Safe Reinforcement Learning via Confidence-Based Filters
arxiv(2022)
摘要
Ensuring safety is a crucial challenge when deploying reinforcement learning (RL) to real-world systems. We develop confidence-based safety filters, a control-theoretic approach for certifying state safety constraints for nominal policies learned via standard RL techniques, based on probabilistic dynamics models. Our approach is based on a reformulation of state constraints in terms of cost functions, reducing safety verification to a standard RL task. By exploiting the concept of hallucinating inputs, we extend this formulation to determine a "backup" policy that is safe for the unknown system with high probability. Finally, the nominal policy is minimally adjusted at every time step during a roll-out towards the backup policy, such that safe recovery can be guaranteed afterwards. We provide formal safety guarantees, and empirically demonstrate the effectiveness of our approach.
更多查看译文
关键词
backup policy,certifying state safety constraints,confidence-based filters,confidence-based safety filters,control-theoretic approach,cost functions,deploying reinforcement learning,ensuring safety,formal safety guarantees,nominal policy,probabilistic dynamics models,real-world systems,safe recovery,safe reinforcement learning,safety verification,standard RL task,standard RL techniques,state constraints,unknown system
AI 理解论文
溯源树
样例
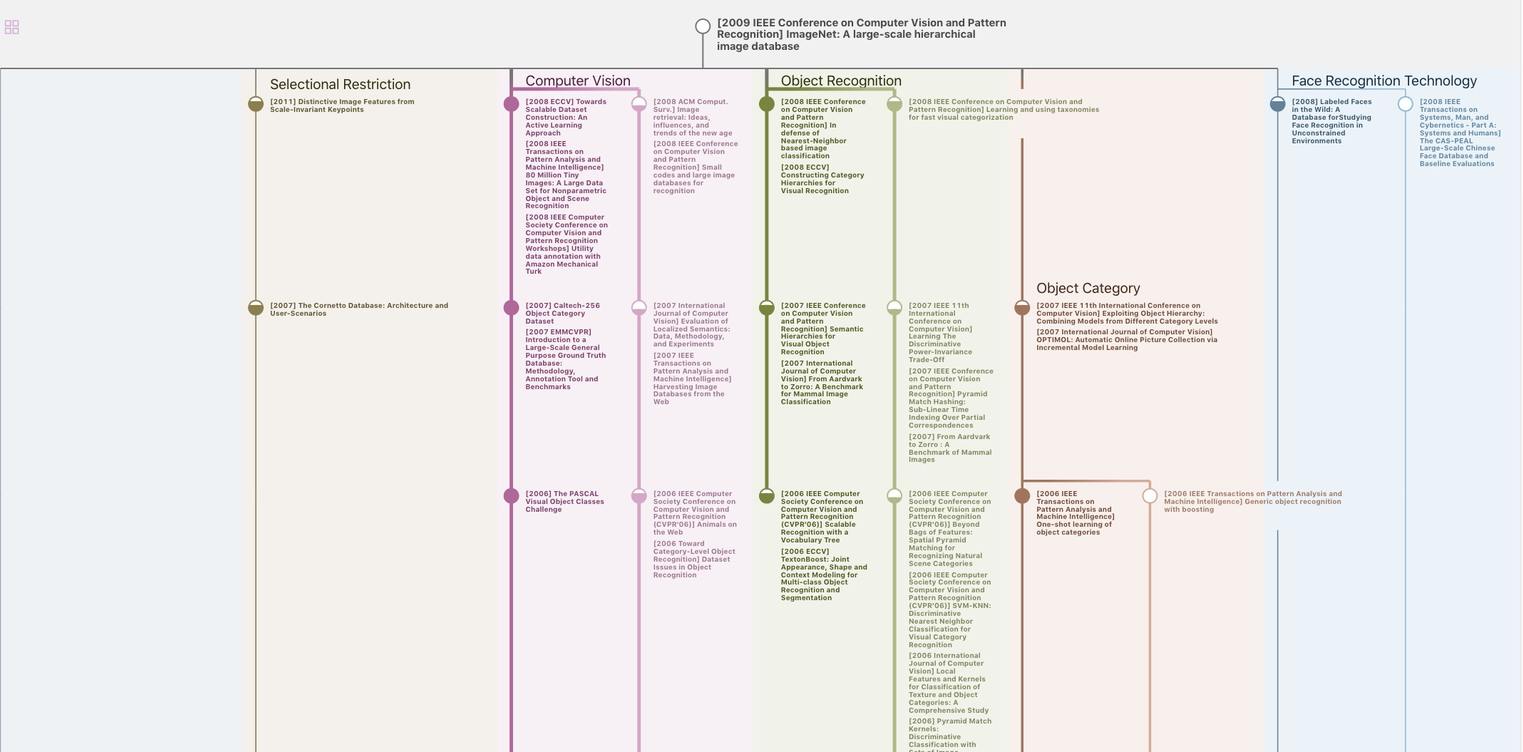
生成溯源树,研究论文发展脉络
Chat Paper
正在生成论文摘要