Render unto Numerics: Orthogonal Polynomial Neural Operator for PDEs with Non-periodic Boundary Conditions
arxiv(2023)
摘要
By learning the mappings between infinite function spaces using carefully designed neural networks, the operator learning methodology has exhibited significantly more efficiency than traditional methods in solving complex problems such as differential equations, but faces concerns about their accuracy and reliability. To overcomes these limitations, combined with the structures of the spectral numerical method, a general neural architecture named spectral operator learning (SOL) is introduced, and one variant called the orthogonal polynomial neural operator (OPNO), developed for PDEs with Dirichlet, Neumann and Robin boundary conditions (BCs), is proposed later. The strict BC satisfaction properties and the universal approximation capacity of the OPNO are theoretically proven. A variety of numerical experiments with physical backgrounds show that the OPNO outperforms other existing deep learning methodologies, as well as the traditional 2nd-order finite difference method (FDM) with a considerably fine mesh (with the relative errors reaching the order of 1e-6), and is up to almost 5 magnitudes faster than the traditional method.
更多查看译文
关键词
orthogonal polynomial neural operator,pdes
AI 理解论文
溯源树
样例
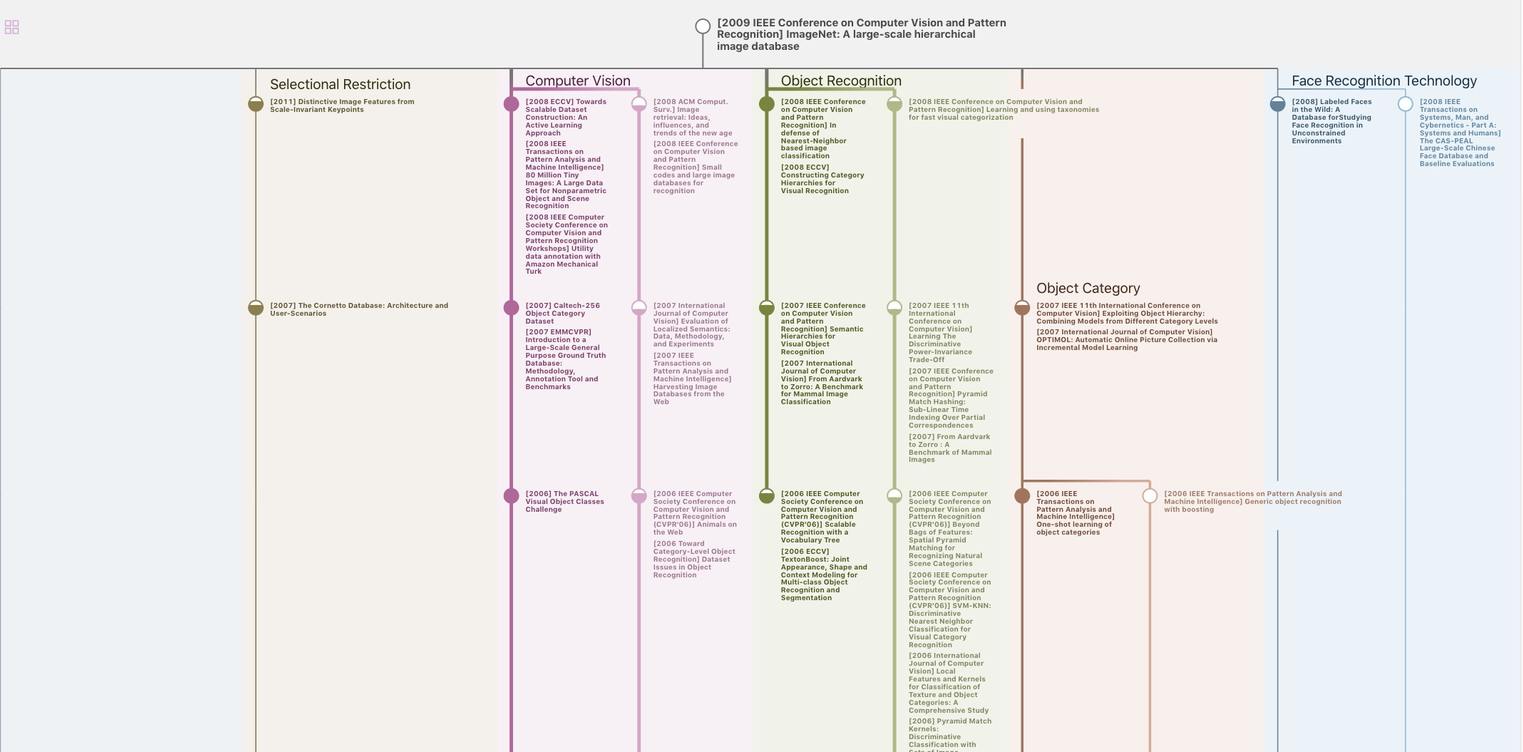
生成溯源树,研究论文发展脉络
Chat Paper
正在生成论文摘要