Tackling Asymmetric and Circular Sequential Social Dilemmas with Reinforcement Learning and Graph-based Tit-for-Tat
arxiv(2022)
摘要
In many societal and industrial interactions, participants generally prefer their pure self-interest at the expense of the global welfare. Known as social dilemmas, this category of non-cooperative games offers situations where multiple actors should all cooperate to achieve the best outcome but greed and fear lead to a worst self-interested issue. Recently, the emergence of Deep Reinforcement Learning (RL) has generated revived interest in social dilemmas with the introduction of Sequential Social Dilemma (SSD). Cooperative agents mixing RL policies and Tit-for-tat (TFT) strategies have successfully addressed some non-optimal Nash equilibrium issues. However, this kind of paradigm requires symmetrical and direct cooperation between actors, conditions that are not met when mutual cooperation become asymmetric and is possible only with at least a third actor in a circular way. To tackle this issue, this paper extends SSD with Circular Sequential Social Dilemma (CSSD), a new kind of Markov games that better generalizes the diversity of cooperation between agents. Secondly, to address such circular and asymmetric cooperation, we propose a candidate solution based on RL policies and a graph-based TFT. We conducted some experiments on a simple multi-player grid world which offers adaptable cooperation structures. Our work confirmed that our graph-based approach is beneficial to address circular situations by encouraging self-interested agents to reach mutual cooperation.
更多查看译文
关键词
circular sequential social dilemmas,reinforcement learning,graph-based,tit-for-tat
AI 理解论文
溯源树
样例
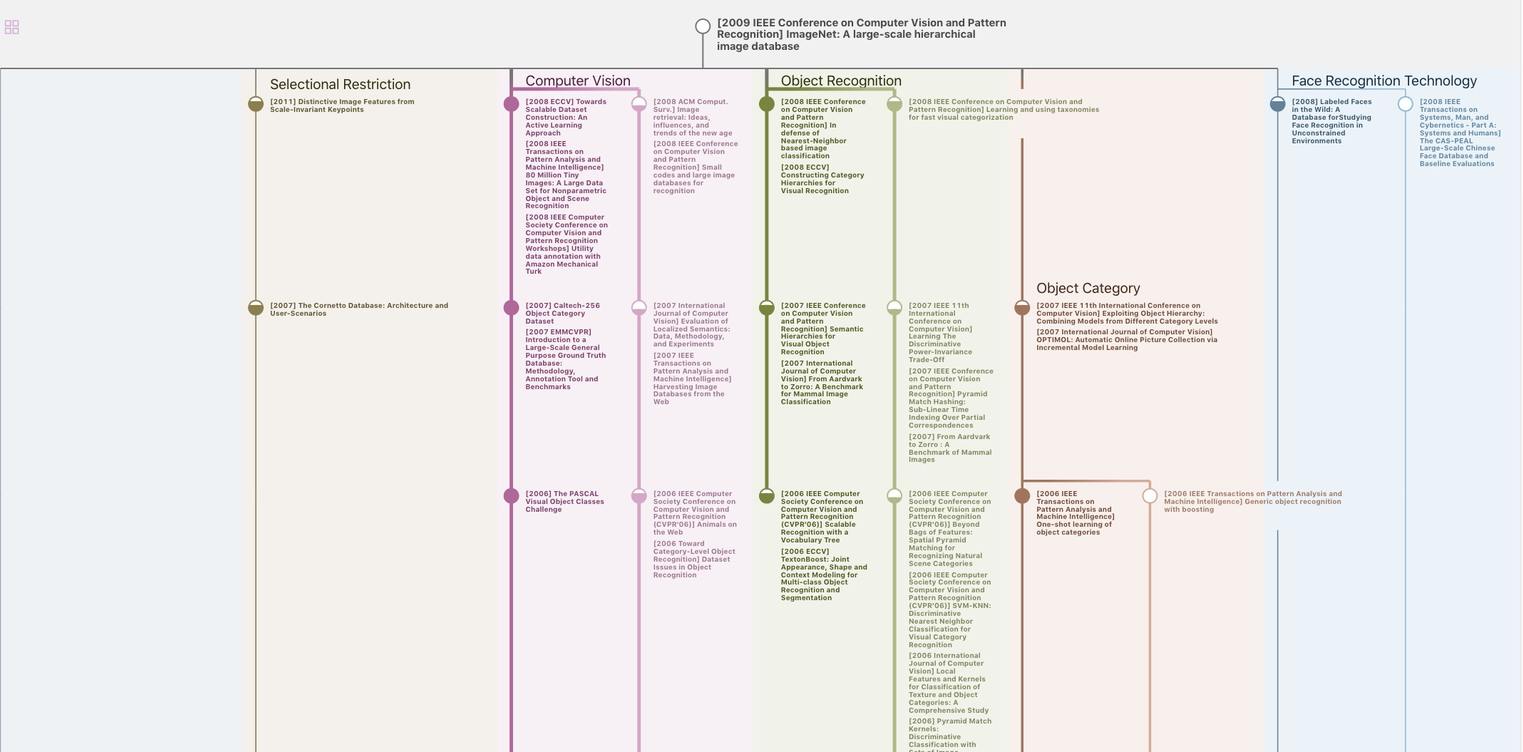
生成溯源树,研究论文发展脉络
Chat Paper
正在生成论文摘要