Toward Large-Scale Evolutionary Multitasking: A GPU-Based Paradigm
IEEE Transactions on Evolutionary Computation(2022)
摘要
Evolutionary multitasking (EMT), which shares knowledge across multiple tasks while the optimization progresses online, has demonstrated superior performance in terms of both optimization quality and convergence speed over its single-task counterpart in solving complex optimization problems. However, most of the existing EMT algorithms only consider handling two tasks simultaneously. As the computational cost incurred in the evolutionary search and knowledge transfer increased rapidly with the number of optimization tasks, these EMT algorithms cannot meet today’s requirements of optimization service on the cloud for many real-world applications, where hundreds or thousands of optimization requests (labeled as large-scale EMT) are often received simultaneously and require to be optimized in a short time. Recently, graphics processing unit (GPU) computing has attracted extensive attention to accelerate the applications possessing large-scale data volume that are traditionally handled by the central processing unit (CPU). Taking this cue, toward large-scale EMT, in this article, we propose a new EMT paradigm based on the island model with the compute unified device architecture (CUDA), which is able to handle a large number of continuous optimization tasks efficiently and effectively. Moreover, under the proposed paradigm, we develop the GPU-based
implicit
and
explicit
knowledge transfer mechanisms for EMT. To evaluate the performance of the proposed paradigm, comprehensive empirical studies have been conducted against its CPU-based counterpart in large-scale EMT.
更多查看译文
关键词
Compute unified device architecture (CUDA),evolutionary multitasking (EMT),graphics processing unit (GPU) computing,knowledge transfer,optimization
AI 理解论文
溯源树
样例
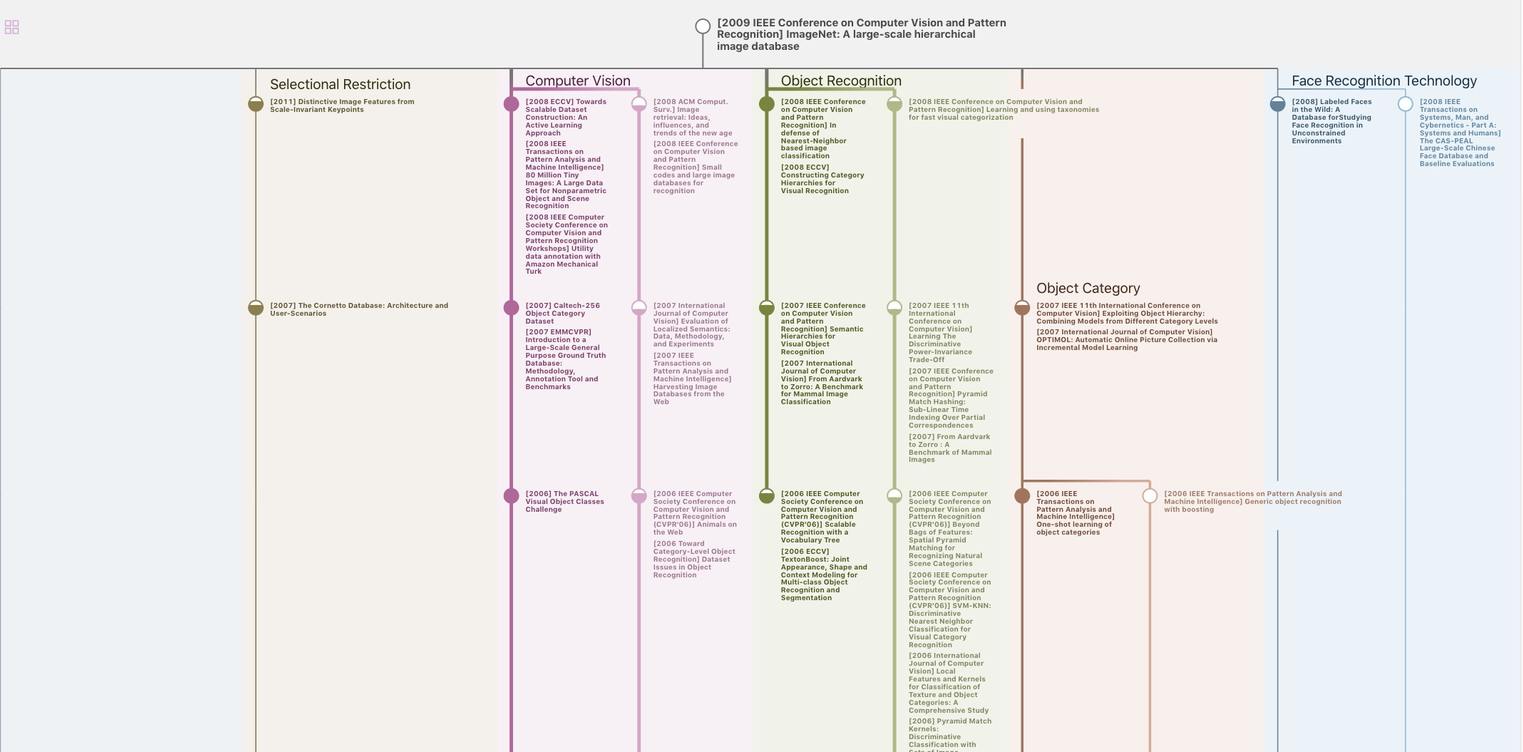
生成溯源树,研究论文发展脉络
Chat Paper
正在生成论文摘要