Threat Modeling Intimate Partner Violence: Tech Abuse as a Cybersecurity Challenge in the Internet of Things
The Emerald International Handbook of Technology-Facilitated Violence and Abuse(2021)
摘要
Technology-facilitated abuse, so-called “tech abuse,” through phones, trackers, and other emerging innovations, has a substantial impact on the nature of intimate partner violence (IPV). The current chapter examines the risks and harms posed to IPV victims/survivors from the burgeoning Internet of Things (IoT) environment. IoT systems are understood as “smart” devices such as conventional household appliances that are connected to the internet. Interdependencies between different products together with the devices' enhanced functionalities offer opportunities for coercion and control. Across the chapter, we use the example of IoT to showcase how and why tech abuse is a socio-technological issue and requires not only human-centered (i.e., societal) but also cybersecurity (i.e., technical) responses. We apply the method of “threat modeling,” which is a process used to investigate potential cybersecurity attacks, to shift the conventional technical focus from the risks to systems toward risks to people. Through the analysis of a smart lock, we highlight insufficiently designed IoT privacy and security features and uncover how seemingly neutral design decisions can constrain, shape, and facilitate coercive and controlling behaviors. Keywords Tech abuse Intimate partner violence Domestic violence Cybersecurity Threat modeling Internet of things Citation Slupska, J. and Tanczer, L.M. (2021), "Threat Modeling Intimate Partner Violence: Tech Abuse as a Cybersecurity Challenge in the Internet of Things", Bailey, J., Flynn, A. and Henry, N. (Ed.) The Emerald International Handbook of Technology-Facilitated Violence and Abuse (Emerald Studies In Digital Crime, Technology and Social Harms), Emerald Publishing Limited, Bingley, pp. 663-688. https://doi.org/10.1108/978-1-83982-848-520211049 Publisher: Emerald Publishing Limited Copyright © 2021 Julia Slupska and Leonie Maria Tanczer. Published by Emerald Publishing Limited. This chapter is published under the Creative Commons Attribution (CC BY 4.0) licence. Anyone may reproduce, distribute, translate and create derivative works of these chapters (for both commercial and non-commercial purposes), subject to full attribution to the original publication and authors. The full terms of this licence may be seen at http://creativecommons.org/licences/by/4.0/legalcode. License This chapter is published under the Creative Commons Attribution (CC BY 4.0) licence. Anyone may reproduce, distribute, translate and create derivative works of these chapters (for both commercial and non-commercial purposes), subject to full attribution to the original publication and authors. The full terms of this licence may be seen at http://creativecommons.org/licences/by/4.0/legalcode. “I changed the lock on my front door so you can't see me anymore. And you can't come inside my house, and you can't lie down on my couch. I changed the lock on my front door” —Lucinda Williams “Changed the Locks”. Introduction Technology-facilitated abuse or “tech abuse” through Global Positioning System (GPS) trackers, smartphone apps, or platforms such as Facebook has a substantial impact on the nature of intimate partner violence (IPV). The latter encompasses diverse forms of abuse (e.g., physical, sexual, financial) and coercive and controlling behavior by a (current or former) partner or spouse (Bagwell-Gray, Messing, & Baldwin-White, 2015). IPV, and more specifically, domestic abuse 1 globally affects about 1 in 3 (35% of) women in their lifetime (World Health Organization, 2017) and more than 2.4 million UK adults a year (Office for National Statistics, 2019). Parallel to the widespread deployment of technologies, their misuse, especially in the context of domestic and sexual violence, is increasing. While national figures remain absent (Tanczer, Neira, Parkin, & Danezis, 2018), data points gathered by charities such as Think Social Tech, Snook and SafeLives (2019), and Women's Aid (2018) point to the rising scale as well as the urgency of this issue. According to Refuge (2020), the UK's largest domestic violence charity, more than 72% of their service users experience abuse through technology. Furthermore, emerging technologies such as smart, internet-connected devices have begun to enter our households. These so-called “Internet of Things” (IoT) range from gadgets such as “smart speakers,” as well as embedded infrastructures such as connected thermostats, blinds, or locks. IoT devices open up new avenues to remotely monitor, control, and harass victims/survivors (Parkin, Patel, Lopez-Neira, & Tanczer, 2019). 