Nonblind Image Deconvolution via Leveraging Model Uncertainty in An Untrained Deep Neural Network
International Journal of Computer Vision(2022)
摘要
Nonblind image deconvolution (NID) is about restoring the latent image with sharp details from a noisy blurred one using a known blur kernel. This paper presents a dataset-free deep learning approach for NID using untrained deep neural networks (DNNs), which does not require any external training data with ground-truth images. Based on a spatially-adaptive dropout scheme, the proposed approach learns a DNN with model uncertainty from the input blurred image, and the deconvolution result is obtained by aggregating the multiple predictions from the learned dropout DNN. It is shown that the solution approximates a minimum-mean-squared-error estimator in Bayesian inference. In addition, a self-supervised loss function for training is presented to efficiently handle the noise in blurred images. Extensive experiments show that the proposed approach not only performs noticeably better than existing non-learning-based methods and unsupervised learning-based methods, but also performs competitively against recent supervised learning-based methods.
更多查看译文
关键词
Deconvolution, Inverse problems, Unsupervised learning, Untrained neural networks
AI 理解论文
溯源树
样例
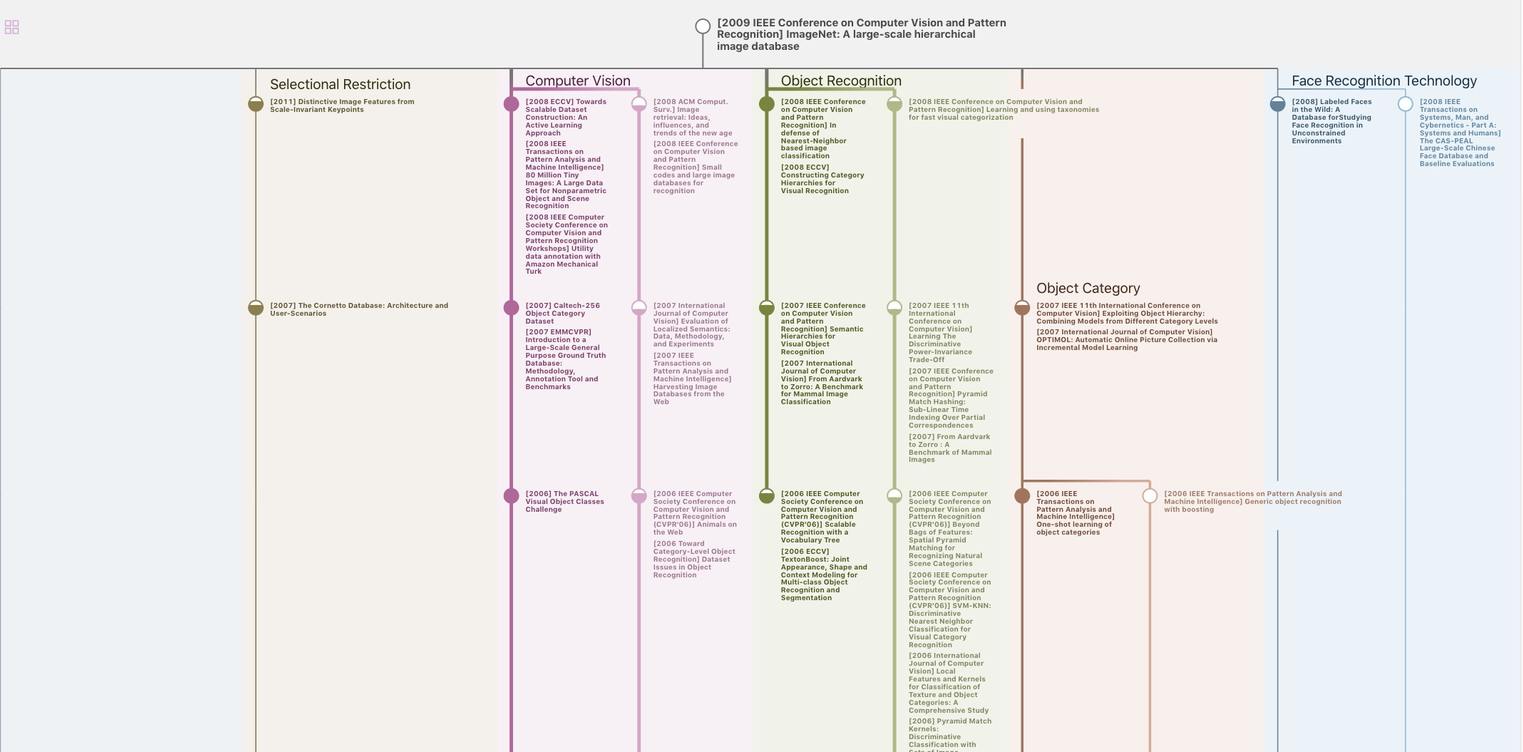
生成溯源树,研究论文发展脉络
Chat Paper
正在生成论文摘要