Development of a Slow Loris Computer Vision Detection Model
ANIMALS(2022)
摘要
Simple Summary Slow lorises are nocturnal primates native to south-east Asia. All the species of slow loris have been listed in Appendix I of the Convention on International Trade in Endangered Species of Wild Fauna and Flora (CITES). It is difficult to artificially detect the slow loris due to its nocturnal habit and venomous bite. This article investigates the feasibility of computer vision for slow loris detection and proposes an improved YOLOv5 algorithm that contributes to formulating an available model for behavior recognition of this endangered taxon. The slow loris (Genus Nycticebus) is a group of small, nocturnal and venomous primates with a distinctive locomotion mode. The detection of slow loris plays an important role in the subsequent individual identification and behavioral recognition and thus contributes to formulating targeted conservation strategies, particularly in reintroduction and post-release monitoring. However, fewer studies have been conducted on efficient and accurate detection methods of this endangered taxa. The traditional methods to detect the slow loris involve long-term observation or watching surveillance video repeatedly, which would involve manpower and be time consuming. Because humans cannot maintain a high degree of attention for a long time, they are also prone to making missed detections or false detections. Due to these observational challenges, using computer vision to detect slow loris presence and activity is desirable. This article establishes a novel target detection dataset based on monitoring videos of captive Bengal slow loris (N. bengalensis) from the wildlife rescue centers in Xishuangbanna and Pu'er, Yunnan, China. The dataset is used to test two improvement schemes based on the YOLOv5 network: (1) YOLOv5-CBAM + TC, the attention mechanism and deconvolution are introduced; (2) YOLOv5-SD, the small object detection layer is added. The results demonstrate that the YOLOv5-CBAM + TC effectively improves the detection effect. At the cost of increasing the model size by 0.6 MB, the precision rate, the recall rate and the mean average precision (mAP) are increased by 2.9%, 3.7% and 3.5%, respectively. The YOLOv5-CBAM + TC model can be used as an effective method to detect individual slow loris in a captive environment, which helps to realize slow loris face and posture recognition based on computer vision.
更多查看译文
关键词
Nycticebus, computer vision, object detection, animal protection, behavior recognition
AI 理解论文
溯源树
样例
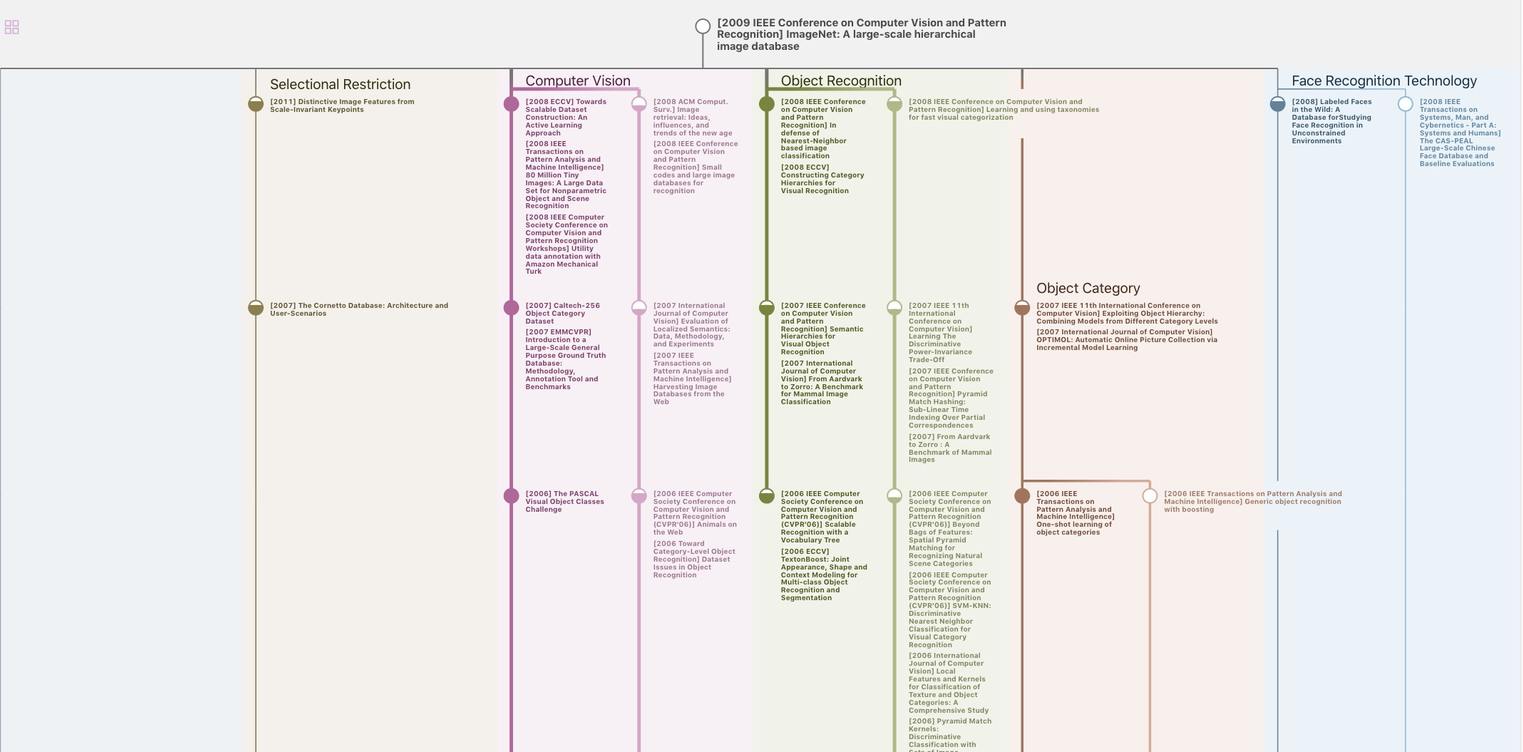
生成溯源树,研究论文发展脉络
Chat Paper
正在生成论文摘要