2 Their interconnectedness and growing level of sophistication make them tools to help facilitate other coercive and controlling offenses, including stalking. The current chapter sets out to examine the risks and harms 3 that derive from the burgeoning IoT environment. We showcase how and why tech abuse is a socio-technological issue that requires not only human-centered (i.e., societal), but also cybersecurity (i.e., technical) responses. We thus use the notion of “threat modeling,” which is a process that investigates potential cybersecurity attacks to focus on the risks both to systems and to people (Uzunov & Fernandez, 2014). Through the analysis of a smart lock, we exemplify insufficiently addressed dangers and uncover how seemingly neutral design decisions can constrain, shape, and facilitate coercive and controlling behaviors. Existing Research IoT-Enabled Technology-Facilitated Abuse The proliferation of so-called smart, internet-connected devices poses a new tech abuse challenge. The move toward IoT includes the direct and indirect extension of the internet into a range of physical objects, devices, and products, with a broad range of applications (Tanczer, Brass, Elsden, Carr, & Blackstock, 2019, p. 37). Previously “offline” and “unrelated” technologies such as conventional household appliances are now being interconnected and become part of a network which allows them to – put simply – “speak” to one another. While IoT systems range from tiny sensors to large-scale cyber-physical systems such as cars, consumer IoT devices form a dominant focus of ongoing analyses. Consumer IoT describe systems created to be used by “average” end users in a personal capacity and/or within the home setting. Such devices include, for example, smart speakers, wearables, and a range of security systems. In the UK, 31% of the 35–44 age group own three or more connected devices with IoT usage expected to increase significantly over the next decades (Tech UK, 2019). IoT appliances not only collect reams of information, including personal data, preference settings, and usage patterns, but offer an opportunity to be remotely controlled. Combined with features such as video and audio recording functionalities, IoT devices open up significant exploitative avenues in an IPV context (Leitão, 2019). Society is therefore in urgent need to understand the broader classes of harms IoT systems may cause and conceptualize how these harms could move beyond “conventional” understandings of safety, security, and privacy. So far, the research on IoT-affiliated tech abuse in the context of IPV is in its infancy. Only a handful of studies have evaluated the tech abuse risks that derive from the deployment of smart devices in the home. Leitão (2018, 2019) examined the potential security and privacy threats that victims/survivors of IPV would face. Strengers, Kennedy, Arcari, Nicholls, and Gregg (2019) conducted an ethnographic study with early IoT adopters. They showed that women need to be able to operate IoT systems safely and securely without exposing themselves or others to additional internal or external threats. Slupska (2019) reviewed 40 smart home security papers and uncovered that the only article that explicitly addressed IPV in their analysis was dismissive of the risk potential and displaced the responsibility of protection onto potential targets of abuse. Besides, the Gender and IoT research project at University College London conducted a usability analysis of the shared device ecosystem (Parkin et al., 2019), exposing, among other findings, that the lack of security and privacy prompts can negatively impact tech abuse victims/survivors. Based on their findings, the research team produced guides and resources for the IPV support sector (Tanczer, Patel, Parkin, & Danezis, 2018, 2019) and briefings