Category Alignment Adversarial Learning for Cross-Modal Retrieval
IEEE Transactions on Knowledge and Data Engineering(2023)
摘要
Cross-modal retrieval aims to retrieve one semantically similar media from multiple media types based on queries entered by another type of media. An intuitive idea is to map different media data into a common space and then directly measure content similarity between different types of data. In this paper, we present a novel method, called Category Alignment Adversarial Learning (CAAL) for cross-modal retrieval. It aims to find a common representation space supervised by category information, in which the samples from different modalities can be compared directly. Specifically, CAAL first employs two parallel encoders to generate common representations for image and text features respectively. Furthermore, we employ two parallel GANs with category information to generate fake image and text features which next will be utilized with already generated embedding to reconstruct the common representation. At last, two joint discriminators are utilized to reduce the gap between the mapping of the first stage and the embedding of the second stage. Comprehensive experimental results on four widely-used benchmark datasets demonstrate the superior performance of our proposed method compared with the state-of-the-art approaches.
更多查看译文
关键词
Semantics, Correlation, Media, Adversarial machine learning, Pairwise error probability, Hidden Markov models, Feature extraction, Category, cross-modal, adversarial learning, alignment
AI 理解论文
溯源树
样例
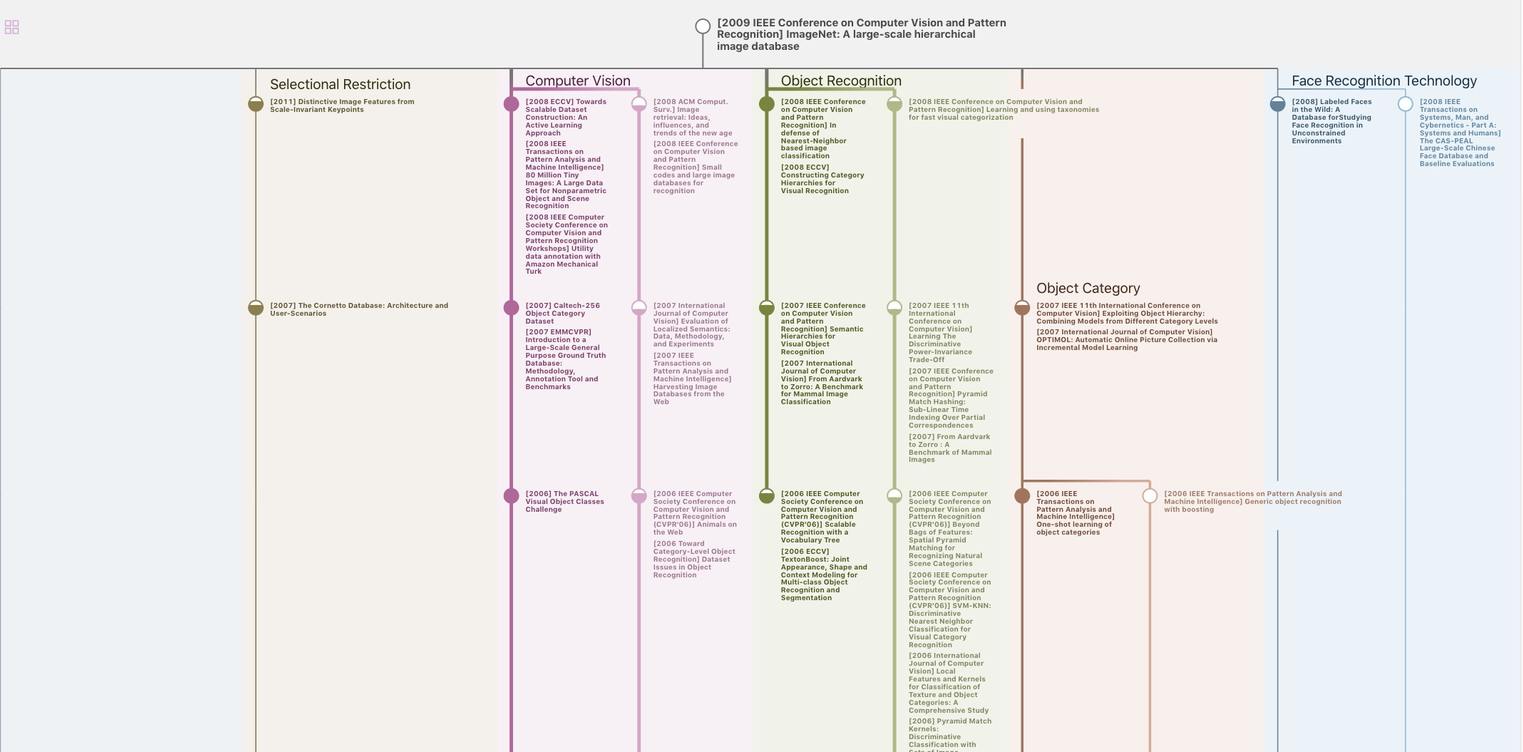
生成溯源树,研究论文发展脉络
Chat Paper
正在生成论文摘要