Asset splitting algorithm for ultrahigh dimensional portfolio selection and its theoretical property
JOURNAL OF ECONOMETRICS(2024)
摘要
The presence of a huge number of assets poses challenges to classical portfolio selection algorithms. Constrained l1 minimization approaches have been proposed to directly estimate effective parameters in the optimal portfolio. Linear programming method and alternating direction method of multiplier (ADMM) algorithm is used to solve the corresponding minimization problems. However, these two algorithms may fail due to the limitations of computing time and computing memory when a huge number of assets are considered in the portfolio optimization. This article proposes an asset splitting ADMM (AS-ADMM for short), a parallel computing algorithm, to tackle such challenges, and establishes the convergence property of the new algorithm. Furthermore, we develop a new regularization method for estimating the effective parameters with the folded-concave penalty and establish its oracle property. The local linear approximation (LLA) algorithm is used to redirect the new method to a weighted l1 regularization method. We conduct simulation studies to investigate the advantage of the proposed algorithm and regularized model in solving the high dimensional portfolio selection problems. A real data example is also included to demonstrate the applicability of the proposed algorithms and regularization methods. (c) 2022 Elsevier B.V. All rights reserved.
更多查看译文
关键词
ADMM,High dimensional portfolio selection,Parallel computing
AI 理解论文
溯源树
样例
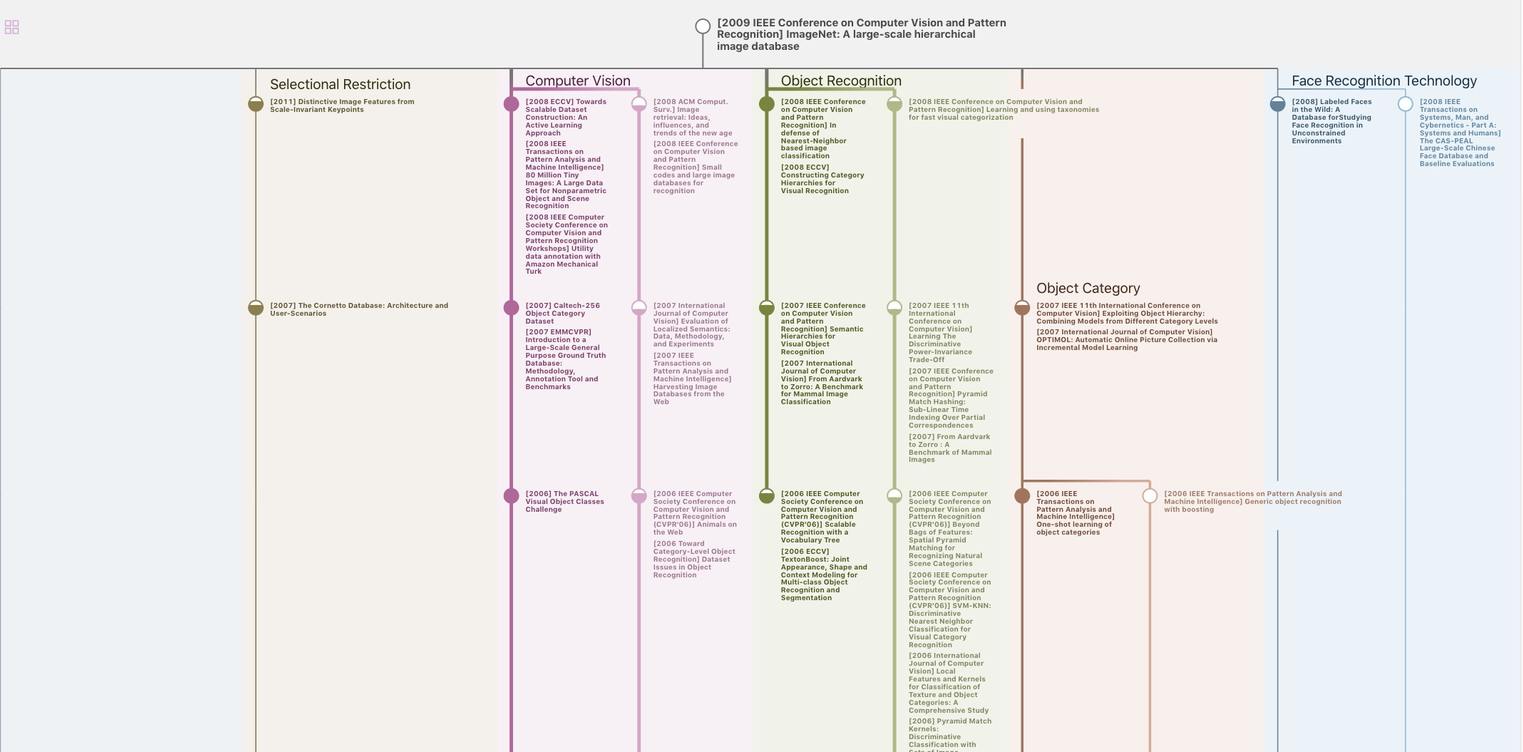
生成溯源树,研究论文发展脉络
Chat Paper
正在生成论文摘要