Id-Free Person Similarity Learning
IEEE Conference on Computer Vision and Pattern Recognition(2022)
摘要
Learning a unified person detection and re-identification model is a key component of modern trackers. However, training such models usually relies on the availability of training images / videos that are manually labeled with both person boxes and their identities. In this work, we explore training such a model by only using person box annotations, thus removing the necessity of manually labeling a training dataset with additional person identity annotation as these are expensive to collect. To this end, we present a contrastive learning framework to learn person similarity without using manually labeled identity annotations. First, we apply image-level augmentation to images on public person detection datasets, based on which we learn a strong model for general person detection as well as for short-term person re-identification. To learn a model capable of longerterm re-identification, we leverage the natural appearance evolution of each person in videos to serve as instance-level appearance augmentation in our contrastive loss formulation. Without access to the target dataset or person identity annotation, our model achieves competitive results compared to existing fully-supervised state-of-the-art methods on both person search and person tracking tasks. Our model also shows promising results for saving the annotation cost that is needed to achieve a certain level of performance on the person search task.
更多查看译文
关键词
Self-& semi-& meta- Motion and tracking, Recognition: detection,categorization,retrieval, Representation learning
AI 理解论文
溯源树
样例
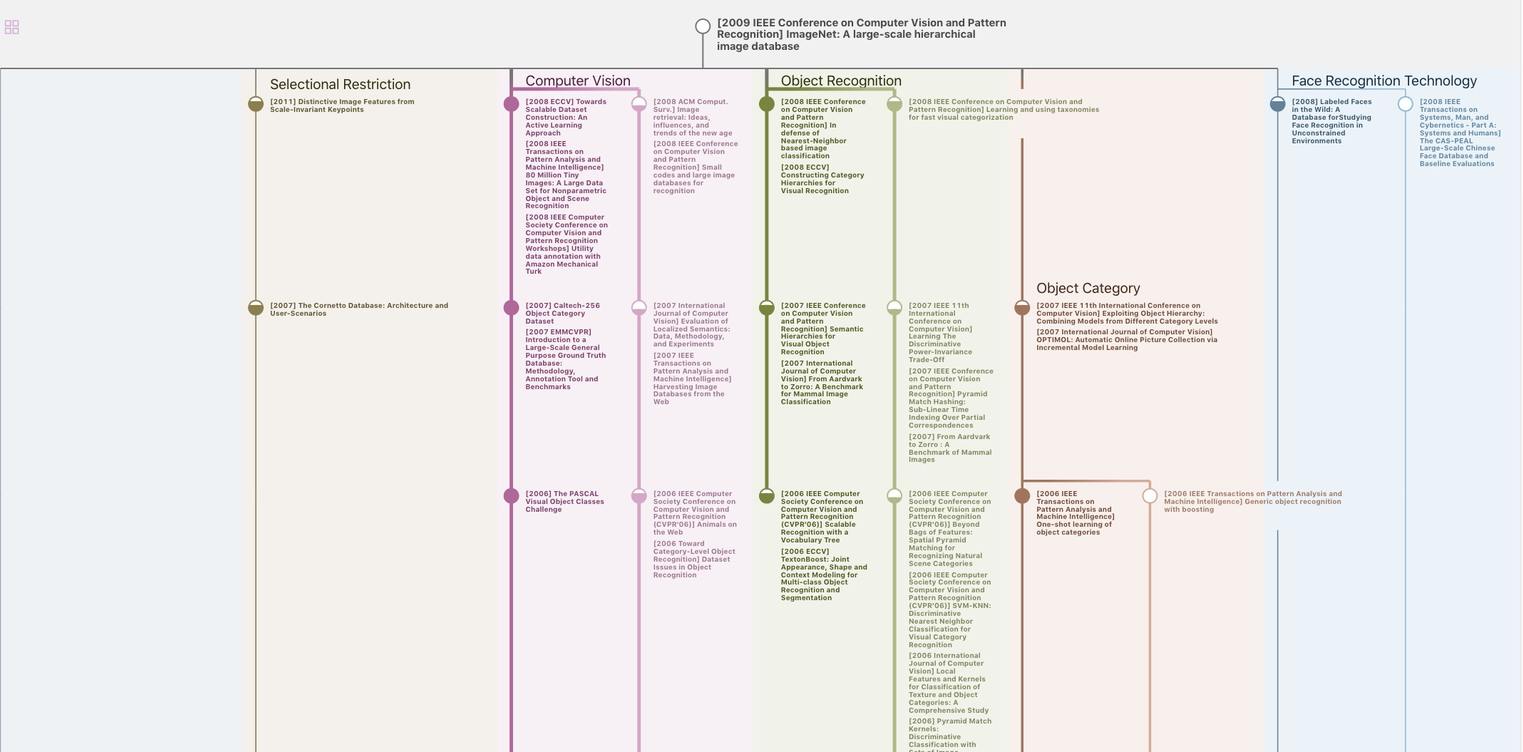
生成溯源树,研究论文发展脉络
Chat Paper
正在生成论文摘要