A Shallow Neural Network for Recognition of Strip Steel Surface Defects Based on Attention Mechanism
Social Science Research Network(2022)
摘要
This research proposes an efficient strip steel surface defect classification model (ASNet) based on convolutional neural network (CNN), which can run in real time on commonly used serial computing platforms. We only used a very shallow CNN structure to extract features of the defect images, and an attention layer which makes the model ignore some irrelevant noise and obtain an effective description of the defects is designed. In addition, a nonlinear perceptron is added to the top of the model to recognize defects based on the extracted features. On the strip steel surface defect image dataset NEU-CLS, our model achieves an average classification accuracy of 99.9%, while the number of parameters of the model is only 0.041 M and the computational complexity of the model is 98.1 M FLOPs. It can meet the requirements of real-time operation and large-scale deployment on a common serial computing platform with high recognition accuracy.
更多查看译文
关键词
strip steel defects,convolutional neural network,attention mechanism,lightweight model
AI 理解论文
溯源树
样例
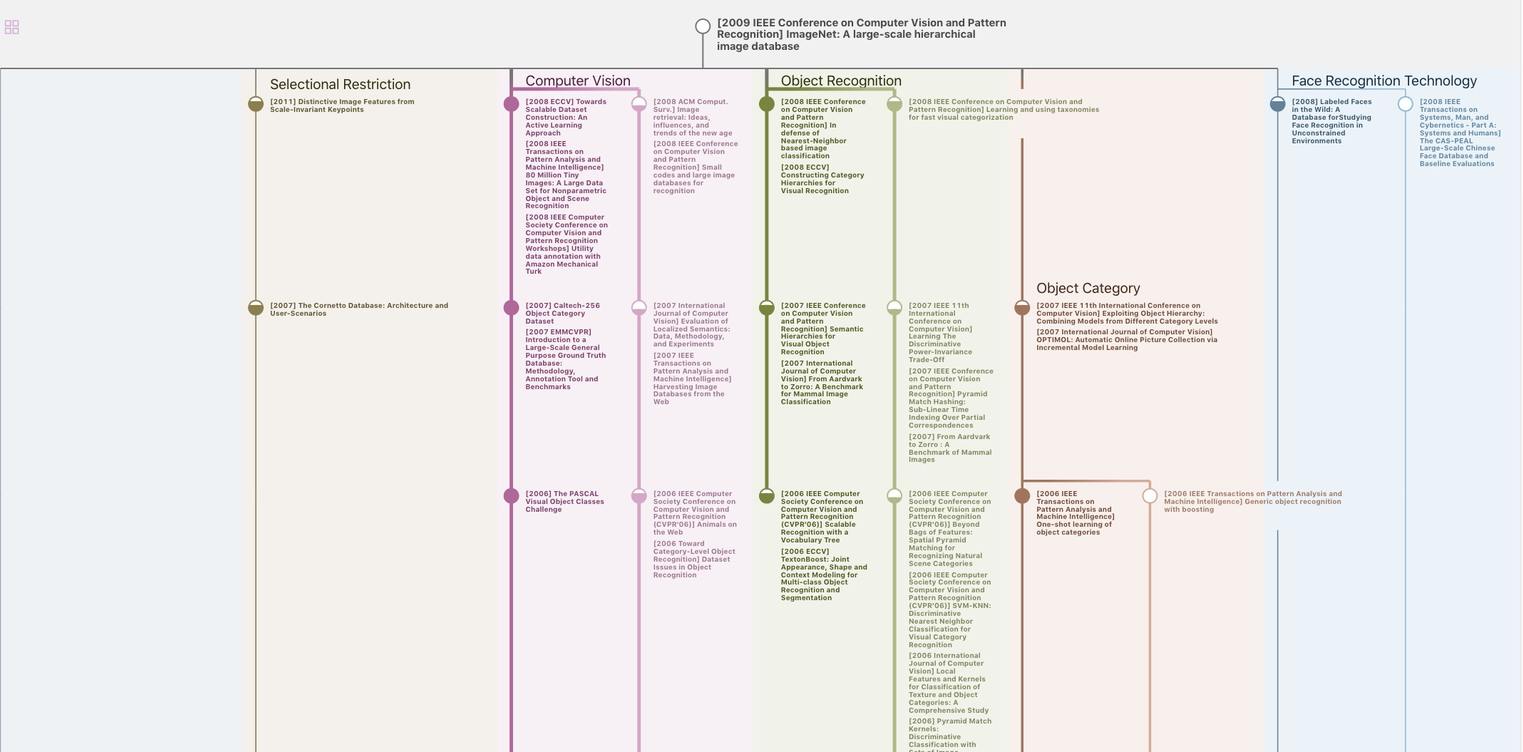
生成溯源树,研究论文发展脉络
Chat Paper
正在生成论文摘要