Enabling Multi-scale Features and Double-Attention for Single-image 3D Urban Reconstruction
2021 China Automation Congress (CAC)(2021)
摘要
3D reconstruction from a single RGB image for urban scenes has been a foundation for safety-critical applications such as autonomous driving and city planning. It is essential to develop 3D reconstruction models that are accurate for these applications. The most recent single-image 3D reconstruction models leverage representative neural networks to address the problem and have achieved promising results. However, these approaches cannot correctly calculate the segmentation map and reconstruct depth for ambiguous scene regions and when scene information gets lost going through down-sampling layers. To solve this problem, we propose EMERALD, an end-to-end single-image 3D reconstruction framework, in which we empower the fully convolutional networks (FCN) with multi-scale contextual features and a novel double-attention mechanism to advance its discrimination capability on ambiguous scene regions and loss of scene details. The evaluation results on two leading urbanscene data sets, Cityscapes (Segmentation Covering (SC)1 of 61.2%) and SYNTHIA (SC of 81.9%), demonstrate the optimized network architecture’s effectiveness.
更多查看译文
关键词
multiscale features,single RGB image,urban scenes,safety-critical applications,autonomous driving,city planning,ambiguous scene regions,scene information,end-to-end single-image 3D reconstruction framework,multiscale contextual features,double-attention mechanism,scene details,single-image 3D reconstruction models,representative neural networks,fully convolutional networks
AI 理解论文
溯源树
样例
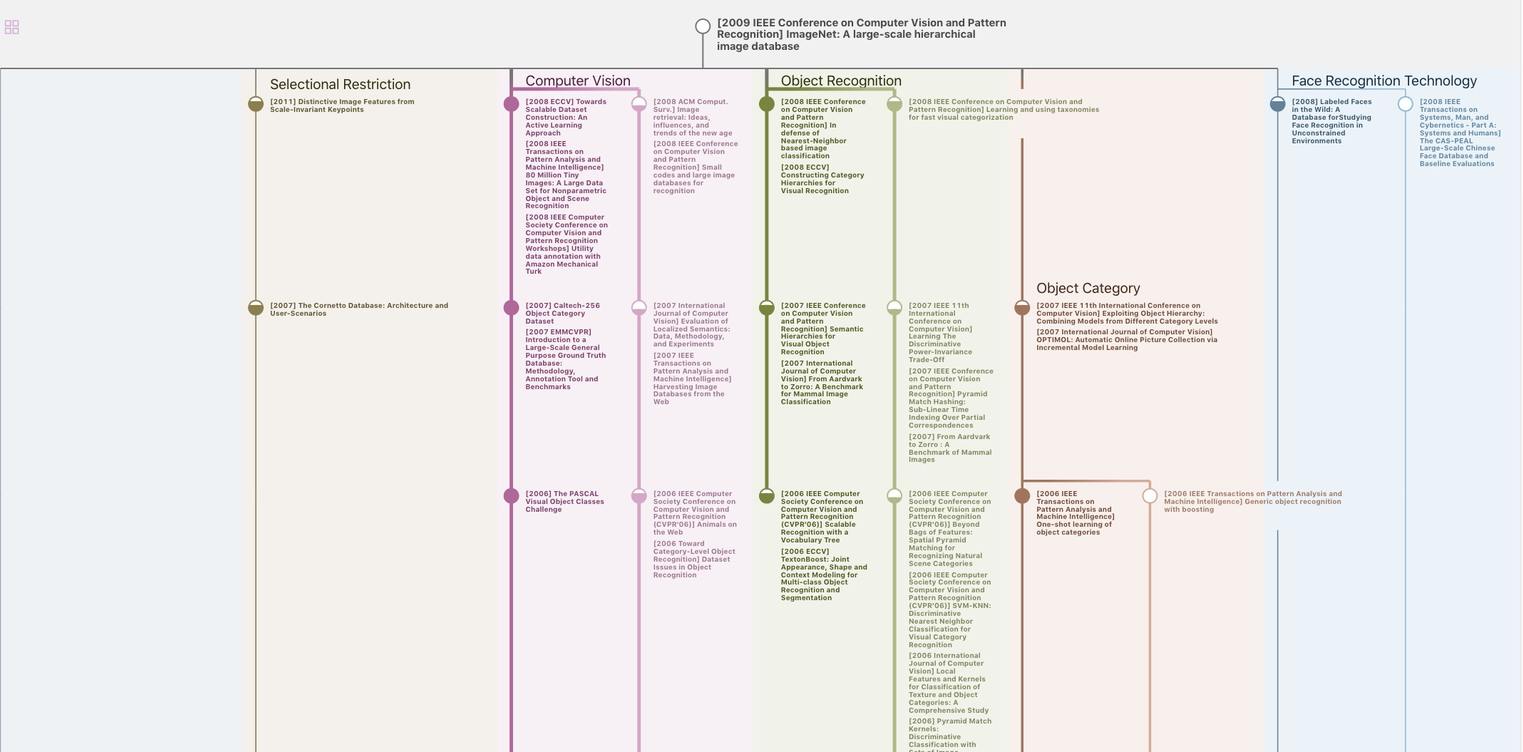
生成溯源树,研究论文发展脉络
Chat Paper
正在生成论文摘要