Crowd counting method via a dynamic-refined density map network
Neurocomputing(2022)
摘要
At present, most existing crowd counting methods use density maps to estimate the number of people, so the quality of density maps is particularly important to the counting results. In practical application, the density map generated by the existing methods is easily affected by the change of head proportion, which cannot truly reflect the size of head in the image and affect the counting accuracy. To effectively overcome the above problems, a crowd counting method via a dynamic-refined density map network is proposed in this paper. This method refines the existing ground-truth density maps by jointly training Counting Net and learnable Refine Net, and counts through Counting Net. Specifically, we design a Refine Net, which adaptively adjusts the relationship between the head sizes at different positions in the dot map through the U-shaped network structure and the regional attention module (RAM), so as to obtain a ground truth that is more in line with the real head size. In addition, we propose a Counting Net based on coprime dilation rate convolution groups to ensure the continuity of information. Extensive experiments on four benchmark datasets (ShanghaiTech, UCF_CC_50, UCF-QNRF and JHU-CROWD++) indicate that our method can achieve state-of-the-art counting performance and high robustness.
更多查看译文
关键词
Crowd counting,Density map,Regional attention module,Coprime dilation rate convolution group
AI 理解论文
溯源树
样例
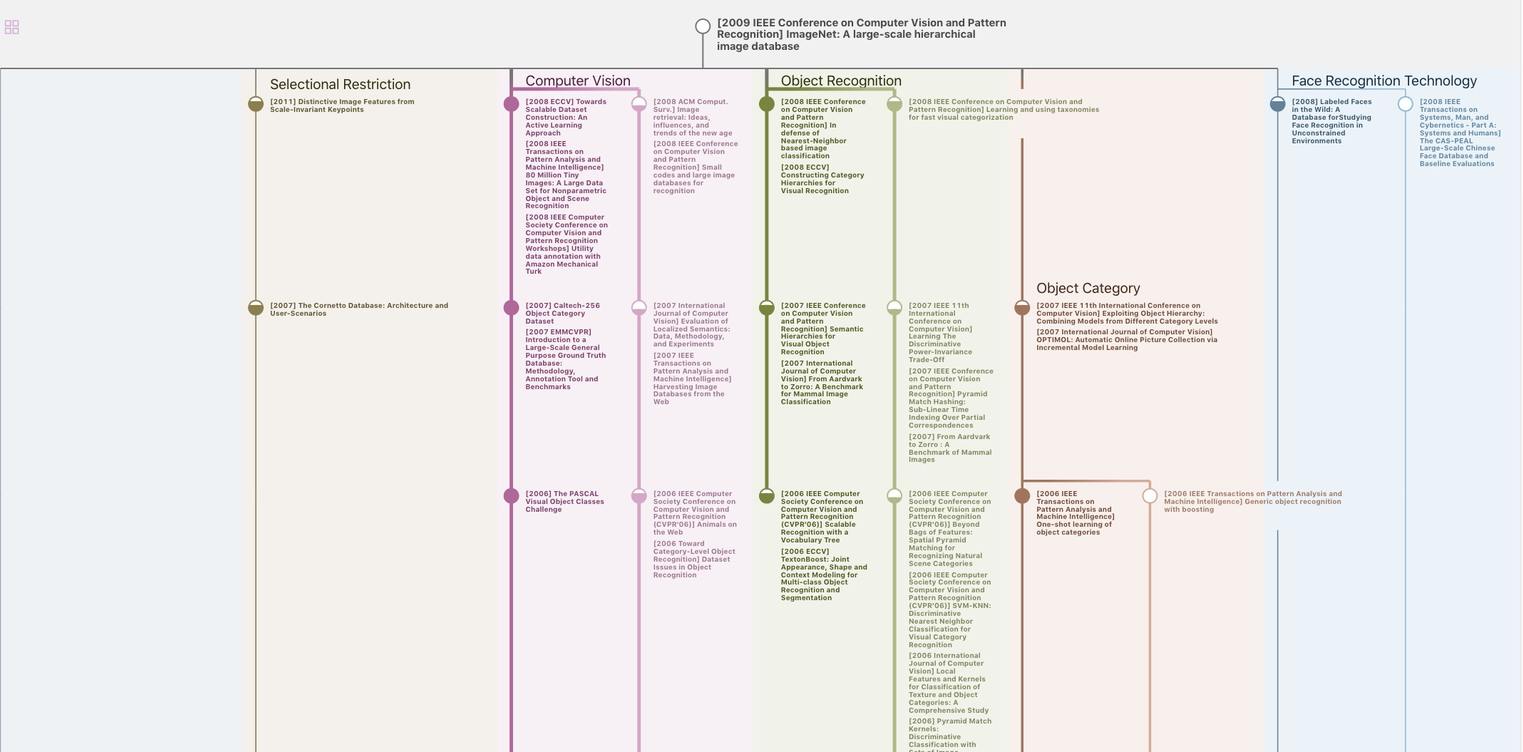
生成溯源树,研究论文发展脉络
Chat Paper
正在生成论文摘要