Learning Switching Criteria for Sim2Real Transfer of Robotic Fabric Manipulation Policies.
IEEE Conference on Automation Science and Engineering (CASE)(2022)
摘要
Simulation-to-reality transfer has emerged as a popular and highly successful method to train robotic control policies for a wide variety of tasks. However, it is often challenging to determine when policies trained in simulation are ready to be transferred to the physical world. Deploying policies that have been trained with very little simulation data can result in unreliable and dangerous behaviors on physical hardware. On the other hand, excessive training in simulation can cause policies to overfit to the visual appearance and dynamics of the simulator. In this work, we study strategies to automatically determine when policies trained in simulation can be reliably transferred to a physical robot. We specifically study these ideas in the context of robotic fabric manipulation, in which successful sim2real transfer is especially challenging due to the difficulties of precisely modeling the dynamics and visual appearance of fabric. Results in a fabric smoothing task suggest that our switching criteria correlate well with performance in real. In particular, our confidence-based switching criteria achieve average final fabric coverage of 87.2-93.7% within 55-60% of the total training budget. See https://tinyurl.com/lsc-case for code and supplemental materials.
更多查看译文
关键词
deploying policies,simulation data,unreliable behaviors,dangerous behaviors,physical hardware,excessive training,physical robot,successful sim2real transfer,fabric smoothing task,switching criteria,average final fabric coverage,total training budget,robotic fabric manipulation policies,simulation-to-reality transfer,popular method,highly successful method,robotic control policies,physical world
AI 理解论文
溯源树
样例
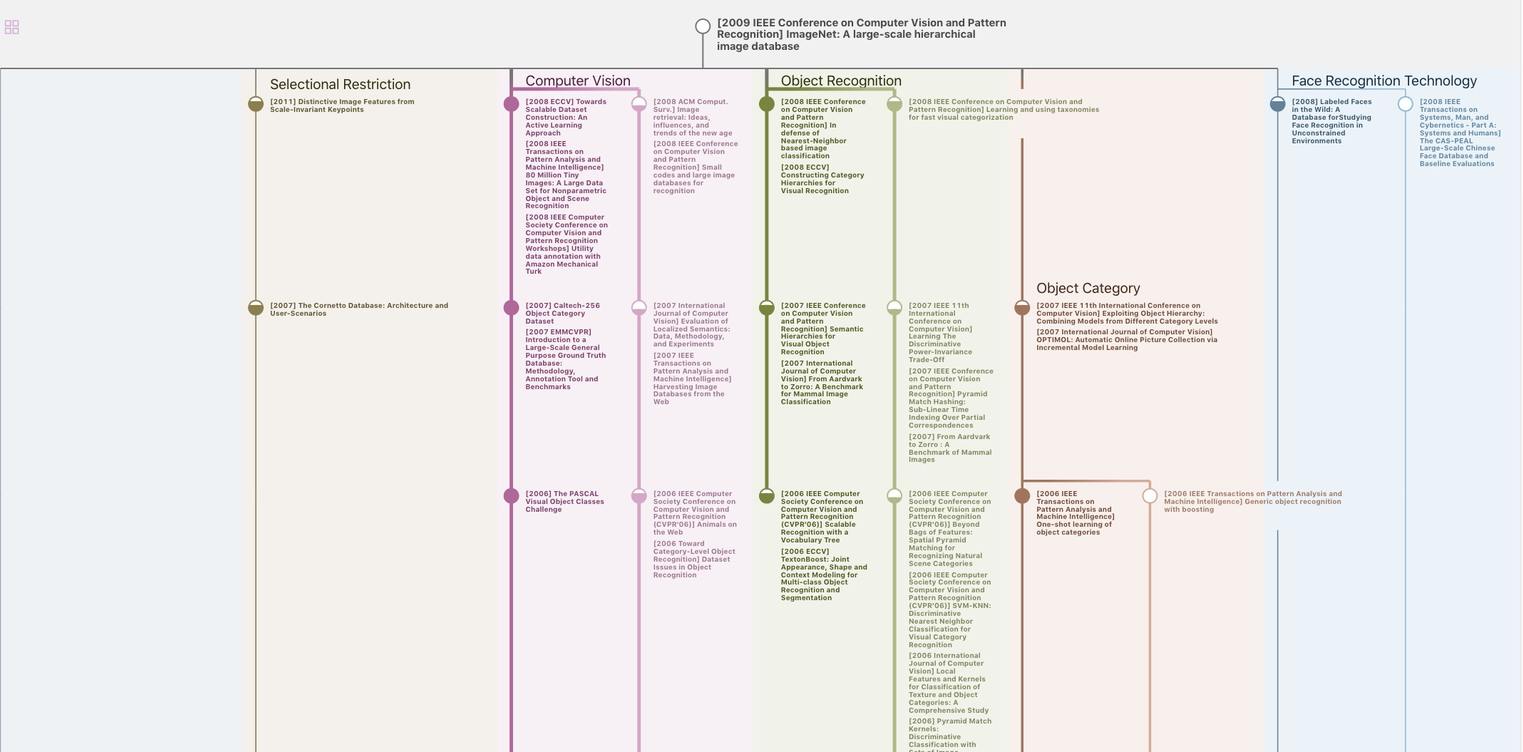
生成溯源树,研究论文发展脉络
Chat Paper
正在生成论文摘要