for the policy community (Tanczer, Lopez-Neira et al., 2018). Despite the limited evidence-base on IoT-enabled tech abuse, emerging classes of harms have to be evaluated in light of the dynamics of IPV (Katerndahl, Burge, Ferrer, Becho, & Wood, 2010; de Lucena et al., 2016). For example, Matthews et al. (2017) developed a framework for organizing victims'/survivors' technology practices and challenges into three phases, including: physical control, escape, and life apart (see Fig. 39.1). While their research centered on “conventional” devices such as computers and phones, similar considerations will have to be applied to both the social and technical responses to IoT. Opens in a new window.Fig. 39.1. Three Phases of IPV that Affected Technology Use, Focusing on Privacy & Security Practices. Matthews et al.’s (2017) findings further showcase that tech abuse victims/survivors face high levels of stress and risk, which makes it harder for them to pay attention to user interface (UI) details. The latter are means by which a user interacts with and regulates a technical system. UI can be graphical controls such as one's home screen or the menu bar, as well as hardware devices such as a remote, switch, or keyboard (Myers, 1989). Victims/survivors are consequently disadvantaged in making use of privacy and security features and struggle to identify, access, and act upon instruction materials (e.g., how to block a phone number, how to set up multi-factor authentication). 4 Drawing on these insights, Matthews et al. (2017) suggested that technology designers should consider the usability of their inventions and acknowledge the distinct privacy and security requirements of IPV victims/survivors. This focus seems of high relevance looking at the limited (i.e., fewer buttons) as well as dispersed (i.e., control through the device as well as an app on the phone) interfaces IoT technologies such as smart speakers offer. Yet, as the upcoming section will display, the tech sector has so far ignored the potential challenges that these devices create and failed to implement technical responses to the daily privacy and security trade-offs that IPV victims/survivors must make (Freed et al., 2018; Slupska, 2019). Cybersecurity Design Shortcomings The threats and consequent risks deriving from IPV are almost absent in the cybersecurity literature as well as practice and even less of a discussion point in the emerging field of IoT (Slupska, 2019; Tanczer et al., 2018). Instead, the cybersecurity community often focuses on “hard” technical problems posed by remote “external” adversaries who exploit hardware or software vulnerabilities. Yet, as Freed et al. (2018) pointed out, most IPV attacks are technologically unsophisticated. This allows perpetrators to interact with a victim's/survivor's device or account via their standard settings, generic UI, or by simply downloading and installing a ready-made application that facilitates, for instance, the spying on a victim/survivor. Hence, the “typical” tech abuse perpetrators must be thought of as a “UI-bound adversary” (Freed et al., 2018). This perspective distinguishes IPV perpetrators from the cybercriminals who are the central focal point of cybersecurity research. In fact, perpetrators' lack of technical skill carries the risk that a focus on tech abuse could be dismissed as trivial. However, the socio-technical and interpersonal factors that characterize tech abuse undermine the foundational assumptions under which current digital systems have been designed and built (i.e., insider vs. outsider; legitimate vs. illegitimate user etc.). For example, “safety features” of devices, such as location tracking, are co-opted by abusers for surveillance purposes. This dynamic makes IPV attacks both challenging to technically counteract but also extremely damaging to victims/survivors. Like most digital products and services, smart home systems are based on an “authentication model.” This model implies that features such as passwords and security questions guarantee that an unauthenticated user (i.e., individual without login credentials) cannot access the system. However, IPV perpetrators are often aware of sign-in details, either because they purchased, installed, and maintained the device, or because they convinced or