Land cover classification in a mixed forest-grassland ecosystem using LResU-net and UAV imagery
Journal of Forestry Research(2021)
摘要
Using an unmanned aerial vehicle (UAV) paired with image semantic segmentation to classify land cover within natural vegetation can promote the development of forest and grassland field. Semantic segmentation normally excels in medical and building classification, but its usefulness in mixed forest-grassland ecosystems in semi-arid to semi-humid climates is unknown. This study proposes a new semantic segmentation network of LResU-net in which residual convolution unit (RCU) and loop convolution unit (LCU) are added to the U-net framework to classify images of different land covers generated by UAV high resolution. The selected model enhanced classification accuracy by increasing gradient mapping via RCU and modifying the size of convolution layers via LCU as well as reducing convolution kernels. To achieve this objective, a group of orthophotos were taken at an altitude of 260 m for testing in a natural forest-grassland ecosystem of Keyouqianqi, Inner Mongolia, China, and compared the results with those of three other network models (U-net, ResU-net and LU-net). The results show that both the highest kappa coefficient (0.86) and the highest overall accuracy (93.7%) resulted from LResU-net, and the value of most land covers provided by the producer’s and user’s accuracy generated in LResU-net exceeded 0.85. The pixel-area ratio approach was used to calculate the real areas of 10 different land covers where grasslands were 67.3%. The analysis of the effect of RCU and LCU on the model training performance indicates that the time of each epoch was shortened from U-net (358 s) to LResU-net (282 s). In addition, in order to classify areas that are not distinguishable, unclassified areas were defined and their impact on classification. LResU-net generated significantly more accurate results than the other three models and was regarded as the most appropriate approach to classify land cover in mixed forest-grassland ecosystems.
更多查看译文
关键词
UAV images, Semantic segmentation, LResU-net, Land cover classification
AI 理解论文
溯源树
样例
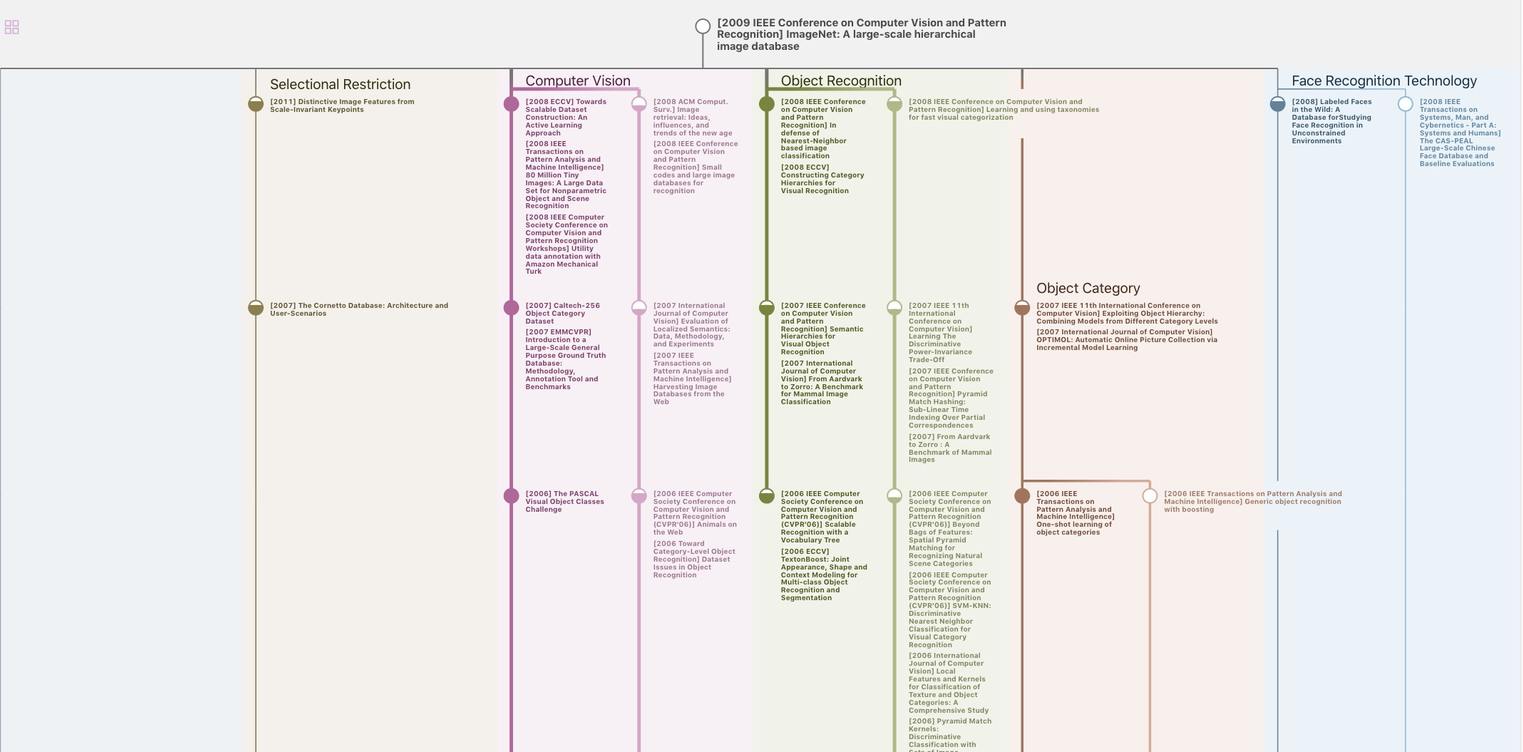
生成溯源树,研究论文发展脉络
Chat Paper
正在生成论文摘要