Reinforcement learning based proportional-integral-derivative controllers design for consensus of multi-agent systems.
ISA transactions(2023)
摘要
This paper develops a novel Proportional-Integral-Derivative (PID) tuning method for multi-agent systems with a reinforced self-learning capability for achieving the optimal consensus of all agents. Unlike the traditional model-based and data-driven PID tuning methods, the developed PID self-learning method updates the controller parameters by actively interacting with unknown environment, with the outcomes of guaranteed consensus and performance optimization of agents. Firstly, the PID control-based consensus problem of multi-agent systems is formulated. Then, finding the PID gains is converted into solving a nonzero-sum game problem, thus an off-policy Q-learning algorithm with the critic-only structure is proposed to update the PID gains using only data, without the knowledge of dynamics of agents. Finally, simulations are given to verify the effectiveness of the proposed method.
更多查看译文
关键词
Consensus control,Multi-agent systems,Neural networks,Nonzero-sum game,PID control,Reinforcement learning
AI 理解论文
溯源树
样例
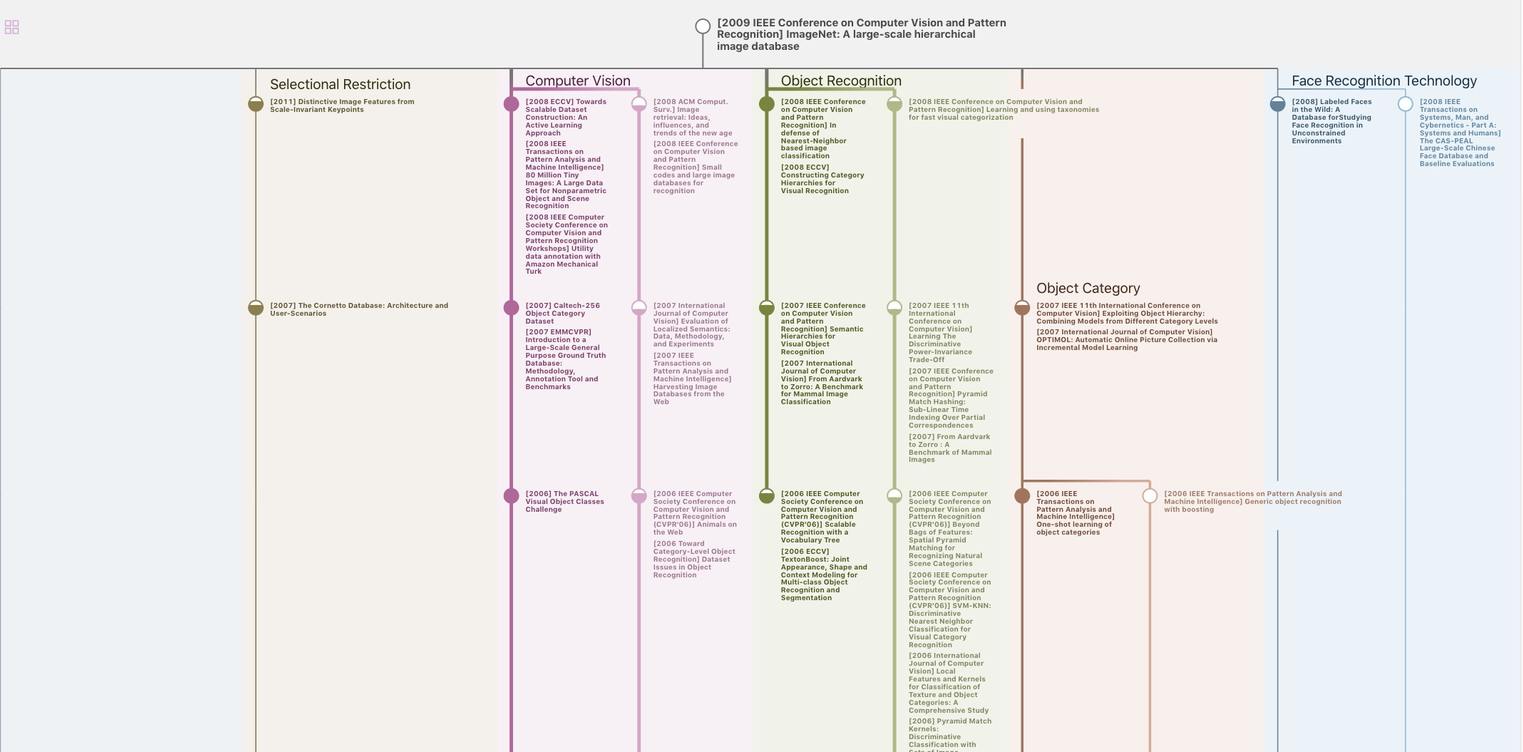
生成溯源树,研究论文发展脉络
Chat Paper
正在生成论文摘要