RESTEP Into the Future: Relational Spatio-Temporal Learning for Multi-Person Action Forecasting
IEEE Transactions on Multimedia(2023)
摘要
Multi-person action forecasting is an emerging topic in the computer vision field, and it is a pivotal step toward video understanding at a semantic level. This task is difficult due to the complexity of spatial and temporal dependencies. Yet, the state-of-the-art literature does not seem to be adequately responsive to this challenge. Hence, how to better foresee the forthcoming actions per actor has to be further pursued. Toward this end, we put forth a novel RElational Spatio-TEmPoral learning approach (RESTEP) for multi-person action forecasting. Our RESTEP explores the key that inherently characterizes actions from a perspective of incorporating the spatial and temporal information in a single pass (spatio-temporal dependencies) by extending relational reasoning. As a result, the RESTEP enables simultaneously predicting the actions of all actors in the scene. Our proposal significantly differs from mainstream works that heavily rely on independently processing the spatial and temporal dependencies. The proposed RESTEP first perceives a graph building upon the historical observations, then reasons the relational spatio-temporal context to extrapolate future actions. In order to augment the comprehension of individual actions that might vary over time, we further delve deeper into the essence behind this point - the evolution of spatio-temporal dependencies via optimizing the corresponding mutual information. We assess the RESTEP method on the large-scale Atomic Visual Actions (AVA) dataset, Activities in Extended Videos (ActEV/VIRAT) dataset and Joint-annotated Human Motion Data Base (J-HMDB). The experimental outcomes reveal that RESTEP can introduce considerable improvements with respect to recent leading studies.
更多查看译文
关键词
Multi-person action forecasting, spatiotemporal dependencies, graph neural network, weakly-supervised learning
AI 理解论文
溯源树
样例
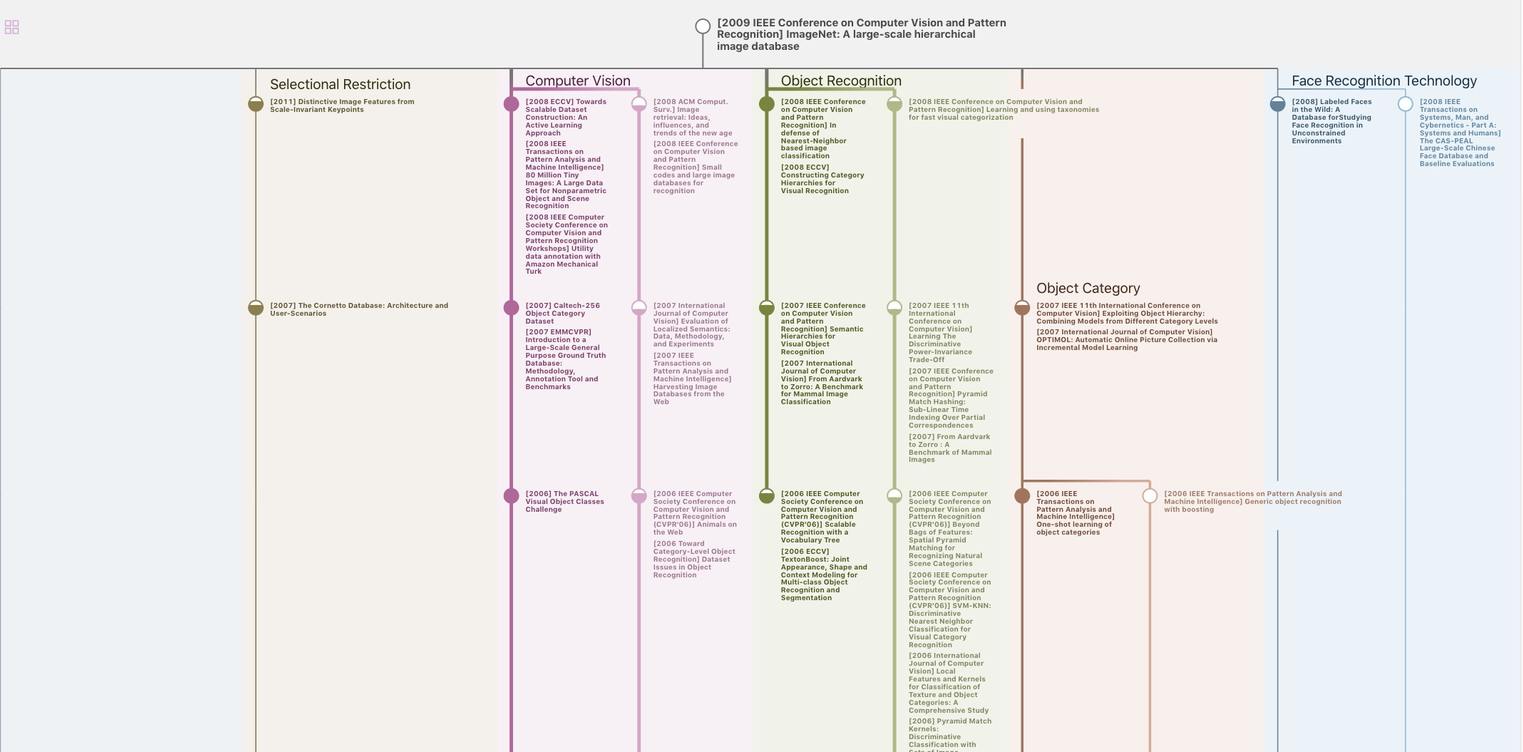
生成溯源树,研究论文发展脉络
Chat Paper
正在生成论文摘要