MF-OMO: An Optimization Formulation of Mean-Field Games
SIAM J. Control. Optim.(2022)
摘要
Recently, theory of mean-field games (MFGs) has experienced an exponential growth. However, existing analytical approaches are by and large restricted to contractive or monotone settings, or with an a priori assumption of the uniqueness of the Nash equilibrium (NE) solution for computational feasibility. This paper proposes a new mathematical framework to analyze discrete-time MFGs with none of these restrictions. The key idea is to reformulate the problem of finding NE solutions in MFGs as solving (equivalently) an optimization problem with bounded variables and simple convex constraints. This is built on the classical work of reformulating a Markov decision process (MDP) as a linear program, and by adding the consistency constraint for MFGs in terms of occupation measures, and by exploiting the complementarity structure of the linear program. Under proper regularity conditions for the rewards and the dynamics of the game, the corresponding framework, called MF-OMO (Mean-Field Occupation Measure Optimization), is shown to provide convergence guarantees for finding multiple (and possibly all) NE solutions of MFGs by popular algorithms such as projected gradient descent. In particular, we show that analyzing the class of MFGs with linear rewards and mean-field independent dynamics can be reduced to solving a finite number of linear programs, hence solved in finite time. This optimization framework can be easily extended for variants of MFGs, including but not limited to personalized MFGs and multi-population MFGs.
更多查看译文
关键词
optimization formulation,mf-omo,mean-field
AI 理解论文
溯源树
样例
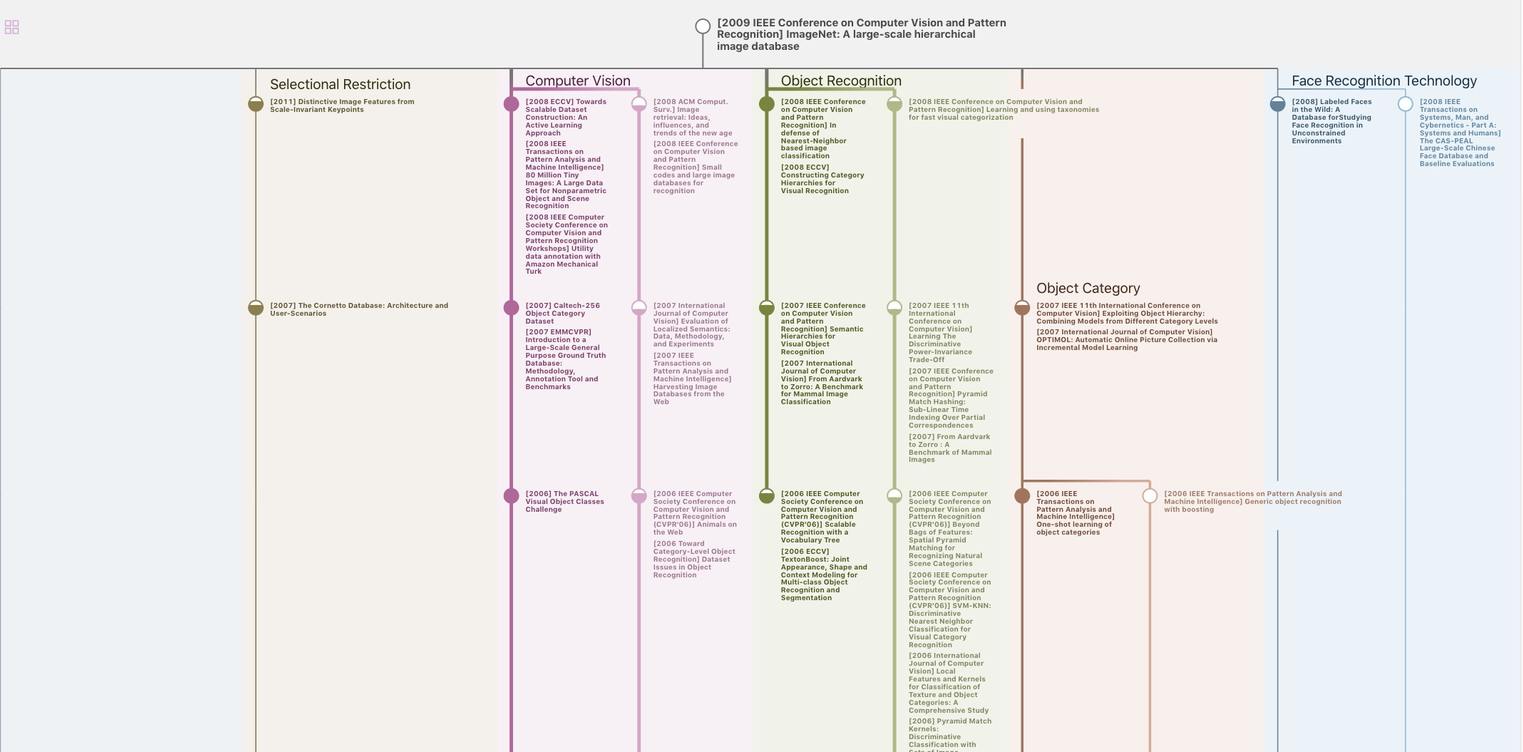
生成溯源树,研究论文发展脉络
Chat Paper
正在生成论文摘要