Geometric and Textural Augmentation for Domain Gap Reduction
IEEE Conference on Computer Vision and Pattern Recognition(2022)
摘要
Research has shown that convolutional neural networks for object recognition are vulnerable to changes in depiction because learning is biased towards the low-level statistics of texture patches. Recent works concentrate on improving robustness by applying style transfer to training examples to mitigate against over-fitting to one depiction style. These new approaches improve performance, but they ignore the geometric variations in object shape that real art exhibits: artists deform and warp objects for artistic effect. Motivated by this observation, we propose a method to reduce bias by jointly increasing the texture and geometry diversities of the training data. In effect, we extend the visual object class to include examples with shape changes that artists use. Specifically, we learn the distribution of warps that cover each given object class. Together with augmenting textures based on a broad distribution of styles, we show by experiments that our method improves performance on several cross-domain benchmarks.
更多查看译文
关键词
Recognition: detection,categorization,retrieval, Machine learning
AI 理解论文
溯源树
样例
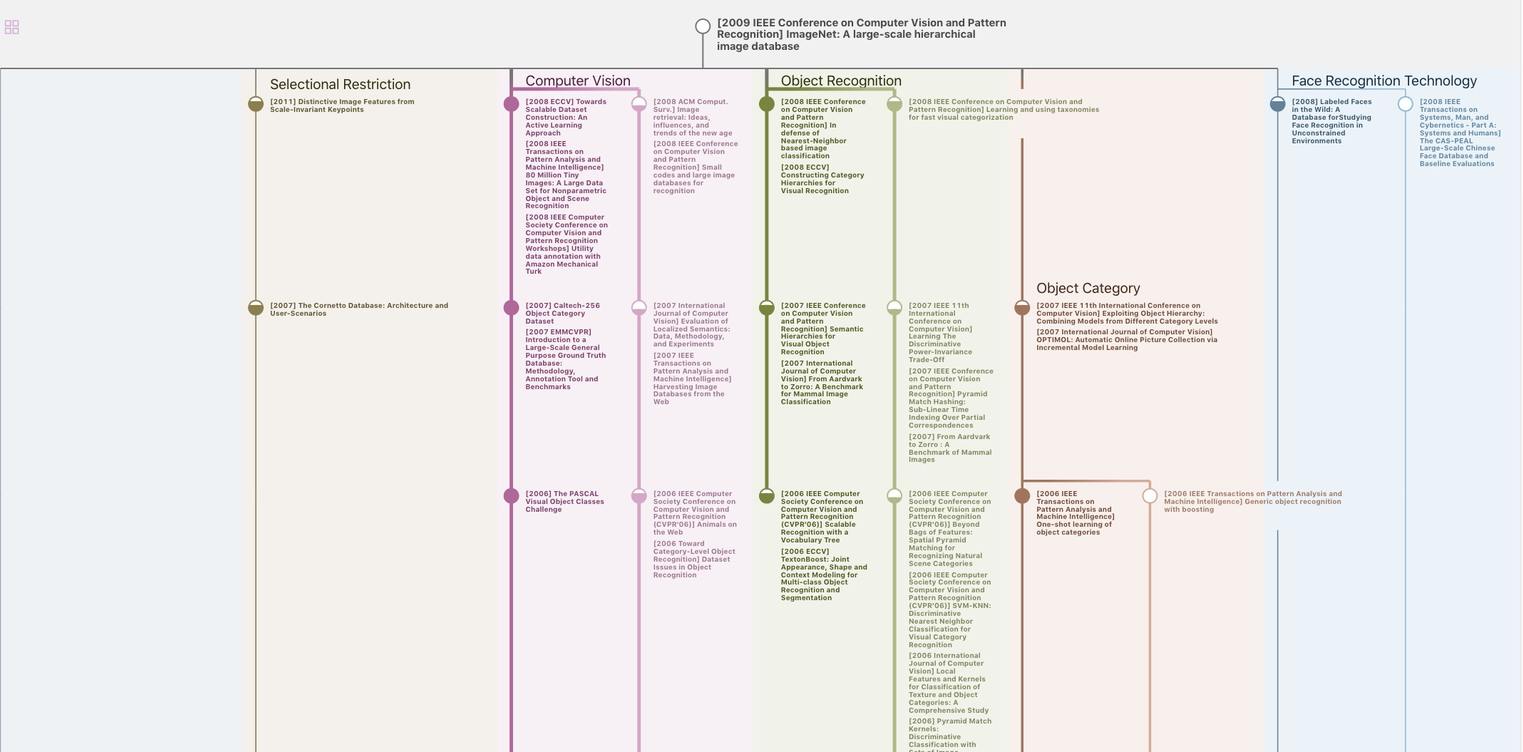
生成溯源树,研究论文发展脉络
Chat Paper
正在生成论文摘要