Sensitivity of Logic Learning Machine for Reliability in Safety-Critical Systems
IEEE Intelligent Systems(2022)
摘要
Nowadays, artificial intelligence (AI) is bursting in many fields, including critical ones, giving rise to reliable AI that means ensuring safety of autonomous decisions. As the false negatives may have a safety impact (e.g., in a mobility scenario, prediction of no collision, but collision in reality), the aim is to push them as close to zero as possible, thus designing “safety regions” in the feature space with statistical zero error. We show here how sensitivity analysis of an explainable AI model drives such statistical assurance. We test and compare the proposed algorithms on two different datasets (physical fatigue and vehicle platooning) and achieve quite different conclusions in terms of achievable performance that strongly depend on the level of noise in the dataset rather than on the algorithms at hand.
更多查看译文
AI 理解论文
溯源树
样例
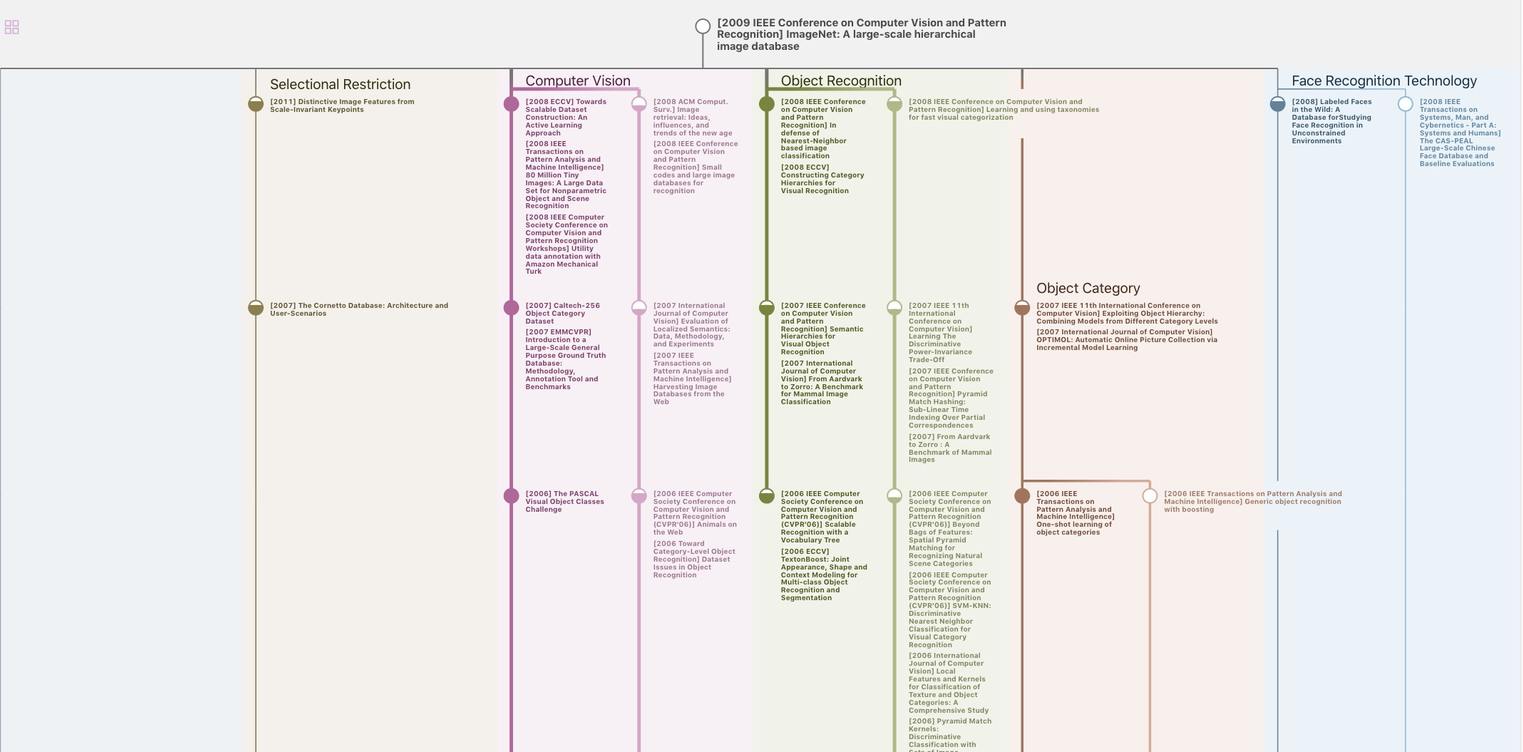
生成溯源树,研究论文发展脉络
Chat Paper
正在生成论文摘要