Rethinking Model-Based Gaze Estimation
PROCEEDINGS OF THE ACM ON COMPUTER GRAPHICS AND INTERACTIVE TECHNIQUES(2022)
摘要
Over the past several years, a number of data-driven gaze tracking algorithms have been proposed, which have been shown to outperform classic model-based methods in terms of gaze direction accuracy. These algorithms leverage the recent development of sophisticated CNN architectures, as well as the availability of large gaze datasets captured under various conditions. One shortcoming of black-box, end-to-end methods, though, is that any unexpected behaviors are difficult to explain. In addition, there is always the risk that a system trained with a certain dataset may not perform well when tested on data from a different source (the "domain gap" problem.) In this work, we propose a novel method to embed eye geometry information in an end-to-end gaze estimation network by means of a "geometric layer". Our experimental results show that our system outperforms other state-of-the-art methods in cross-dataset evaluation, while producing competitive performance over within dataset tests. In addition, the proposed system is able to extrapolate gaze angles outside the range of those considered in the training data.
更多查看译文
关键词
Gaze Estimation, Neural Networks, Eye Geometry
AI 理解论文
溯源树
样例
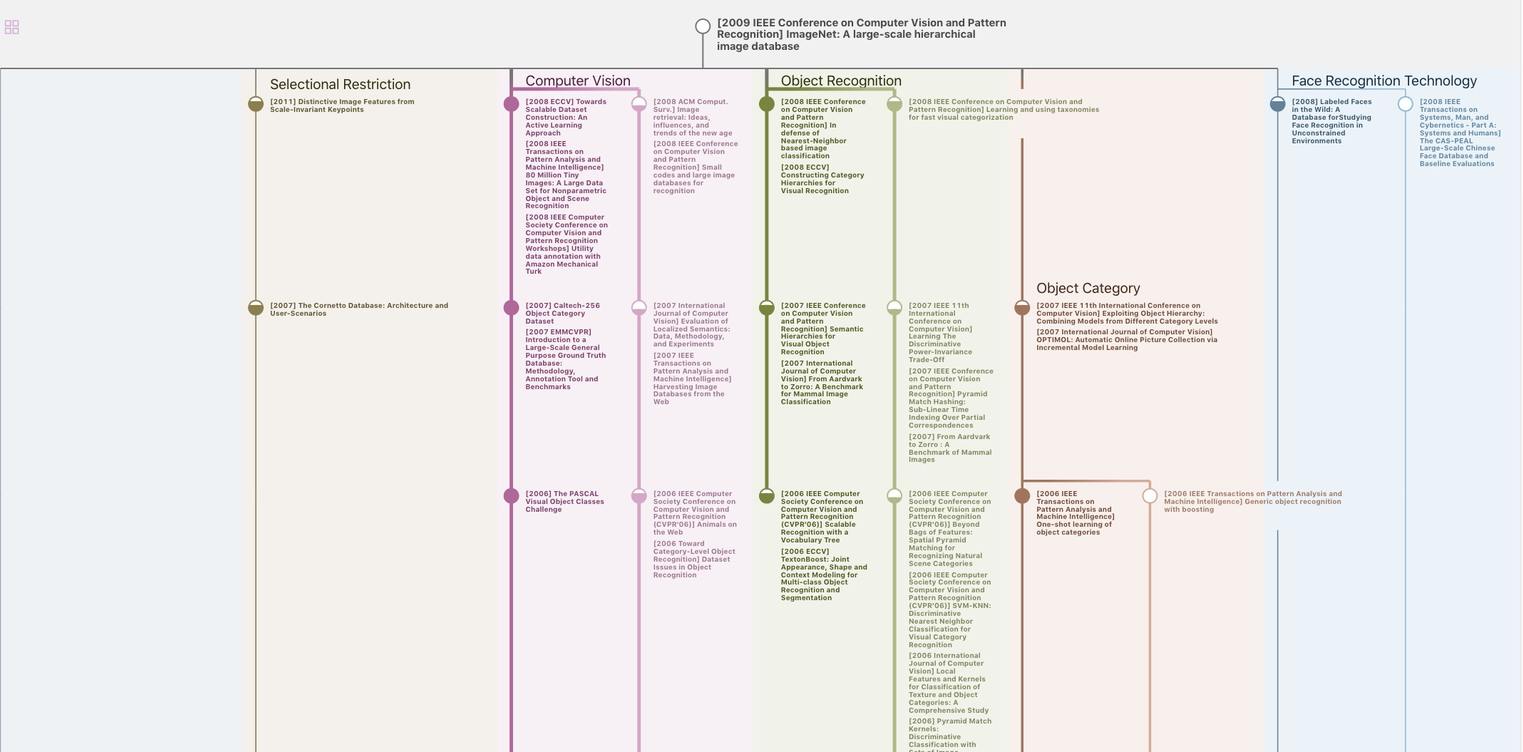
生成溯源树,研究论文发展脉络
Chat Paper
正在生成论文摘要