coerced the victim/survivor into sharing the information. Some perpetrators may be able to guess credentials due to personal knowledge they have of victims/survivors. Thus, in many IPV attack scenarios, the abuser is effectively “authenticated” and “authorized.” A possible parallel to the IPV tech abuse problem within the cybersecurity literature is the so-called “insider threat.” The latter describes a threat posed to an organization by rogue, disgruntled, or careless employees (Bishop & Gates, 2008; Nurse et al., 2014). Since employees often have access to login details, they are also authenticated adversaries. However, insider threats in the context of cybersecurity are almost always conceptualized as actors internal to the company (i.e., rogue employees) rather than internal to the home (i.e., family members). This narrow view of what “insider threat” implies is reflective of the corporate positionality of most cybersecurity research, which we hope to counterbalance in this chapter. The discussed oversights, including the focus on sophisticated attacks as well as corporate rather than domestic threats, are deficiencies of popular cybersecurity “threat models.” Threat models describe a systematic analysis of a probable attacker's profile, the most likely attack vectors, and the assets most desired by an attacker. Threat models, therefore, involve assumptions about likely attackers and can reflect biases and blind spots as seen in the exclusion of IPV perpetrators in cybersecurity practitioners' mental models. Readers may be alerted to the subjective nature of this process. To counter any skewed perspectives, we are arguing in favor of the deployment of thorough procedural methods as well as the inclusion of diverse voices – such as the IPV sector. So far, existing solutions to the problem of tech abuse have mostly involved the development of guidance to aid victims/survivors as well as support services (Online and Digital Abuse, 2018; Tanczer et al., 2018). Although such tools are useful, they shift responsibility onto victims/survivors. The latter already face significant cognitive, emotional, and financial constraints and are now further burdened having to check settings across a multitude of applications. Harris and Woodlock (2019) describe this additional onus as “safety work.” The authors argue that digital coercive control has led to new forms of victim-blaming which manifest itself in women being accused of having inflicted harm upon themselves by choosing to use certain devices and/or platforms. The fact that these systems are frequently victim's/survivor's primary link to their support network is overlooked. Furthermore, possible regional specificities, such as family-internal device sharing practices, are not accounted for (Sambasivan et al., 2019). Moving beyond these victim/survivor-straining proposals, existing issues associated with tech abuse are closely interlinked with the design of technological systems (Levy & Schneier, 2020). This is exemplified in reported cases of compromised webcams (Anderson, 2013), the repurposing of features such as real-time location sharing via Google Maps (Ashworth, 2018), or the review of victim's/survivor's historical queries and online searches by a perpetrator (Women's Aid, 2018). A common obstacle to the implementation of better IPV privacy and security measures stems from the fact that IoT's inherent functionalities (e.g., remote control, speech recognition) can equally benefit perpetrators as much as victims/survivors (Parkin et al., 2019). This “dual-use” problem – a term coined to describe the fact that digital systems may be designed for peaceful use but can also be co-opted for malicious purposes and vice versa – has been widely discussed in the cybersecurity literature (Nye, 2018; Riebe & Reuter, 2019). However, it has not yet been modeled onto the context of IPV. For example, a perpetrator may install a smart camera to spy on their partner, while a victim/survivor may install a smart camera to feel in control of their environment. The answer to who is being empowered by IoT is consequently dependent on who has control over the device and network. The adjustment of established cybersecurity methods like risk assessments, usability tests, and safety reviews can help tech vendors to