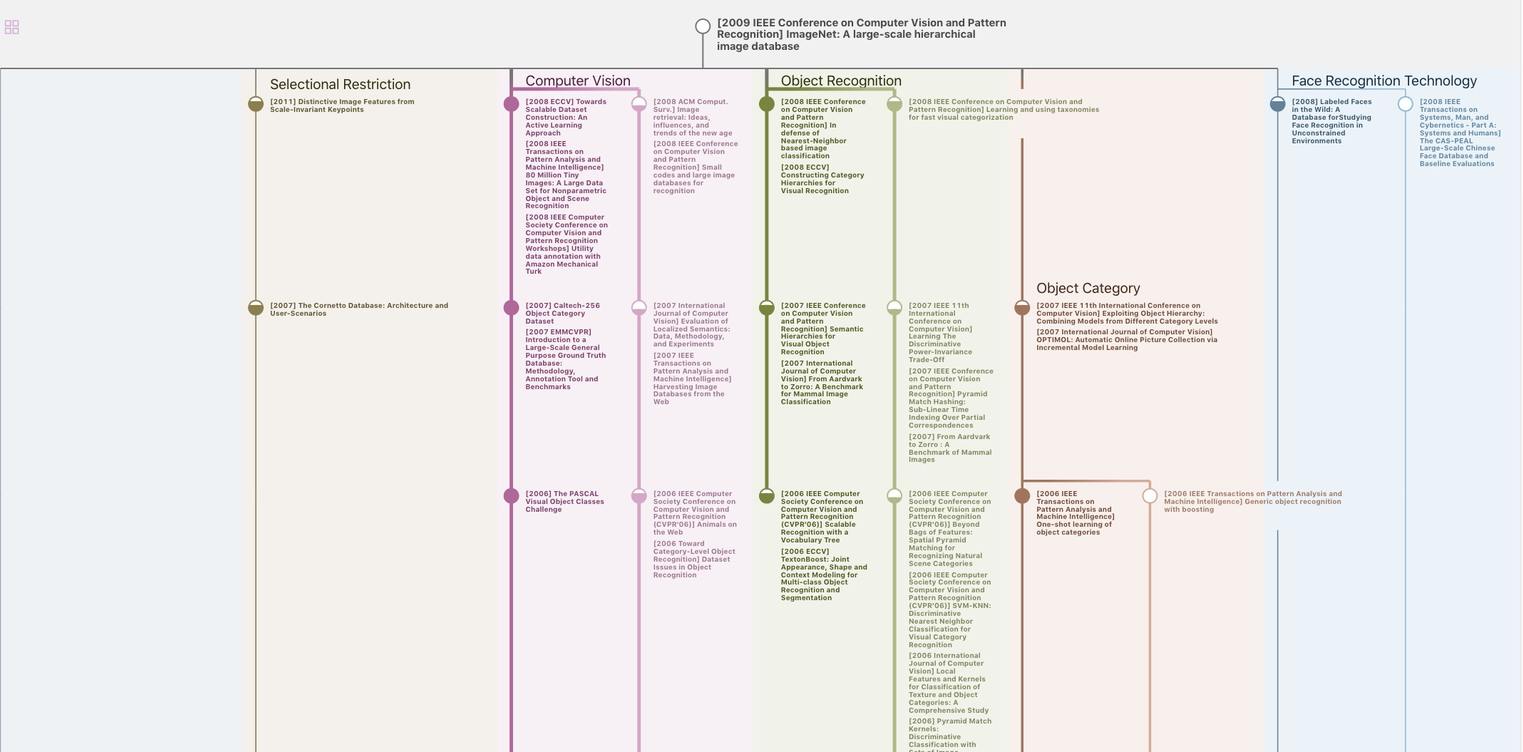
生成溯源树,研究论文发展脉络
Chat Paper
正在生成论文摘要