A More Efficient Deep-learning Processing Unit Architecture with Runtime Configurable Parallelism
2021 China Automation Congress (CAC)(2021)
摘要
Typically, the loop dimension varies greatly between the different layers of a Convolutional Neural Network (CNN). However, the loop dimension’s parallelism degree of most Deep-learning Processing Units (DPUs) is not runtime configurable. It results in low theoretical performance utilization for most high parallelism DPUs. To solve the problem, we propose a new DPU architecture, named Dataflow Driven Multicore Architecture (DDMA). It consists of multiple function cores, and the direction of data flow between them can be configured at runtime by a routing module. This design allows DDMA to improve parallelism degree while maintaining computational efficiency. To verify the property of DDMA, -we designed a Basic-DPU (B-DPU) based on it and obtained the Extended-DPU (E-DPU) by improving the parallelism degree of B-DPU. The experimental results show that the peak performance of B-DPU reaches 512 GOPS, and the computational efficiency reaches 91.9%, -which is 1.69 times that of the peer FPGA implementation under the same test algorithm. The peak performance of E-DPU reaches 1024 GOPS, and the actual performance is 1.96 times that of B-DPU. Meanwhile, its computational efficiency is 90.15%, almost the same as B-DPU.
更多查看译文
关键词
DPU,FPGA,CNN,Configurability,Efficiency
AI 理解论文
溯源树
样例
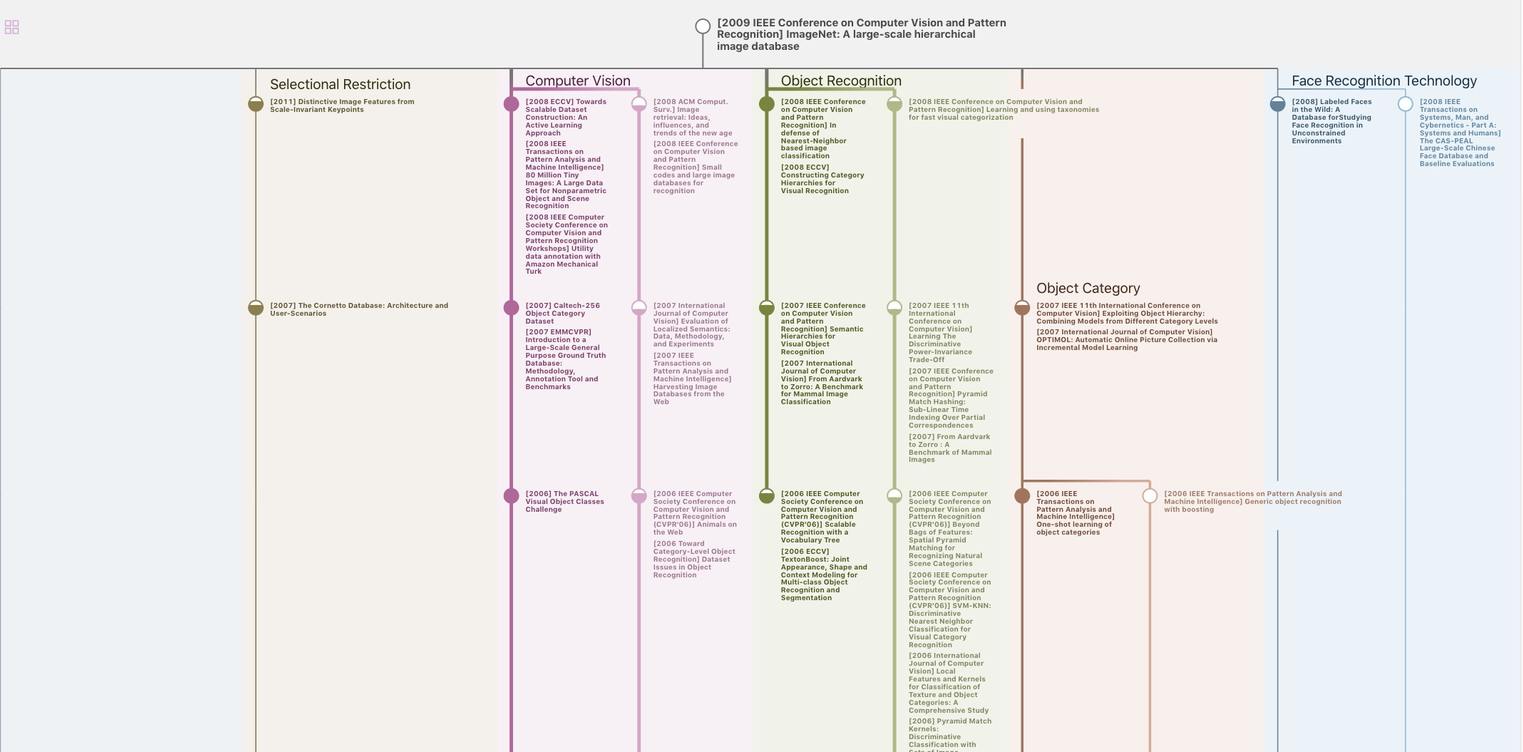
生成溯源树,研究论文发展脉络
Chat Paper
正在生成论文摘要