consider adversarial users when designing and evaluating UIs (Freed et al., 2018; Parkin et al., 2019). On these grounds, we would like to put forward the idea of designing a dedicated “IPV Threat Model” to explore and document avenues for harming IPV victims/survivors. While not a panacea – as some proposed changes may, in some contexts, benefit perpetrators – such a framework can limit an IoT systems' “abusability” (i.e., its capacity to be abused) and account for the cybersecurity needs of some of the most vulnerable groups in society. A Method to Threat Model Intimate Partner Violence Tech Abuse Threat modeling describes a process used to analyze potential attack vectors on a system (Uzunov & Fernandez, 2014). The concept of “threat” is hereby understood as the probable cause of an incident that might result in harm to systems, individuals, and organizations (Sabbagh & Kowalski, 2015), with a “threat actor” being the entity who wishes to cause a – usually negative – impact (Coles & Tarandach, 2020). While threats may arise from both accidental and deliberate activities of “legitimate users” (the owner/account holder of a device; Omotosho, Haruna, & Olaniyi, 2019), we assume that an IPV threat actor may be a perpetrator who intentionally abuses specific technical features to monitor, control, or coerce a victim/survivor. Thus, the perpetrator may be an authorized user yet still abuses the system for illegitimate means. We acknowledge that the literature on threat modeling can be daunting and full of jargon. It is a field populated by acronyms such as DREAD (i.e., Damage, Reproducibility, Exploitability, Affected User, Discoverability) and STRIDE (i.e., Spoofing, Tampering, Repudiation, Information Disclosure, Denial of Service, Elevation of Privilege), and characterized by debates about the distinction of threat and risk. Confusingly, the words “threat model” and “threat modeling” are applied in many dissimilar and perhaps incompatible ways. However, for the purpose of this chapter, we will deploy a pragmatic definition and conceptualize threat modeling as the use of abstractions to aid in thinking about threats and risks (for a detailed review, see Shostack, 2014). There are also various approaches to threat modeling, ranging from: (a) asset-based threat modeling; and (b) system-based threat modeling; to (c) attacker-based threat modeling. These approaches can be applied in conjunction with the attempt to generate: (a) an illustration of the system that is potentially being attacked (e.g., a smart watch); (b) assumptions about the profiles of potential attackers, including their goals, methods, and motives (e.g., an IPV perpetrator); and (c) a catalog of likely threats that may arise (e.g., information disclosure). Threat modeling therefore echoes the risk assessment process currently deployed in the IPV support sector (Nicholls, Pritchard, Reeves, & Hilterman, 2013; van der Put, Gubbels, & Assink, 2019). Following Shostack’s (2014, p. xxvii) suggested system-based approach, threat modeling involves four steps, each answering a deceptively simple question: What are you (i.e., the tech vendor) building? What can go wrong with it once it's built? What should you do about those things that can go wrong? Did you do a decent job? Although system-based approaches such as this one are implicitly aimed at tech developers and vendors, we believe it is valuable for anyone studying tech abuse – whether from the perspective of social or computer science – to be comfortable with the conceptual framework of threat modeling. The latter allows researchers to reflect, understand, document, and react to the possible shortcomings of digital devices and services (Sabbagh & Kowalski, 2015; Torr, 2005). By becoming fluent in the language of threat modeling, IPV scholars and practitioners can more effectively critique problematic technology designs. In the upcoming section, we walk readers through the building blocks of this framework. While it may seem abstract, we hope to showcase the benefit of its adoption in the IPV tech abuse space. Specifically, in the following passages, we will apply Shostack's (2014, p. xxvii) four questions to examine how a hypothetical smart lock IoT system can be breached, leading to the harm of an IPV victim/survivor. System: