Discriminator Based Resilient Multi-agent Deep Deterministic Policy Gradient Under Uncertain Faulty Agents
Journal of physics(2022)
摘要
Abstract In recent years, Multi-agent reinforcement learning (MARL) is widely applied in various of fields, to achieve a global goal in a centralized or distributed manner. However, during its application it is crucial to be fault-tolerance as some agents behave abnormal. In this paper, we propose a Resilient Multi-gent Deep Deterministic Policy Gradient (RMADDPG) algorithm to achieve a cooperative task in the presence of faulty agents via centralized training decentralized execution. At training stage, each normal agent observes and records information only from other normal ones, without access to the faulty ones. Meanwhile, a discriminator is generated based on the well-trained actor network to identify each faulty agent via supervised learning. Followed by executing stage, each normal agent selects its action based on local observation according to its actor network and its discriminator, so as to achieve certain system goal. Specifically, RMADDPG offers a scheme to train agents for improved resilience against arbitrary number of faulty agents. Finally, a cooperative navigation experiment is provided to validate the effectiveness of the proposed algorithm.
更多查看译文
AI 理解论文
溯源树
样例
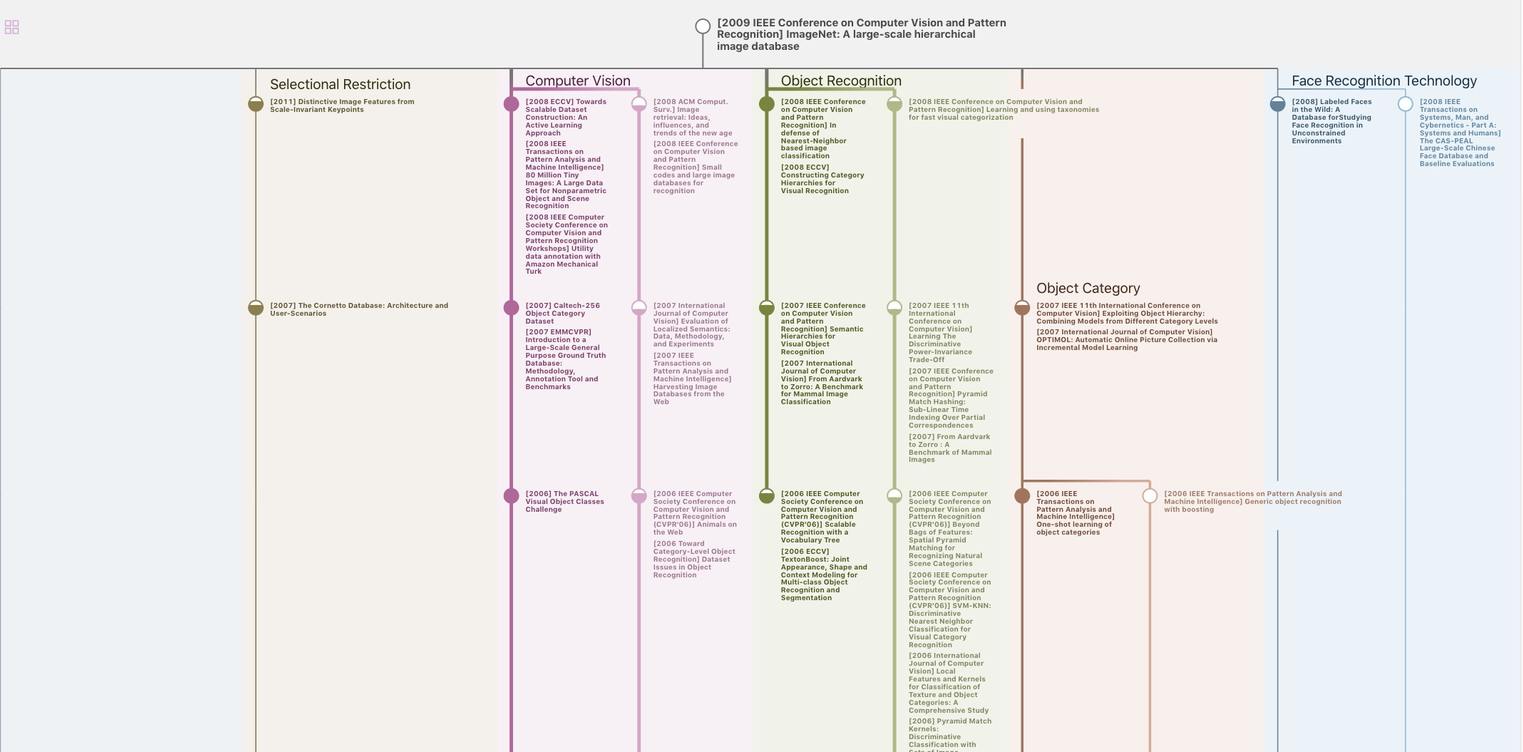
生成溯源树,研究论文发展脉络
Chat Paper
正在生成论文摘要