Producing grain yield maps by merging combine harvester and remote sensing data
Precision agriculture ’21(2021)
摘要
High-quality combine harvester yield data are very important to produce yield maps. However, errors that derive from the combine harvester may be corrected. A data source that could be used to improve the yield maps obtained from harvester data is satellite-based data (e.g. Sentinel 2 (S2) imageries) and unmanned aerial vehicles (UAV) based data that are commonly used to predict grain yield and also define fertilisation rates. In this study, yield data from a combine harvester, a multi-spectral camera mounted on a UAV and S2 images were collected to produce yield maps that offer more accurate representation. To calibrate and validate this method, biomass samples were acquired manually before harvesting while erroneous yield data were replaced by remote sensing data. A regression analysis between the ground-truth biomass samples and the filtered yield data had an R-2 value equal to 0.84, while the R-2 of the biomass with the yield data obtained by the proposed methodology was equal to 0.90.
更多查看译文
关键词
normalised difference vegetation index (NDVI), Sentinel imagery, unmanned aerial vehicle (UAV) data, yield data cleaning
AI 理解论文
溯源树
样例
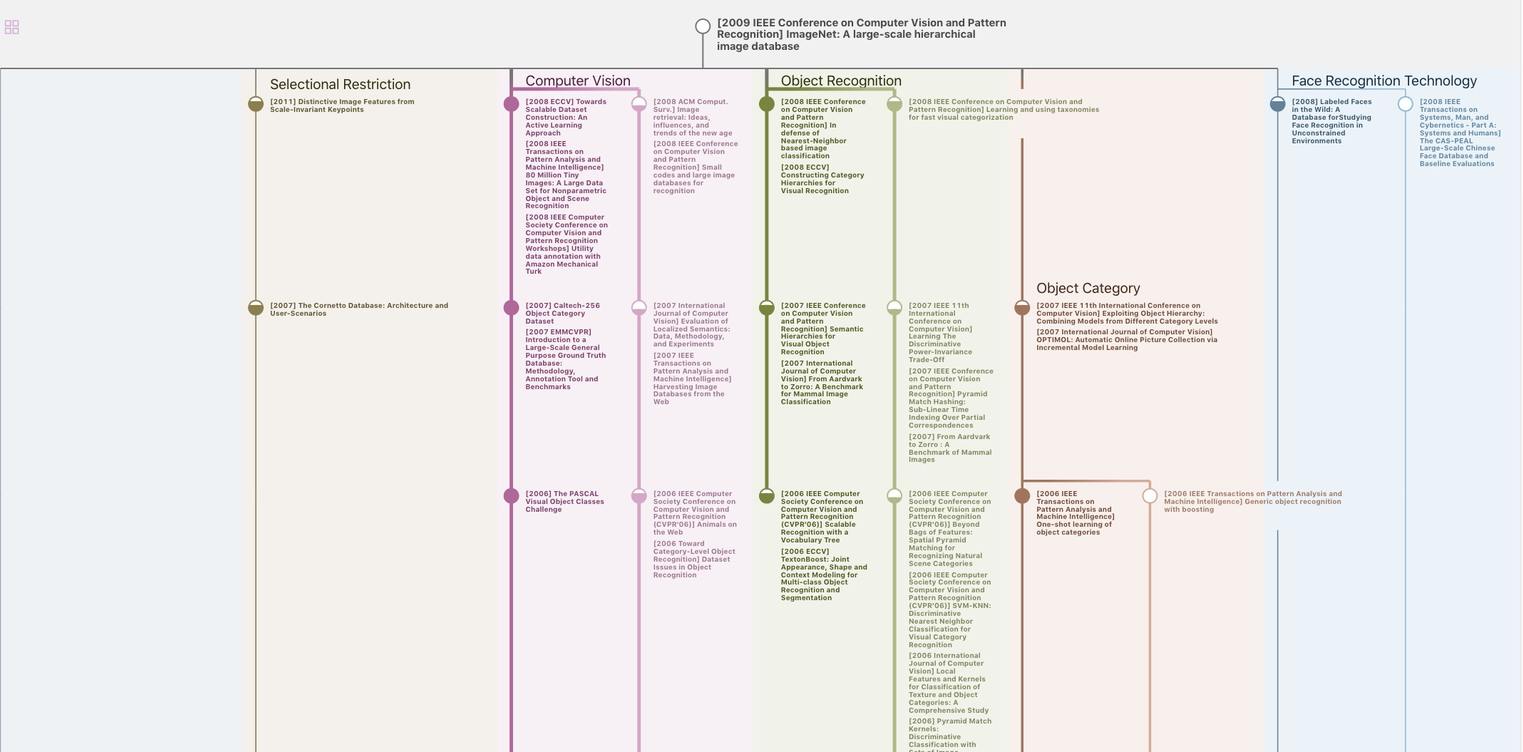
生成溯源树,研究论文发展脉络
Chat Paper
正在生成论文摘要