Event-Triggered privacy-preserving average consensus for continuous-time multi-agent network systems
Journal of the Franklin Institute(2022)
摘要
This paper deals with the privacy-preserving average consensus problem for continuous-time multi-agent network systems (MANSs) based on the event-triggered strategy. A novel event-triggered privacy-preserving consensus algorithm is designed to achieve the average consensus of MANSs while avoiding the disclosure of the agents’ initial states. Different from the approaches incorporating stochastic noises, an output mask function in the proposed algorithm is developed to make initial state of each agent indiscernible by the others. Particularly, under the output mask function, all agents can exactly tend to the average value of initial states rather than the mean square value. Under the proposed algorithm, detailed theoretical proof about average consensus and privacy of the MANSs are conducted. Moreover, the proposed algorithm is extended to nonlinear continuous-time MANSs, and the corresponding results are also derived. A numerical simulation eventually is performed to demonstrate the validity of our results.
更多查看译文
关键词
Privacy preservation,Multi-agent network system,Average consensus,Event-triggered strategy,Zeno-behavior
AI 理解论文
溯源树
样例
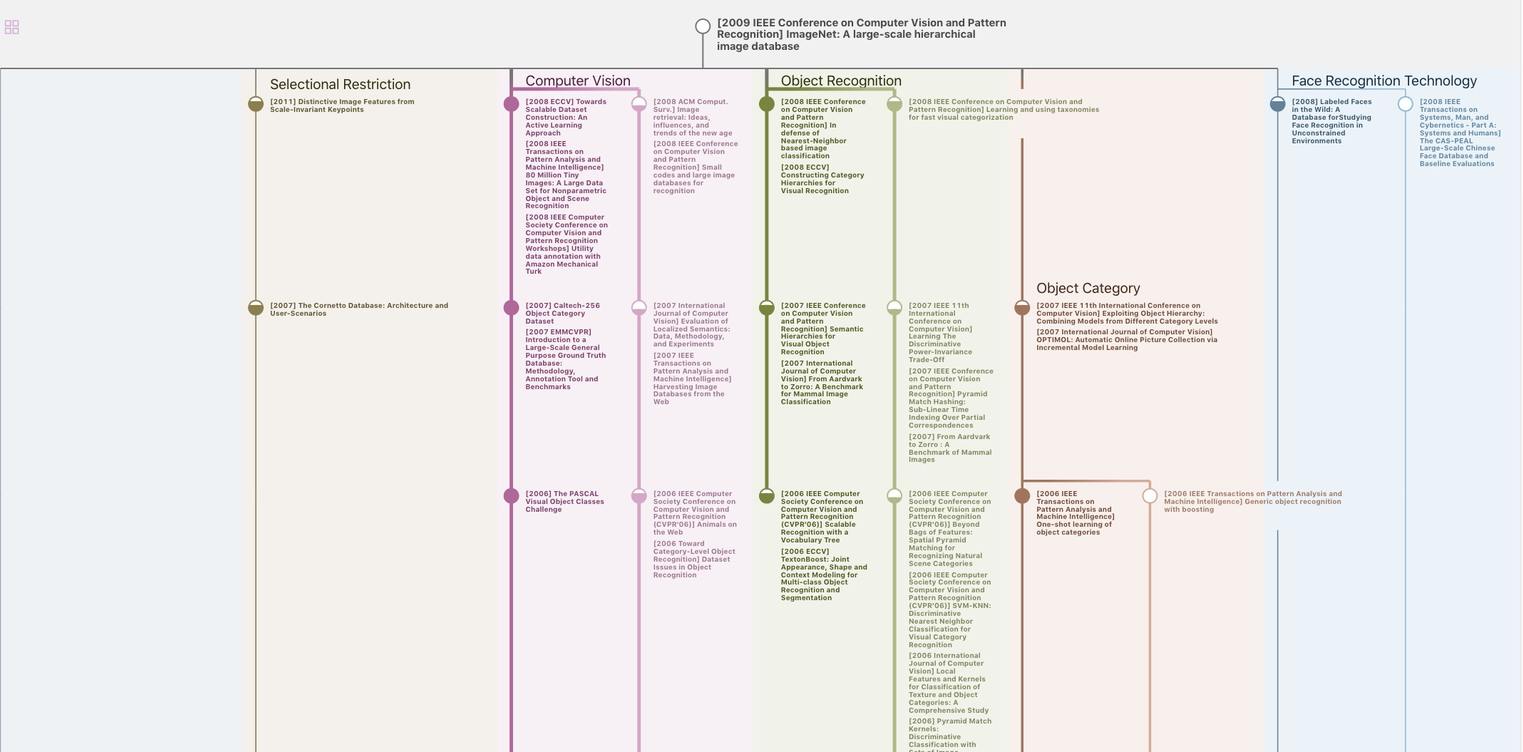
生成溯源树,研究论文发展脉络
Chat Paper
正在生成论文摘要