Approximate Data Deletion in Generative Models
arxiv(2022)
摘要
Users have the right to have their data deleted by third-party learned systems, as codified by recent legislation such as the General Data Protection Regulation (GDPR) and the California Consumer Privacy Act (CCPA). Such data deletion can be accomplished by full re-training, but this incurs a high computational cost for modern machine learning models. To avoid this cost, many approximate data deletion methods have been developed for supervised learning. Unsupervised learning, in contrast, remains largely an open problem when it comes to (approximate or exact) efficient data deletion. In this paper, we propose a density-ratio-based framework for generative models. Using this framework, we introduce a fast method for approximate data deletion and a statistical test for estimating whether or not training points have been deleted. We provide theoretical guarantees under various learner assumptions and empirically demonstrate our methods across a variety of generative methods.
更多查看译文
关键词
generative models,deletion
AI 理解论文
溯源树
样例
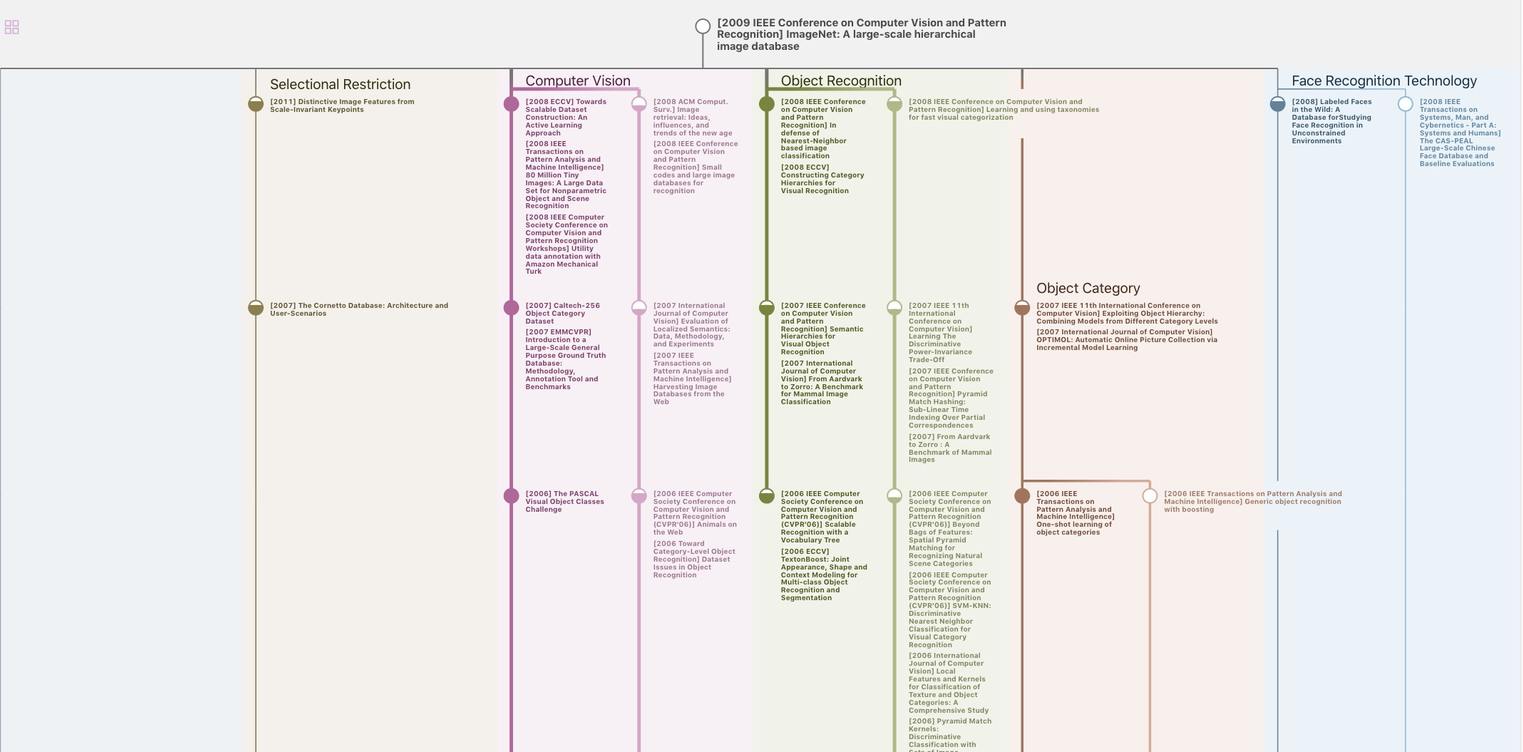
生成溯源树,研究论文发展脉络
Chat Paper
正在生成论文摘要