Unsupervised Kinematic Motion Detection for Part-segmented 3D Shape Collections
International Conference on Computer Graphics and Interactive Techniques(2022)
摘要
BSTRACT3D models of manufactured objects are important for populating virtual worlds and for synthetic data generation for vision and robotics. To be most useful, such objects should be articulated: their parts should move when interacted with. While articulated object datasets exist, creating them is labor-intensive. Learning-based prediction of part motions can help, but all existing methods require annotated training data. In this paper, we present an unsupervised approach for discovering articulated motions in a part-segmented 3D shape collection. Our approach is based on a concept we call category closure: any valid articulation of an object’s parts should keep the object in the same semantic category (e.g. a chair stays a chair). We operationalize this concept with an algorithm that optimizes a shape’s part motion parameters such that it can transform into other shapes in the collection. We evaluate our approach by using it to re-discover part motions from the PartNet-Mobility dataset. For almost all shape categories, our method’s predicted motion parameters have low error with respect to ground truth annotations, outperforming two supervised motion prediction methods.
更多查看译文
关键词
unsupervised kinematic motion detection,3d shape
AI 理解论文
溯源树
样例
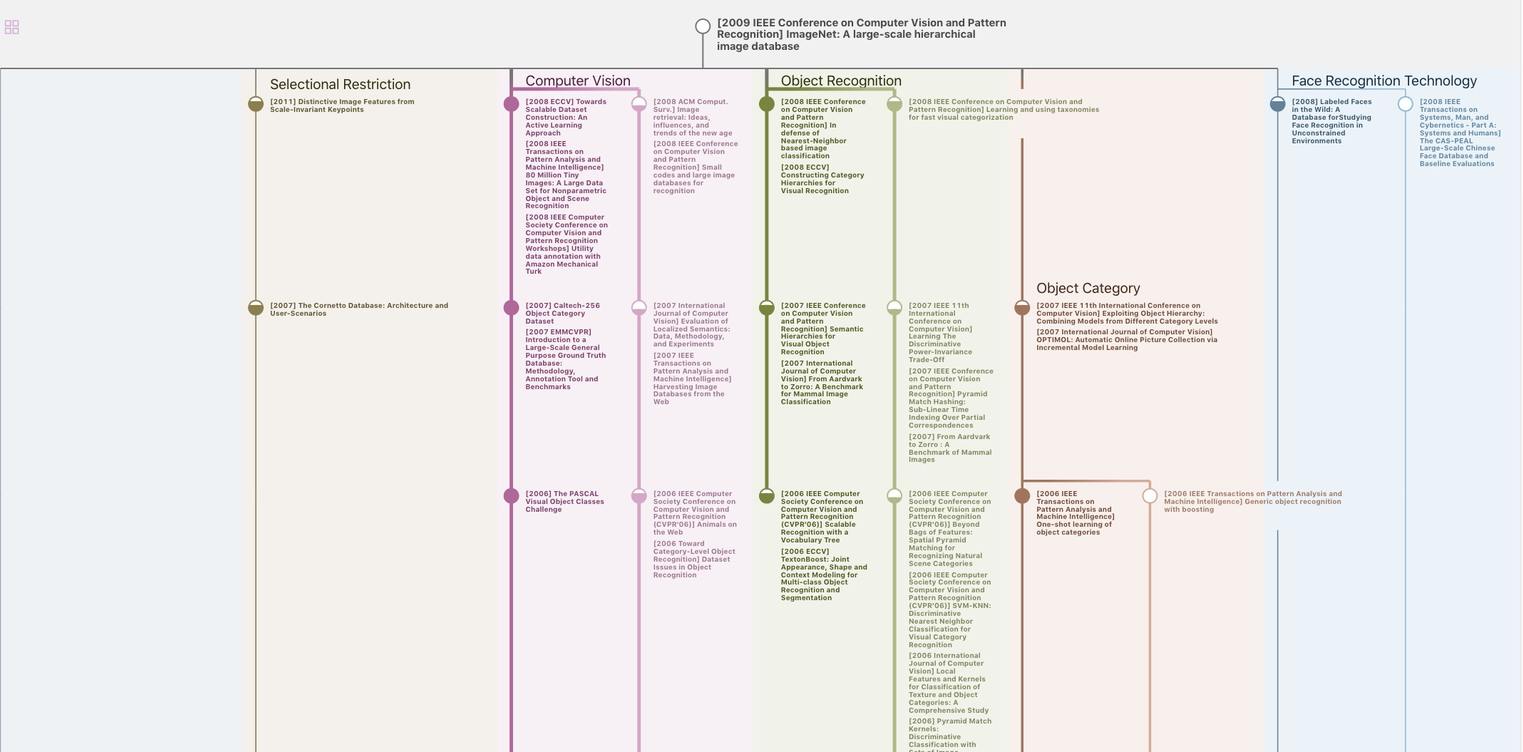
生成溯源树,研究论文发展脉络
Chat Paper
正在生成论文摘要