Beyond Uniform Lipschitz Condition in Differentially Private Optimization
arxiv(2022)
摘要
Most prior convergence results on differentially private stochastic gradient descent (DP-SGD) are derived under the simplistic assumption of uniform Lipschitzness, i.e., the per-sample gradients are uniformly bounded. This assumption is unrealistic in many problems, e.g., linear regression with Gaussian data. We relax uniform Lipschitzness by instead assuming that the per-sample gradients have \textit{sample-dependent} upper bounds, i.e., per-sample Lipschitz constants, which themselves may be unbounded. We derive new convergence results for DP-SGD on both convex and nonconvex functions when the per-sample Lipschitz constants have bounded moments. Furthermore, we provide principled guidance on choosing the clip norm in DP-SGD for convex settings satisfying our relaxed version of Lipschitzness, without making distributional assumptions on the Lipschitz constants. We verify the effectiveness of our recommendation via experiments on benchmarking datasets.
更多查看译文
关键词
uniform lipschitz condition,differentially,optimization
AI 理解论文
溯源树
样例
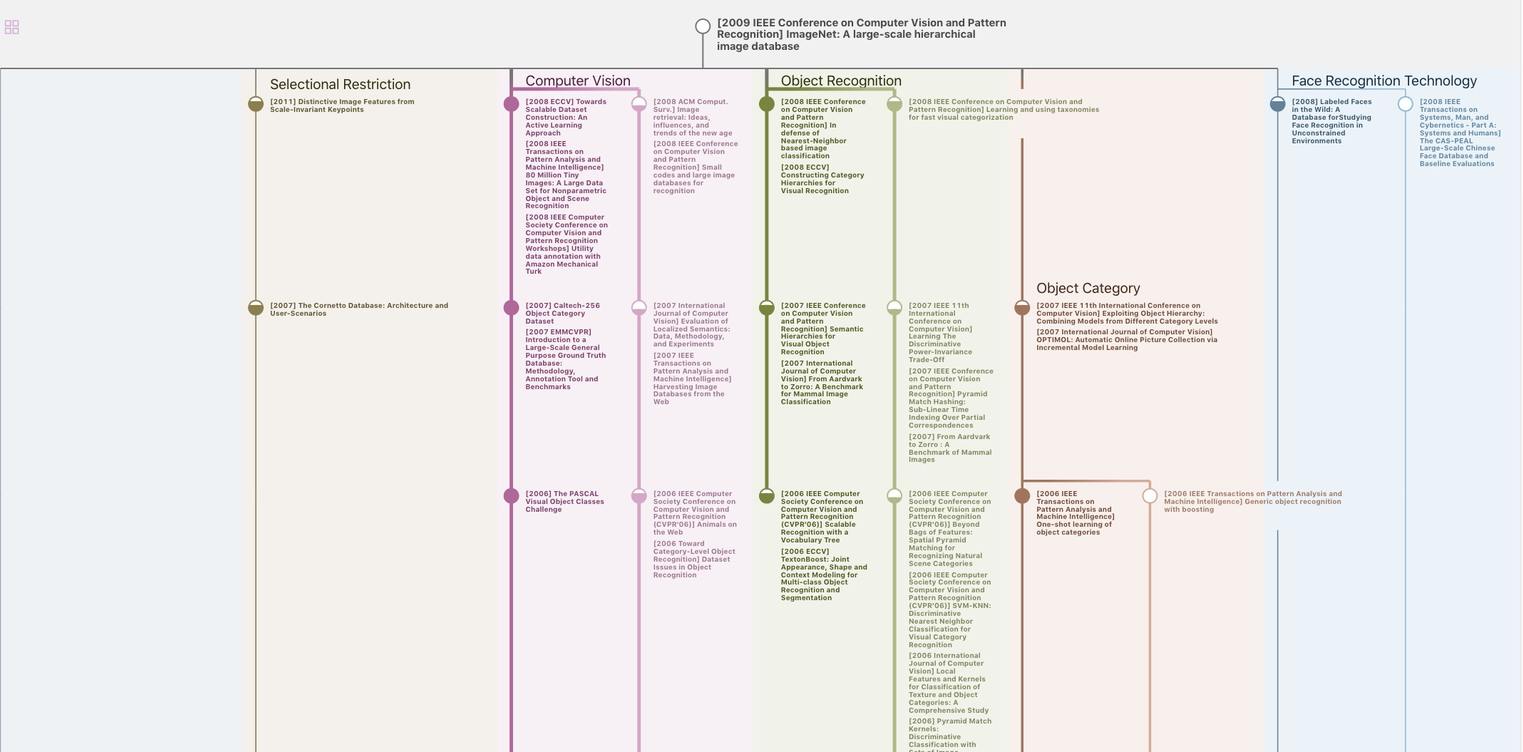
生成溯源树,研究论文发展脉络
Chat Paper
正在生成论文摘要