What Are You Building? The threat modeling process begins with collecting necessary information about the relevant components of a device, software program or system (Torr, 2005). This decomposition gives stakeholders an overview of all the different segments, data points, and interactions to effectively identify, understand, and model its makeup (Xiong & Lagerström, 2019). Developers begin by creating simple diagrams and tables to provide an overview of the system being threat modeled. These diagrams can clarify different interdependencies and features of systems, which are particularly important for smart, internet-connected devices (Steven, 2010). For tech designers and vendors, these visual representations form a useful way to abstract all system properties and diagnose what an application does (Coles & Tarandach, 2020). Threats: What Can Go Wrong with It Once It's Built? After the exposure of the “anatomy” of a system, tech vendors use the generated diagrams to look at what could go wrong. For example, a brainstorming meeting to determine and enumerate all potential threats could be held. As there are an unlimited number of things which could fail, this second step has the potential to be the most overwhelming. The evaluation of interconnected systems such as IoT technologies creates an additional level of intricacy than the analysis of individual devices and application alone. However, in both cases, tech designers should assess opportunities for abuse across the whole infrastructure (Coles & Tarandach, 2020). Some approaches start by profiling probable attackers, including their resources, motivations, and capacity (Atzeni, Cameroni, Faily, Lyle, & Flechais, 2011; Little & Rogova, 2006). The identification of an attacker's intentions can assist in the forecasting of an attack's sophistication level, which is particularly useful when examining IPV cases. The threat identification process involves a certain reliance on assumptions as to the nature of a likely perpetrator. These assumptions are often limited and stereotypical (Atzeni et al., 2011), which is – considering the lack of diversity among cybersecurity practitioners, as well as the lack of data on tech abuse – problematic (Lopez-Neira, Patel, Parkin, Danezis, & Tanczer, 2019; Poster, 2018). Having a diverse team is vital for threat modeling. Institutional and personal life experience shape perceptions of threats. Thus, technologists who specialize in Windows systems will often skew their threat model toward Windows-specific concerns, while web developers will be primarily focused on web-based attacks. Equally, our own biases as authors of this chapter will have influenced the threat actors and attack scenarios we are examining. To mitigate such shortcomings, we want to reiterate that active collaboration with affected groups and communities such as the domestic abuse sector must be sought. When looking at an attacker's profile, both their opportunities for exploitation and/or their attack motives can be significantly influenced by environmental conditions. For instance, a perpetrator with a background in software development may be far more likely to consider exploiting smart home devices. Nonetheless, an attacker's capacity must be contrasted, considering their potential motivation. Depending on both aspects, one must expect changes to the: (a) intensity; (b) sophistication; and (c) probability of a tech abuse attack taking place; as well as (d) a perpetrator's ability to distort/eliminate forensic evidence (UcedaVelez & Morana, 2015). Based on the current evidence-base, tech abuse perpetrators are often highly motivated or even obsessed with the desire to monitor, coerce, intimidate, or otherwise harm a victim/survivor. They can, but do not have to, be physically present (Ho et al., 2016). Abusers are also rarely strangers. 