Balanced and Hierarchical Relation Learning for One-shot Object Detection
IEEE Conference on Computer Vision and Pattern Recognition(2022)
摘要
Instance-level feature matching is significantly important to the success of modern one-shot object detectors. Re-cently, the methods based on the metric-learning paradigm have achieved an impressive process. Most of these works only measure the relations between query and target objects on a single level, resulting in suboptimal performance overall. In this paper, we introduce the balanced and hierarchical learning for our detector. The contributions are two-fold: firstly, a novel Instance-level Hierarchical Relation (IHR) module is proposed to encode the contrastive-level, salient-level, and attention-level relations simultane-ously to enhance the query-relevant similarity representation. Secondly, we notice that the batch training of the IHR module is substantially hindered by the positive-negative sample imbalance in the one-shot scenario. We then in-troduce a simple but effective Ratio-Preserving Loss (RPL) to protect the learning of rare positive samples and sup-press the effects of negative samples. Our loss can adjust the weight for each sample adaptively, ensuring the desired positive-negative ratio consistency and boosting query-related IHR learning. Extensive experiments show that our method outperforms the state-of-the-art method by 1.6% and 1.3% on PASCAL VOC and MS COCO datasets for unseen classes, respectively. The code will be available at https://github.com/hero-y/BHRL.
更多查看译文
关键词
Recognition: detection,categorization,retrieval, Transfer/low-shot/long-tail learning
AI 理解论文
溯源树
样例
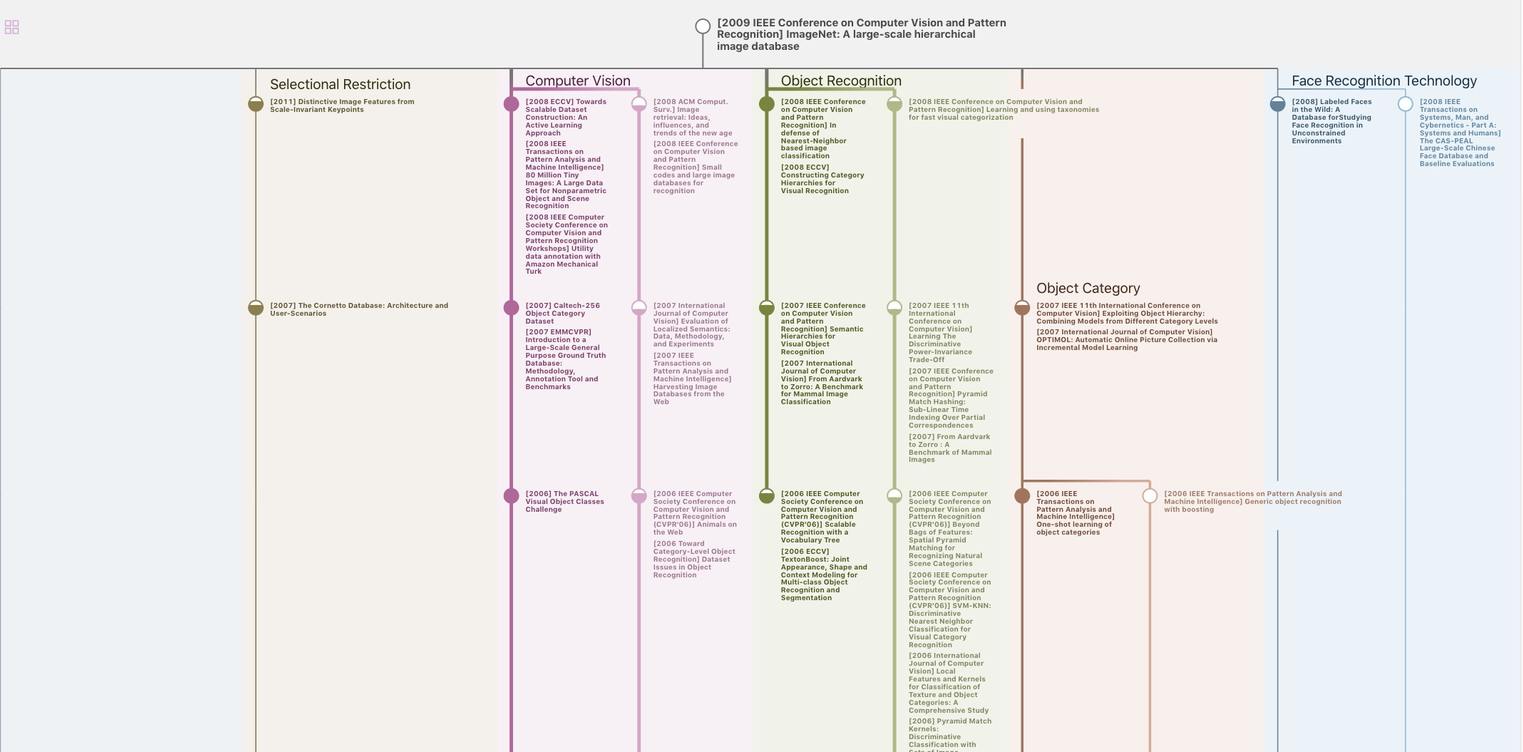
生成溯源树,研究论文发展脉络
Chat Paper
正在生成论文摘要