Weight-Guided Loss for Long-Tailed Object Detection and Instance Segmentation
Social Science Research Network(2022)
摘要
The long-tailed characteristic leads to a significant performance drop for various models on long-tailed distribution datasets. Existing works mainly focus on mitigating the data shortage in tail classes at dataset level by data re-sampling, loss re-weighting or knowledge transfer from head to tail. While in this paper, we focus on another perspective which is also related to the performance drop: the gap between total dataset class number and training batch size. To address this issue, we propose a Weight-Guided (WG) loss which utilizes the classifier weights as auxiliary tail samples. It can be easily deployed to different models. By simply adding WG loss to Mask R-CNN with ResNet-50 backbone, we improve the performance by (i) 0.5 box AP and 0.4 mask AP on COCO dataset, (ii) 0.4 box and mask AP (1.8 mask AP for rare classes) on LVIS v1.0 dataset. Codes will be released.
更多查看译文
关键词
Long-tail,Object detection,Instance segmentation
AI 理解论文
溯源树
样例
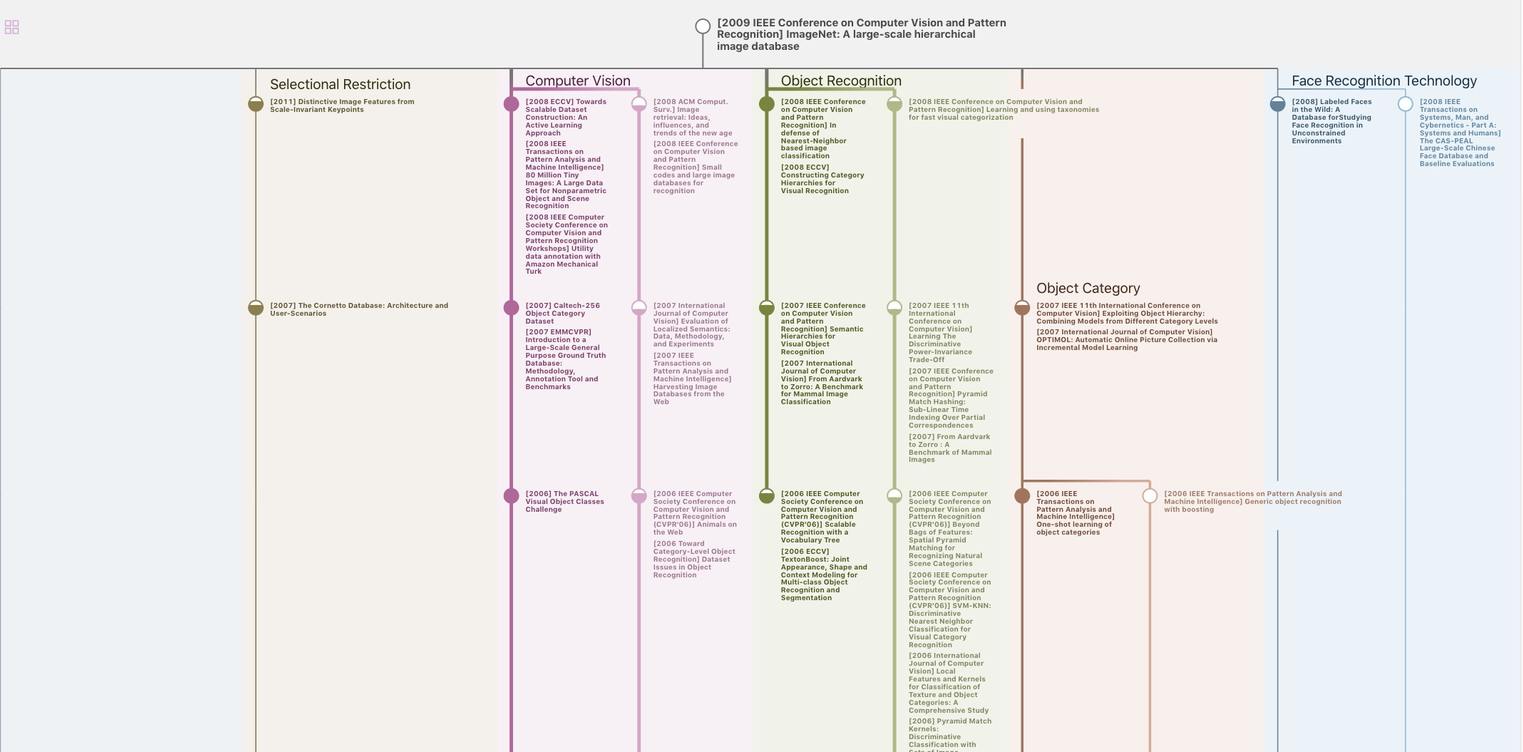
生成溯源树,研究论文发展脉络
Chat Paper
正在生成论文摘要