Intrinsic Anomaly Detection for Multi-Variate Time Series
arxiv(2022)
摘要
We introduce a novel, practically relevant variation of the anomaly detection problem in multi-variate time series: intrinsic anomaly detection. It appears in diverse practical scenarios ranging from DevOps to IoT, where we want to recognize failures of a system that operates under the influence of a surrounding environment. Intrinsic anomalies are changes in the functional dependency structure between time series that represent an environment and time series that represent the internal state of a system that is placed in said environment. We formalize this problem, provide under-studied public and new purpose-built data sets for it, and present methods that handle intrinsic anomaly detection. These address the short-coming of existing anomaly detection methods that cannot differentiate between expected changes in the system's state and unexpected ones, i.e., changes in the system that deviate from the environment's influence. Our most promising approach is fully unsupervised and combines adversarial learning and time series representation learning, thereby addressing problems such as label sparsity and subjectivity, while allowing to navigate and improve notoriously problematic anomaly detection data sets.
更多查看译文
关键词
anomaly,detection,multi-variate
AI 理解论文
溯源树
样例
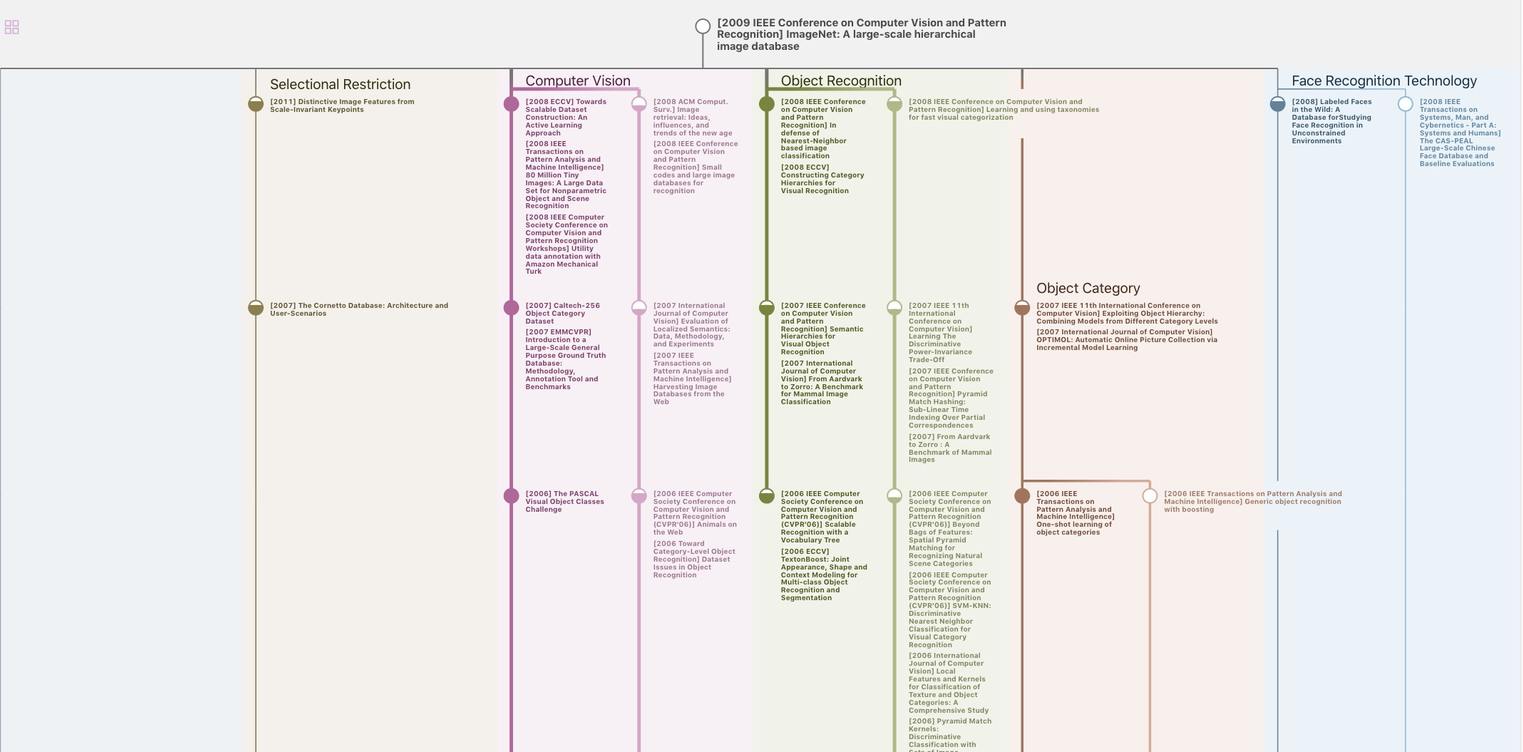
生成溯源树,研究论文发展脉络
Chat Paper
正在生成论文摘要