Boosting Graph Structure Learning with Dummy Nodes.
International Conference on Machine Learning(2022)
摘要
With the development of graph kernels and graph representation learning, many superior methods have been proposed to handle scalability and oversmoothing issues on graph structure learning. However, most of those strategies are designed based on practical experience rather than theoretical analysis. In this paper, we use a particular dummy node connecting to all existing vertices without affecting original vertex and edge properties. We further prove that such the dummy node can help build an efficient monomorphic edge-to-vertex transform and an epimorphic inverse to recover the original graph back. It also indicates that adding dummy nodes can preserve local and global structures for better graph representation learning. We extend graph kernels and graph neural networks with dummy nodes and conduct experiments on graph classification and subgraph isomorphism matching tasks. Empirical results demonstrate that taking graphs with dummy nodes as input significantly boosts graph structure learning, and using their edge-to-vertex graphs can also achieve similar results. We also discuss the gain of expressive power from the dummy in neural networks.
更多查看译文
关键词
graph structure learning
AI 理解论文
溯源树
样例
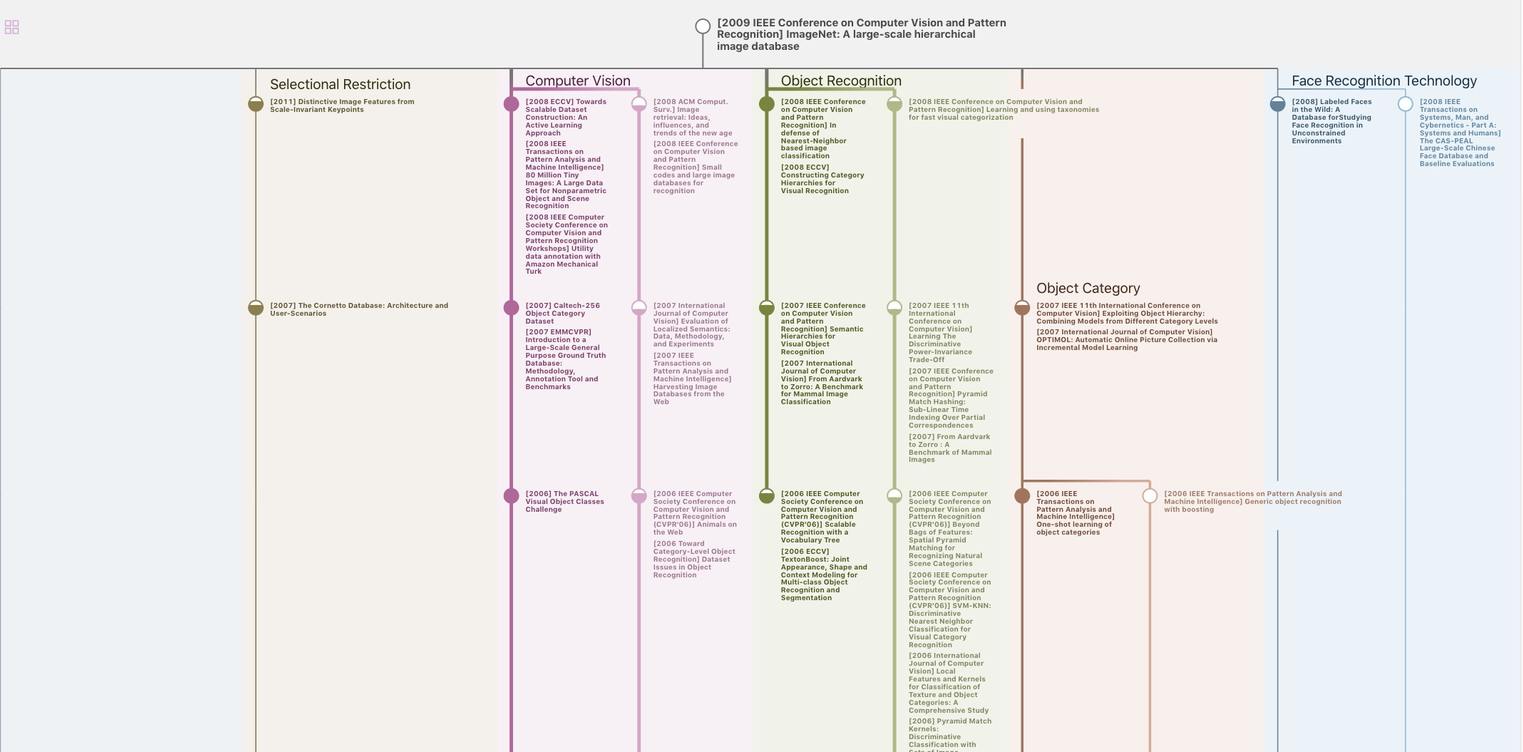
生成溯源树,研究论文发展脉络
Chat Paper
正在生成论文摘要