Learning disentangled representations for controllable human motion prediction
PATTERN RECOGNITION(2024)
摘要
Generative model-based motion prediction techniques have recently realized predicting controlled human motions, such as predicting multiple upper human body motions with similar lower-body motions. However, to achieve this, the state-of-the-art methods require either subsequently learning mapping functions to seek similar motions or training the model repetitively to enable control over the desired portion of body. In this paper, we propose a novel framework to learn disentangled representations for controllable human motion prediction. Our task is to predict multiple future human motions based on the past observed sequence, with the control of partial-body movements. Our network involves a conditional variational auto-encoder (CVAE) architecture to model full-body human motion, and an extra CVAE path to learn only the corresponding partial -body (e.g., lower-body) motion. Specifically, the inductive bias imposed by the extra CVAE path encourages two latent variables in two paths to respectively govern separate representations for each partial-body motion. With a single training, our model is able to provide two types of controls for the generated human motions: (i) strictly controlling one portion of human body and (ii) adaptively controlling the other portion, by sampling from a pair of latent spaces. Additionally, we extend and adapt a sampling strategy to our trained model to diversify the controllable predictions. Our framework also potentially allows new forms of control by flexibly customizing the input for the extra CVAE path. Extensive experimental results and ablation studies demonstrate that our approach is capable of predicting state-of-the-art controllable human motions both qualitatively and quantitatively.
更多查看译文
关键词
Stochastic motion prediction,Deep generative model,Disentanglement learning
AI 理解论文
溯源树
样例
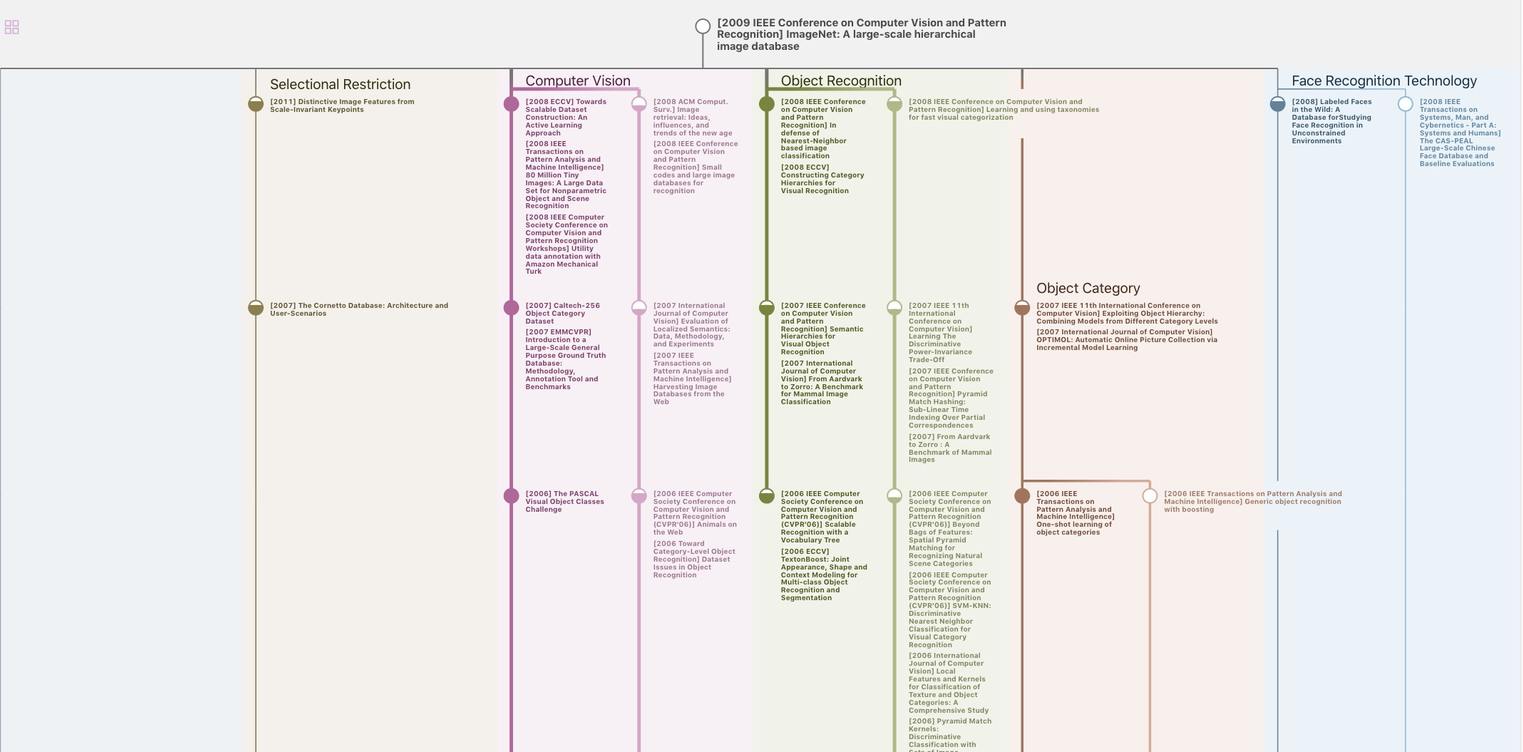
生成溯源树,研究论文发展脉络
Chat Paper
正在生成论文摘要