Inference on the best policies with many covariates
JOURNAL OF ECONOMETRICS(2024)
摘要
Understanding the impact of the most effective policies or treatments on a response variable of interest is desirable in many empirical works in economics, statistics and other disciplines. Due to the widespread winner's curse phenomenon, conventional statistical inference assuming that the top policies are chosen independent of the random sample may lead to overly optimistic evaluations of the best policies. In recent years, given the increased availability of large datasets, such an issue can be further complicated when researchers include many covariates to estimate the policy or treatment effects in an attempt to control for potential confounders. In this manuscript, to simultaneously address the above-mentioned issues, we propose a resampling-based procedure that not only lifts the winner's curse in evaluating the best policies observed in a random sample, but also is robust to the presence of many covariates. The proposed inference procedure yields accurate point estimates and valid frequentist confidence intervals that achieve the exact nominal level as the sample size goes to infinity for multiple best policy effect sizes. We illustrate the finite-sample performance of our approach through Monte Carlo experiments and two empirical studies, evaluating the most effective policies in charitable giving and the most beneficial group of workers in the National Supported Work program. (c) 2023 Elsevier B.V. All rights reserved.
更多查看译文
关键词
Winner's curse,High dimensional data,Linear regression,Order statistics
AI 理解论文
溯源树
样例
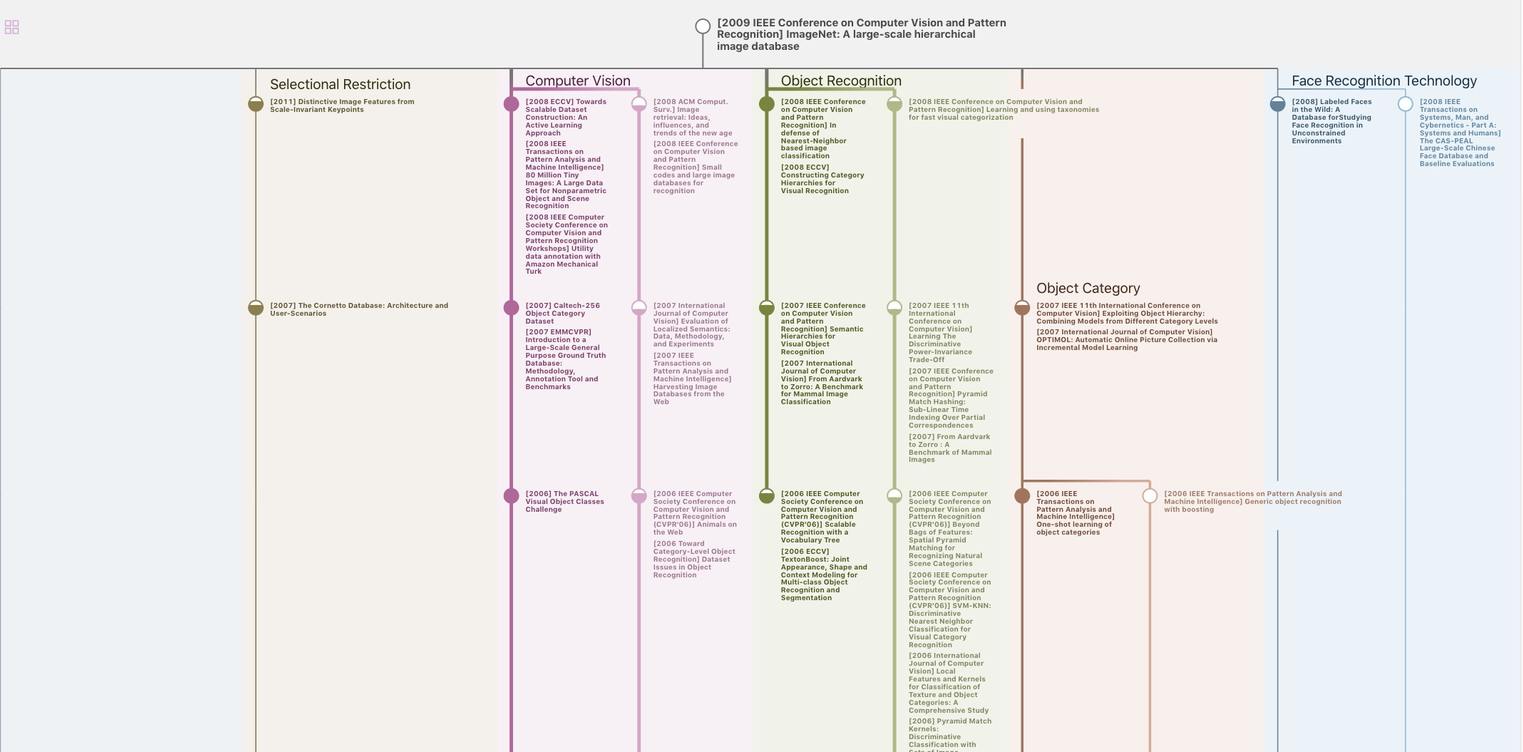
生成溯源树,研究论文发展脉络
Chat Paper
正在生成论文摘要