Medical image segmentation using scalable functional variational Bayesian neural networks with Gaussian processes
Neurocomputing(2022)
摘要
Bayesian neural networks (BNNs) are widely used in medical image segmentation tasks because they provide a probabilistic view of deep learning models by placing a prior distribution over the model parameters, and allow the representation of uncertainties through posterior distribution over the model parameters. However, it is difficult to choose meaningful prior distributions and fit accurate posterior distributions in a high-dimensional weight space, which limit the practical effectiveness of BNNs. In this study, we propose a scalable functional variational BNN with Gaussian processes (GPs) for the purpose of medical image segmentation. Specifically, we regard the prior and variational posterior distributions as GPs, and perform variational inference in function space. We employ a variant of the functional evidence lower bound (fELBO) with a β-weight on the Kullback–Leibler divergence term as the loss objective, and design a content-aware UNet segmentation network, which utilizes content-aware reassembly of features (CARAFE) as an upsampling operator to extract semantic information from input feature maps. The proposed BNN framework enables efficient training and can perform predictive inference with one forward pass. Extensive experiments are conducted on four segmentation datasets; the experimental results demonstrate that the proposed approach improves the segmentation performance metrics and uncertainty estimates compared with several state-of-the-art methods.
更多查看译文
关键词
Bayesian neural networks,Uncertainty estimation,Variational inference,Gaussian process,Medical image segmentation
AI 理解论文
溯源树
样例
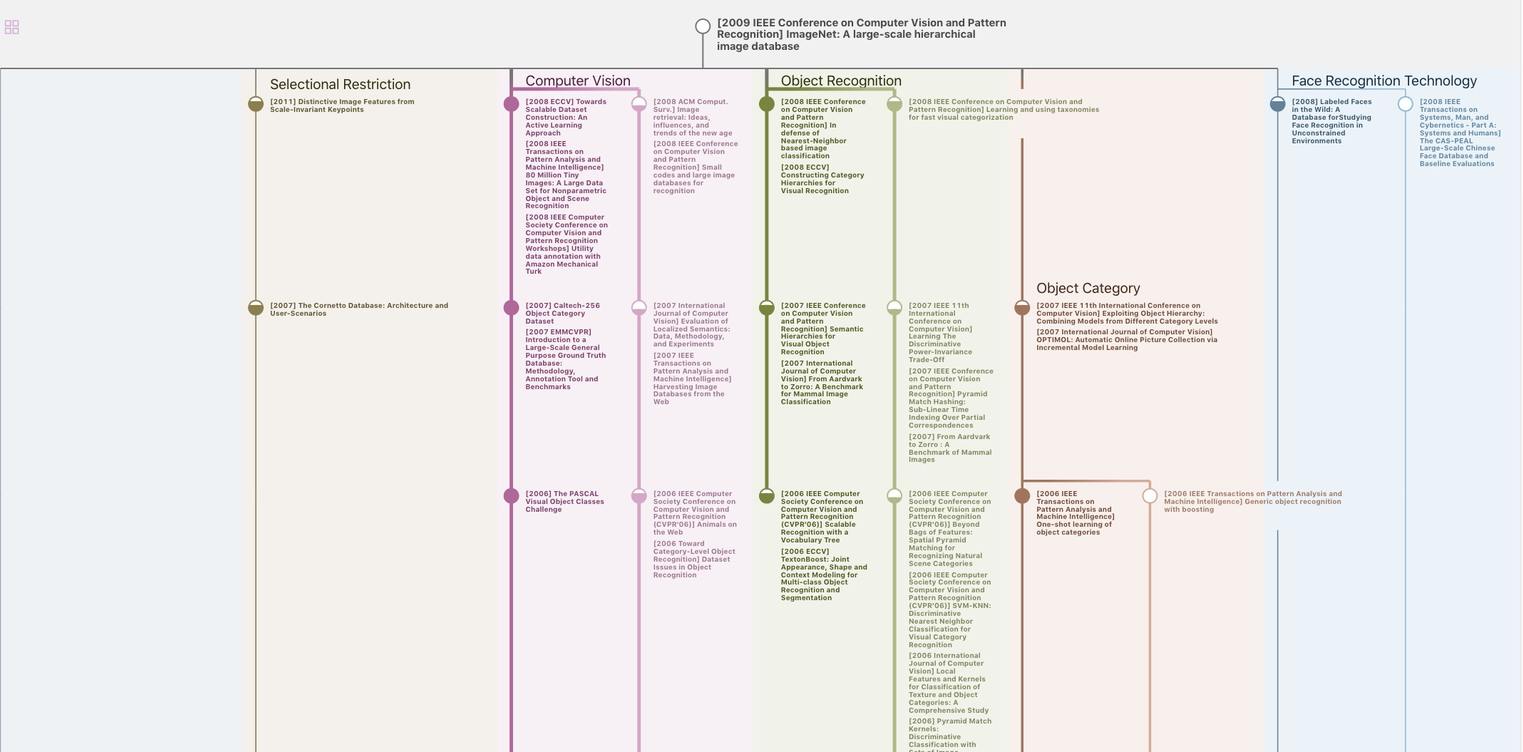
生成溯源树,研究论文发展脉络
Chat Paper
正在生成论文摘要