Multi-branch fusion auxiliary learning for the detection of pneumonia from chest X-ray images
Computers in Biology and Medicine(2022)
摘要
Lung infections caused by bacteria and viruses are infectious and require timely screening and isolation, and different types of pneumonia require different treatment plans. Therefore, finding a rapid and accurate screening method for lung infections is critical. To achieve this goal, we proposed a multi-branch fusion auxiliary learning (MBFAL) method for pneumonia detection from chest X-ray (CXR) images. The MBFAL method was used to perform two tasks through a double-branch network. The first task was to recognize the absence of pneumonia (normal), COVID-19, other viral pneumonia and bacterial pneumonia from CXR images, and the second task was to recognize the three types of pneumonia from CXR images. The latter task was used to assist the learning of the former task to achieve a better recognition effect. In the process of auxiliary parameter updating, the feature maps of different branches were fused after sample screening through label information to enhance the model’s ability to recognize case of pneumonia without impacting its ability to recognize normal cases. Experiments show that an average classification accuracy of 95.61% is achieved using MBFAL. The single class accuracy for normal, COVID-19, other viral pneumonia and bacterial pneumonia was 98.70%, 99.10%, 96.60% and 96.80%, respectively, and the recall was 97.20%, 98.60%, 96.10% and 89.20%, respectively, using the MBFAL method. Compared with the baseline model and the model constructed using the above methods separately, better results for the rapid screening of pneumonia were achieved using MBFAL.
更多查看译文
关键词
Pneumonia,Deep learning,Auxiliary learning,Multi-task learning,Feature fusion
AI 理解论文
溯源树
样例
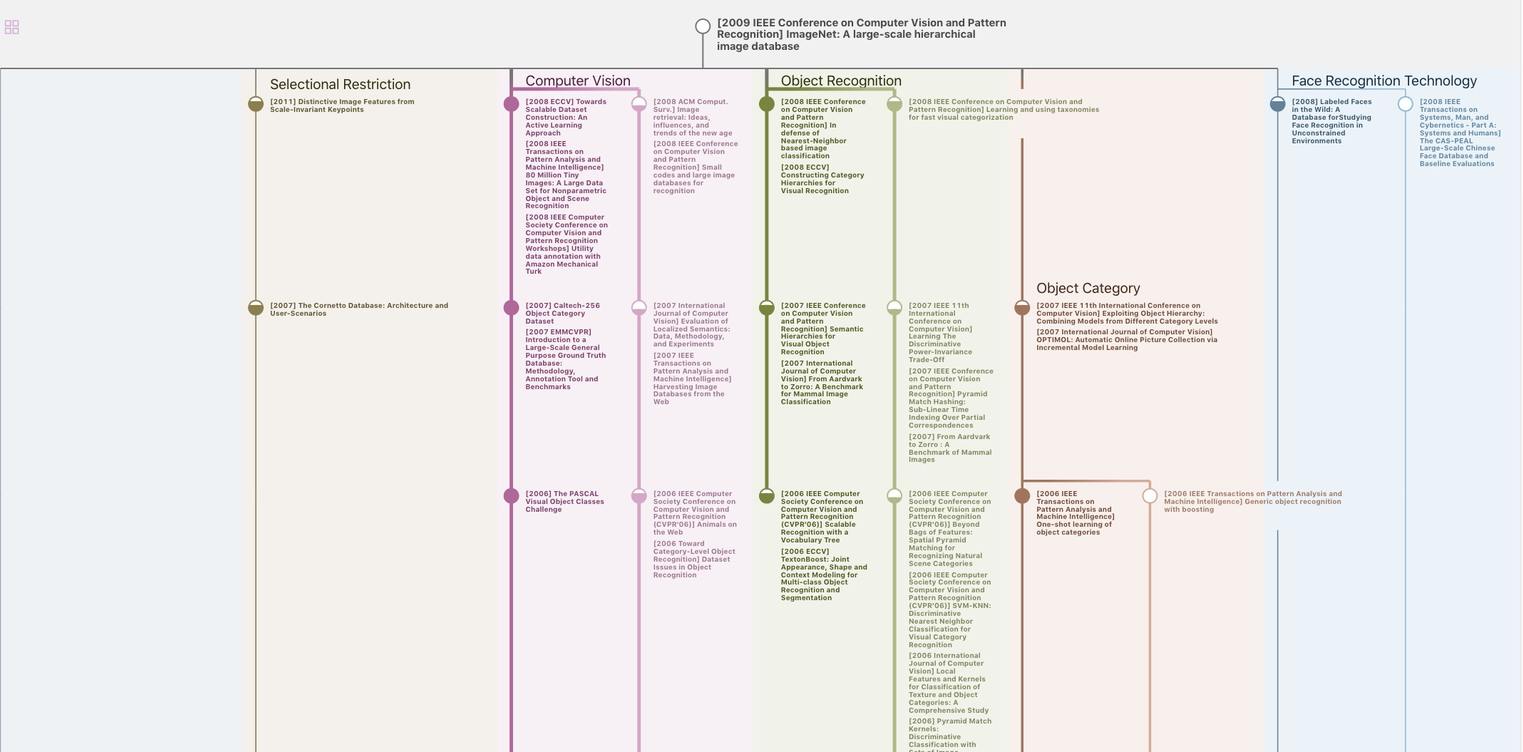
生成溯源树,研究论文发展脉络
Chat Paper
正在生成论文摘要