Joint Generator-Ranker Learning for Natural Language Generation
conf_acl(2022)
摘要
Generate-then-rank is a widely used mechanism for text generation, where a generator produces multiple candidates and a ranker chooses the best one. However, existing methods usually train the generator and the ranker separately, which causes a lack of mutual feedback and a misalignment of their objectives. This results in suboptimal generation quality. To address this issue, we propose JGR, a novel joint training algorithm that integrates the generator and the ranker in a single framework. JGR optimizes the generator with a hybrid objective that combines data likelihood and ranker reward, and trains the ranker with a contrastive loss that compares the generator outputs. By alternately updating the generator and the ranker, JGR can effectively harmonize their learning and enhance their quality jointly. We evaluate JGR on various text generation tasks and demonstrate that it surpasses existing methods on four public datasets across three common generation scenarios. Our code, data, and models are available at https://github.com/microsoft/AdvNLG.
更多查看译文
关键词
generation,learning,language,generator-ranker
AI 理解论文
溯源树
样例
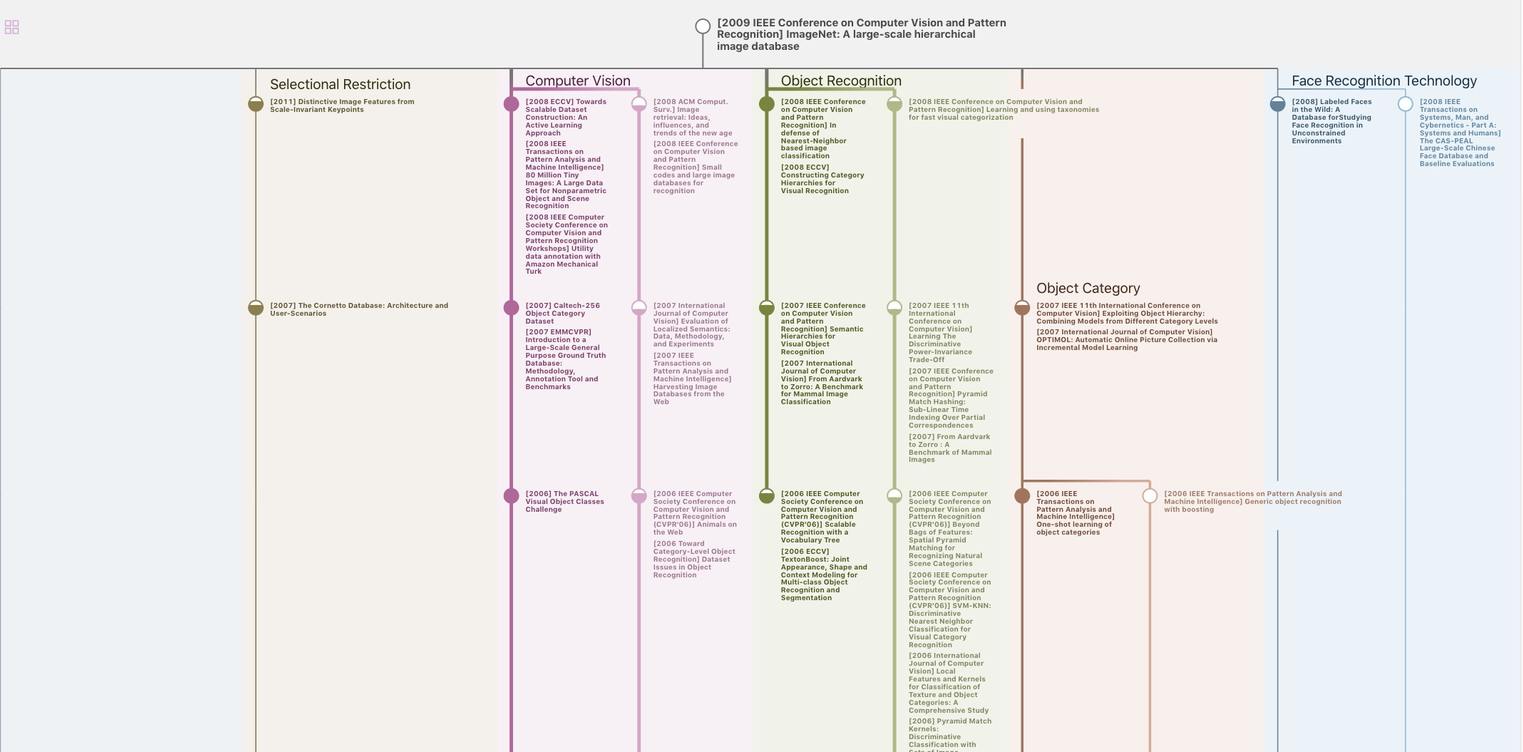
生成溯源树,研究论文发展脉络
Chat Paper
正在生成论文摘要