5 They often have or had romantic relations with victims/survivors. Nonetheless, tech abuse can also be perpetrated by family members, colleagues, roommates, or acquaintances (Levy, 2015). IPV perpetrators often have intimate knowledge of the victim/survivor, including awareness of their daily habits, history, and login details, or access to personal data like sexually explicit or embarrassing photos and messages (Table 39.1). Table 39.1. Tech Abuse Threat Model. Name Description Ownership-based access Being the Owner of a device or account allows a perpetrator to prohibit victims'/survivors' usage or track their location and actions; Account/device compromise Guessing or coercing credentials which enables a perpetrator to install spyware, monitor the victim/survivor, steal their data, or lock them out of their account; Harmful messages Contacting victims/survivors or their friends, family, employers, etc. without their consent; Exposure of information Posting or threatening to post private information or nonconsensual pornography (i.e., image-based sexual abuse); Gaslighting Using a device's functionality (e.g., remote changing of temperature) to make a victim/survivor feel as if they are losing their sanity and/or control over their home. In addition to this profiling exercise, it is helpful to account for known attack patterns (UcedaVelez & Morana, 2015). Drawing on Freed et al. (2017) and Leitão (2019), we propose a model of five common tech abuse threats: These threats can be connected to the specific features of the device in order to identify which forms of tech abuse are possible/likely (as we do in the following section). The second step ends with documenting as well as rating all diagnosed threats (Meier et al., 2003). Response: What Should You Do about Those Things that Can Go Wrong? The third question involves the examination of countermeasures to tackle each threat. Conventionally, responses are (a) to reduce/mitigate threats through the implementation of safeguards and changes to eliminate vulnerabilities or block threats; (b) to assign/transfer threats by placing the cost of the threat onto another entity or organization such as purchasing insurance or outsourcing; or (c) to accept the threat by evaluating if the cost of the countermeasure outweighs the possible cost of loss due to the threat. While the full elimination of threats is generally possible, it would require almost always the removal of features which industry actors may be opposed to (Shostack, 2014). Mitigations are consequently specific to a device's design goals and limited by a vendor's resources, interests, and capacity. Therefore, this step also involves prioritizing different threats in order to identify which mitigations are most urgent. In the private sector, such assessments are often quantified and based on financial losses. Tech vendors have so far struggled – and often failed – to incorporate more intangible social, emotional, or psychological harms, including damage to reputation or mental health implications. The industry's viewpoint on the importance of economic ramifications disproportionally disregards the broader implications technical innovations may have on different groups of society, which we aspire to alleviate in this chapter. Validation: Did You Do a Decent Job of Analysis? The final question involves a critical reflection on the efficacy of the generated threat model. To support this evaluation process, different validation methods can be deployed (Xiong & Lagerström, 2019). What unifies these methods is their attempt to check the model's completeness and accuracy. The scrutiny guarantees that the final model matches the system that is built, addresses all the right and relevant threats, and covers all the decisions that have been made (Shostack, 2014). By this stage, every possible attack scenario should have been considered and accounted for and a planned countermeasure laid out. A common practice to support this step is the reliance on “test cases” or “case studies” (Shostack, 2014; Xiong & Lagerström, 2019). Another form of explanation and validation includes collecting data on device usage “in the wild.” Moreover, data on reported breaches can be helpful, especially if contrasted with initial threat models to understand whether a threat was inadequately addressed or missed entirely. Together with a frequent reiteration of the threat modeling exercise, new and unanticipated threats can be accounted for and timely and effective mitigation strategies implemented. Threat Modeling a Smart, Internet-Connected Lock The followin
更多查看译文
关键词
intimate partner violence,cybersecurity challenge,tech abuse
AI 理解论文
溯源树
样例
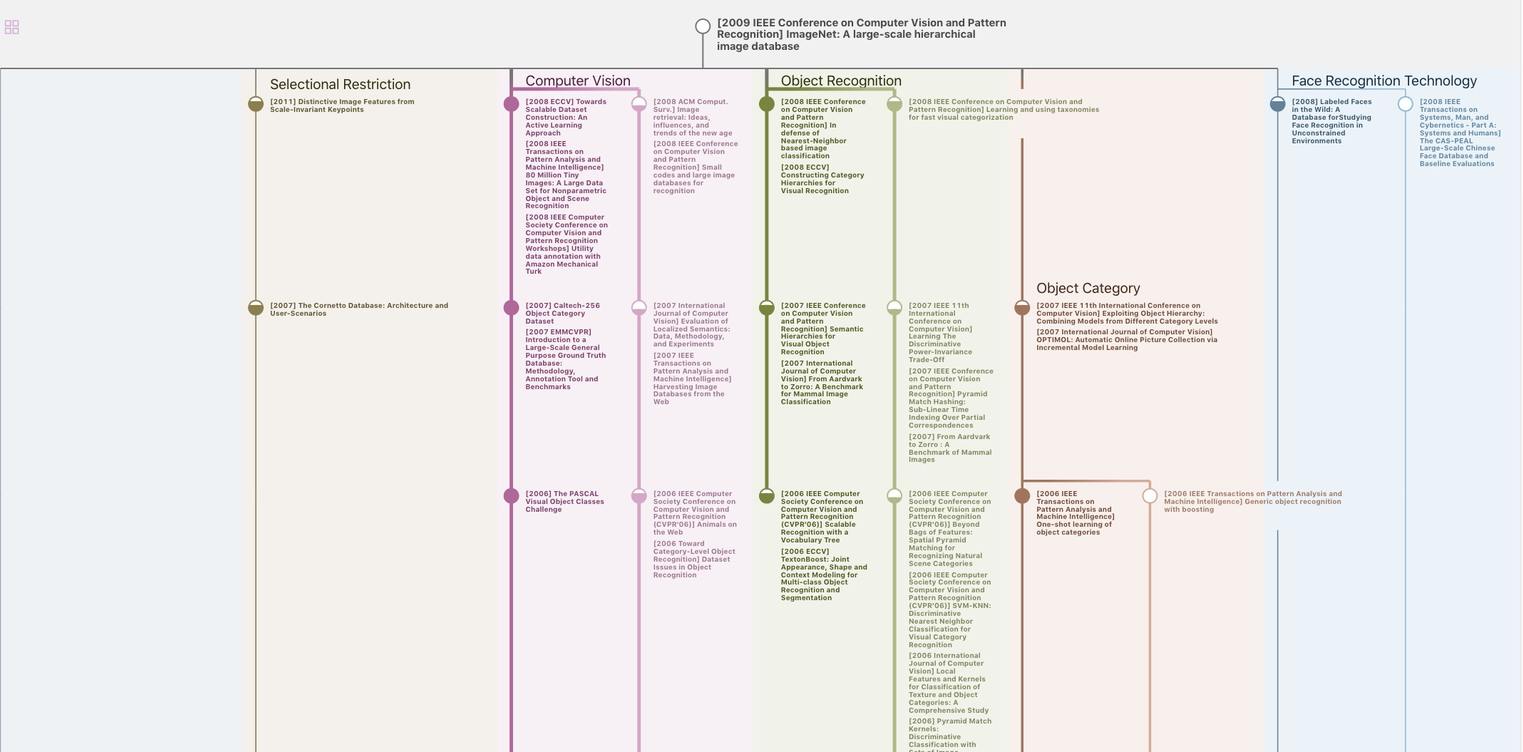
生成溯源树,研究论文发展脉络
Chat Paper
正在生成论文摘要