Full transformer network with masking future for word-level sign language recognition
Neurocomputing(2022)
摘要
Word-level sign language recognition (SLR) is a significant task which transcribes a sign language video into a word. Currently, deep-learning-based frameworks mostly combine spatial feature extractors based on convolution neural networks (CNNs) and sequence learners. These methods either lack the sufficient capacity to establish the high-level vision semantic knowledge and incorporate the details in images or perform weak intelligence on video frame sequence comprehension. Focusing on gestures and facial expressions is essential to interpreting sign language; however, it is challenging to crop these elements from pictures and distill them end-to-end. In this paper, a full self-attention framework for word-level SLR is proposed to tackle the above issue, which integrates a Vision Transformer as spatial encoder and an improved temporal Transformer. In addition, we introduce the masking future operation to improve the Transformer for the temporal module. The vision Transformer first refines the latent high-level semantic feature sequences from sign language videos and feeds them into the temporal module. Then the masking future Transformer enhances this sequence by making subsequent time invisible at each moment of frames and generates the final recognition. This approach integrates global and local spatial information; furthermore, it can also distinguish the latent semantic features contained in sign language action sequences. To validate the proposed approach, we perform extensive experiments on two datasets. The results and ablation studies demonstrate the effectiveness of this method, and it achieves new state-of-the-art performance on the WLASL dataset by using RGB images alone.
更多查看译文
关键词
Word-level sign language recognition,Transformer,Mask Future
AI 理解论文
溯源树
样例
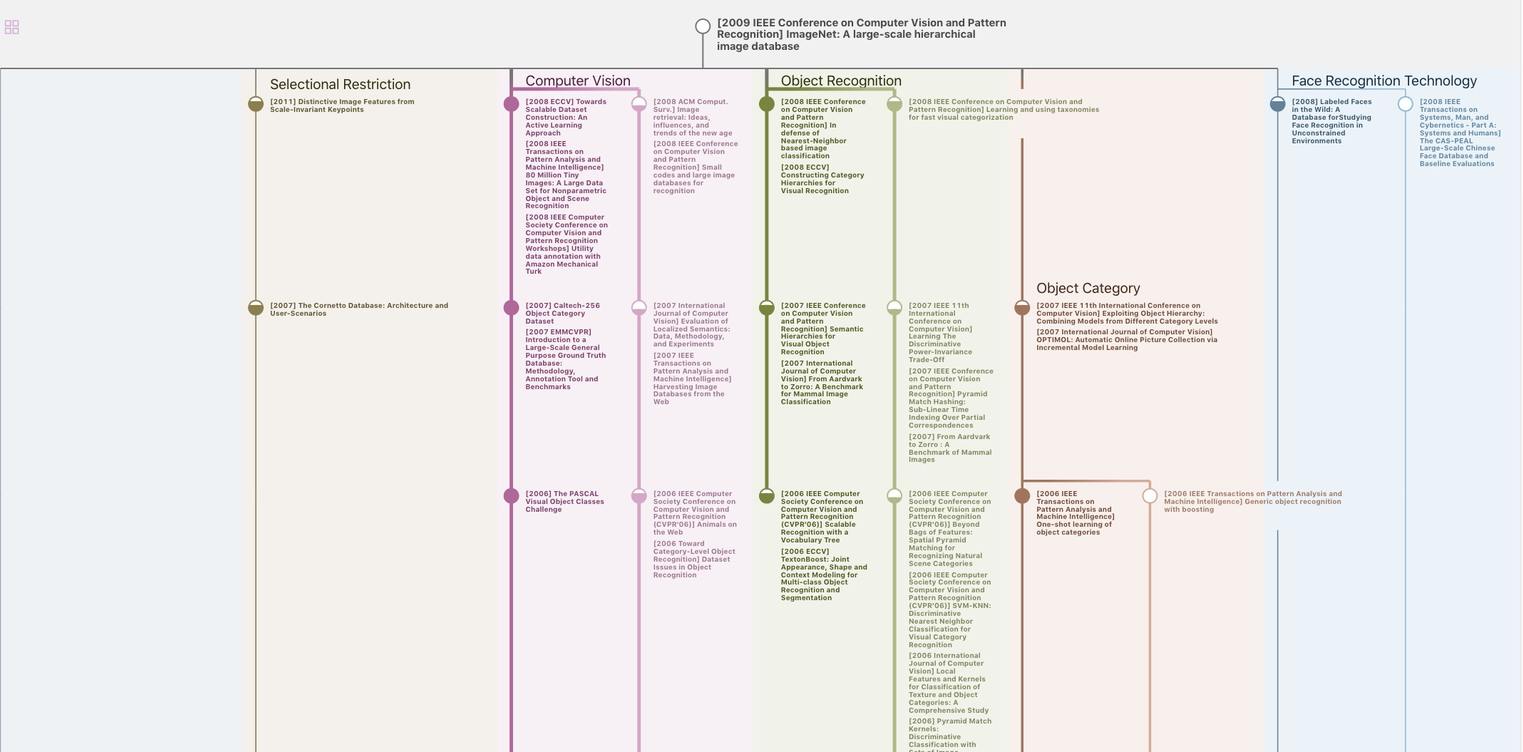
生成溯源树,研究论文发展脉络
Chat Paper
正在生成论文摘要