Identifying network biomarkers of cancer by sample-specific differential network
BMC Bioinformatics(2022)
摘要
Abundant datasets generated from various big science projects on diseases have presented great challenges and opportunities, which contributed to unfolding the complexity of diseases. The discovery of disease-associated molecular networks for each individual plays an important role in personalized therapy and precision treatment of cancer-based on the reference networks. However, there are no effective ways to distinguish the consistency of different reference networks. In this study, we developed a statistical method, i.e. a sample-specific differential network (SSDN), to construct and analyze such networks based on gene expression of a single sample against a reference dataset. We proved that the SSDN is structurally consistent even with different reference datasets if the reference dataset can follow certain conditions. The SSDN also can be used to identify patient-specific disease modules or network biomarkers as well as predict the potential driver genes of a tumor sample.
更多查看译文
关键词
Single-sample-differential-network, Gene-expression-data, Cancer driver-gene, Enrichment analysis
AI 理解论文
溯源树
样例
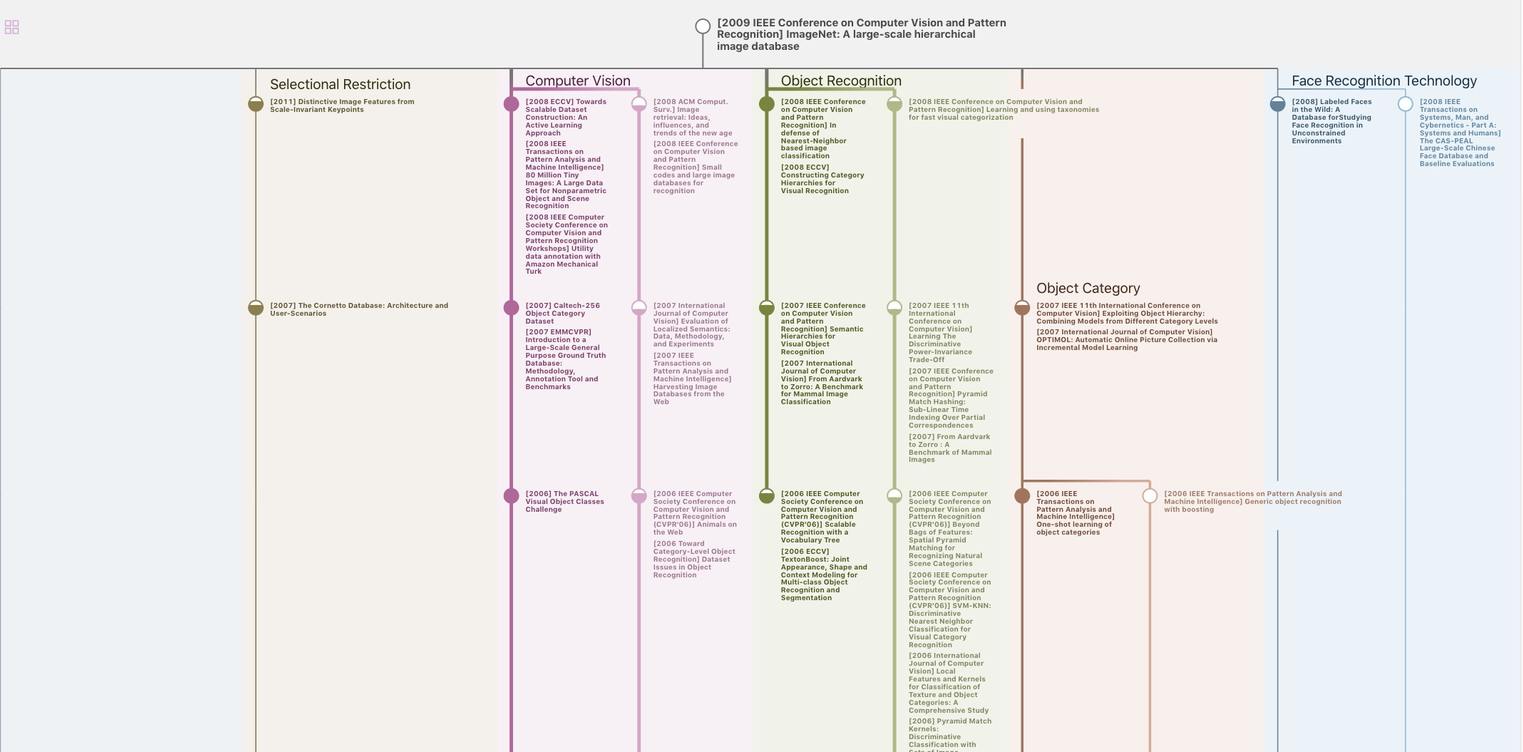
生成溯源树,研究论文发展脉络
Chat Paper
正在生成论文摘要